TECHNOLOGY
The Convergence of Semantics and Machine Learning
