TECHNOLOGY
The State of Computer Vision in 2023: Opportunities and Challenges Ahead
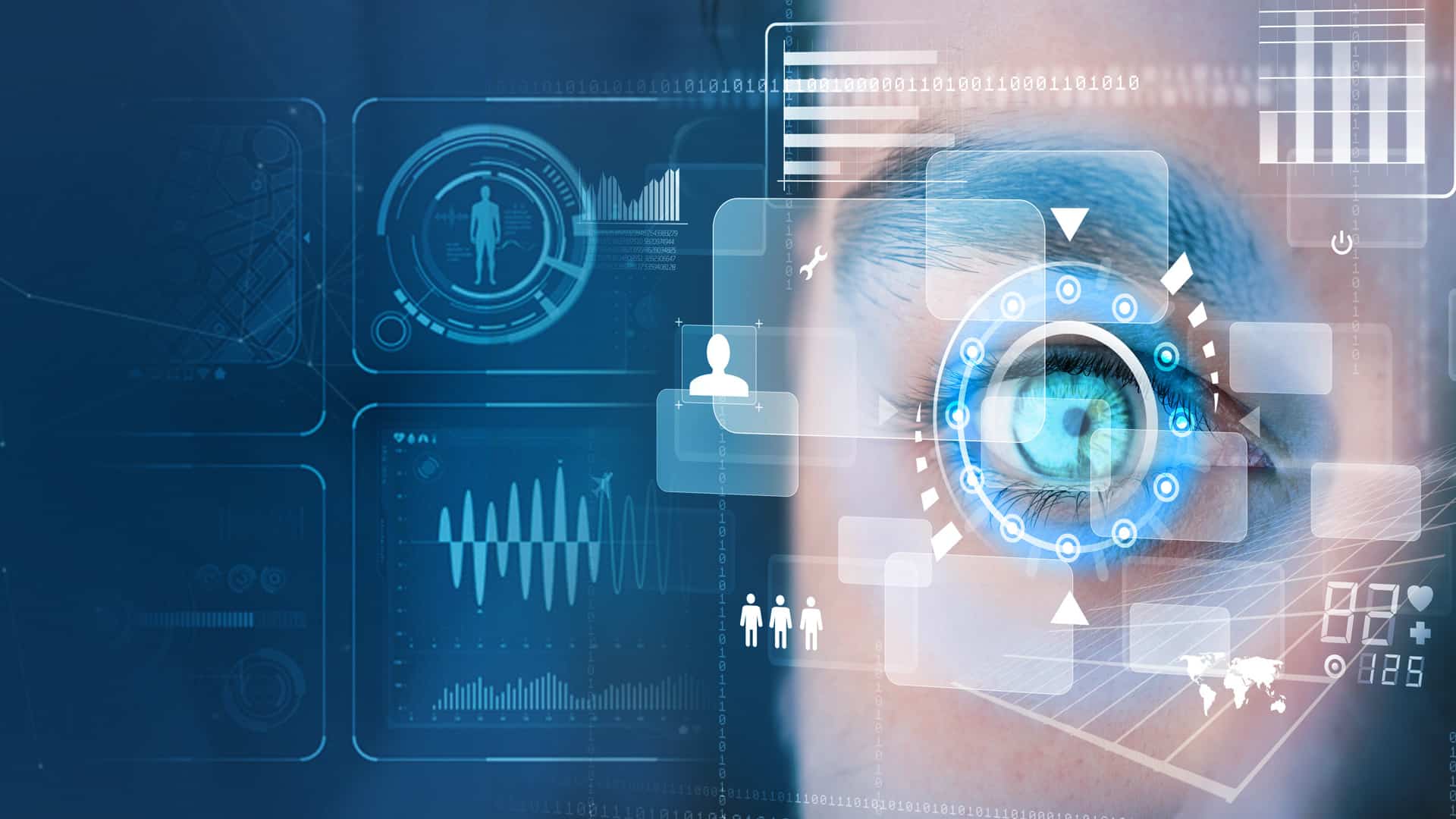
The field of computer vision has come a long way since the first experiments with image recognition in the 1960s.
Computer vision technology is being used in a wide range of applications, from self-driving cars to healthcare to security systems. In 2023, the state of computer vision is strong, with recent advances in deep learning, neural networks, and image processing pushing the boundaries of what’s possible. But there are also significant challenges facing the field, including ethical considerations and the need for more diverse and representative data sets. In this article, we’ll explore the state of computer vision in 2023, the opportunities that lie ahead, and the challenges that must be overcome to unlock its full potential.
Recent Advances in Computer Vision
In recent years, deep learning has emerged as a powerful tool for computer vision. Deep learning algorithms, which use artificial neural networks to mimic the way the human brain processes information, have been used to achieve breakthroughs in image recognition and classification. For example, in 2012, a deep learning algorithm called AlexNet achieved a record-breaking 15.3% error rate in the ImageNet Large Scale Visual Recognition Challenge, beating the previous best result by a wide margin.
Since then, deep learning has continued to improve, with new algorithms and architectures pushing the limits of what’s possible. For example, in 2020, researchers at Google introduced a new deep learning architecture called EfficientNet, which achieved state-of-the-art results on a range of image classification tasks while using fewer parameters than previous models. EfficientNet has since been adopted by a wide range of companies and researchers, highlighting the power of deep learning in computer vision.
Another area of recent progress in computer vision is in image processing. Advances in image processing algorithms have made it possible to extract more information from images, such as detecting and tracking objects in real-time video streams. For example, in 2018, researchers at Stanford University developed a real-time object detection algorithm called YOLO (You Only Look Once), which achieved state-of-the-art performance on a range of benchmarks. YOLO has since been used in a wide range of applications, including self-driving cars and security systems.
Opportunities for Computer Vision
The recent advances in computer vision have opened up a range of new opportunities in a variety of industries. Here are a few examples:
Healthcare: Computer vision can be used in a wide range of healthcare applications, such as diagnosing diseases from medical images, monitoring patients remotely, and improving surgical outcomes. For example, in 2018, researchers at Stanford University developed a deep learning algorithm that can diagnose skin cancer with the same accuracy as human dermatologists.
Retail: Computer vision can be used in retail to improve the shopping experience, such as by automatically detecting and recognizing products, or by tracking customer behavior to make personalized recommendations. For example, Amazon Go stores use computer vision to track customers as they move around the store and automatically charge them for the products they take.
Security: Computer vision can be used in security systems to detect and track intruders, or to recognize individuals based on their facial features. For example, the Chinese government has developed a nationwide surveillance system called Skynet, which uses facial recognition technology to track individuals and monitor their behavior.
Challenges Facing Computer Vision
While the opportunities for computer vision are significant, there are also significant challenges facing the field. Here are a few examples:
Ethics: Computer vision can be used for both good and bad purposes, such as in surveillance systems that infringe on privacy or in facial recognition systems that perpetuate biases. It’s important for researchers and developers to consider the ethical implications of their work and to ensure that their systems are designed to respect individual rights and promote social justice.
Data Bias: Computer vision algorithms are only as good as the data they are trained on. If the data is biased or unrepresentative, the algorithm will learn those biases and perpetuate them in its predictions. This can lead to unfair and discriminatory outcomes, particularly in applications like facial recognition, where biases can disproportionately impact marginalized communities. To overcome this challenge, researchers and developers must ensure that their data sets are diverse, representative, and free from bias.
Adversarial Attacks: Computer vision algorithms can also be vulnerable to adversarial attacks, where an attacker deliberately manipulates an image or video to deceive the algorithm. Adversarial attacks can be used to trick security systems, misclassify objects, or even cause self-driving cars to crash. To address this challenge, researchers are developing new algorithms and techniques that can detect and defend against adversarial attacks.
Hardware Limitations: Computer vision algorithms can be computationally expensive, requiring large amounts of processing power and memory. This can limit their scalability and practicality in real-world applications. To overcome this challenge, researchers are developing more efficient algorithms and hardware architectures, such as specialized chips designed specifically for deep learning.
What is the Future of Computer Vision?
According to Allied Market Research, the computer vision market has been expanding across multiple industries in the past years, leading to an expected growth of $17.4 billion in revenue by 2023 and $41.11 billion by 2030.
The state of computer vision in the future is strong, with recent advances in deep learning, neural networks, and image processing pushing the boundaries of what’s possible. Computer vision is being used in a wide range of applications, from healthcare to retail to security systems, and holds tremendous promise for the future. Nonetheless, there are also significant challenges facing the field, including ethical considerations, data bias, adversarial attacks, and hardware limitations. To unlock the full potential of computer vision, researchers and developers must continue to address these challenges and ensure that their systems are designed to promote fairness, transparency, and social justice.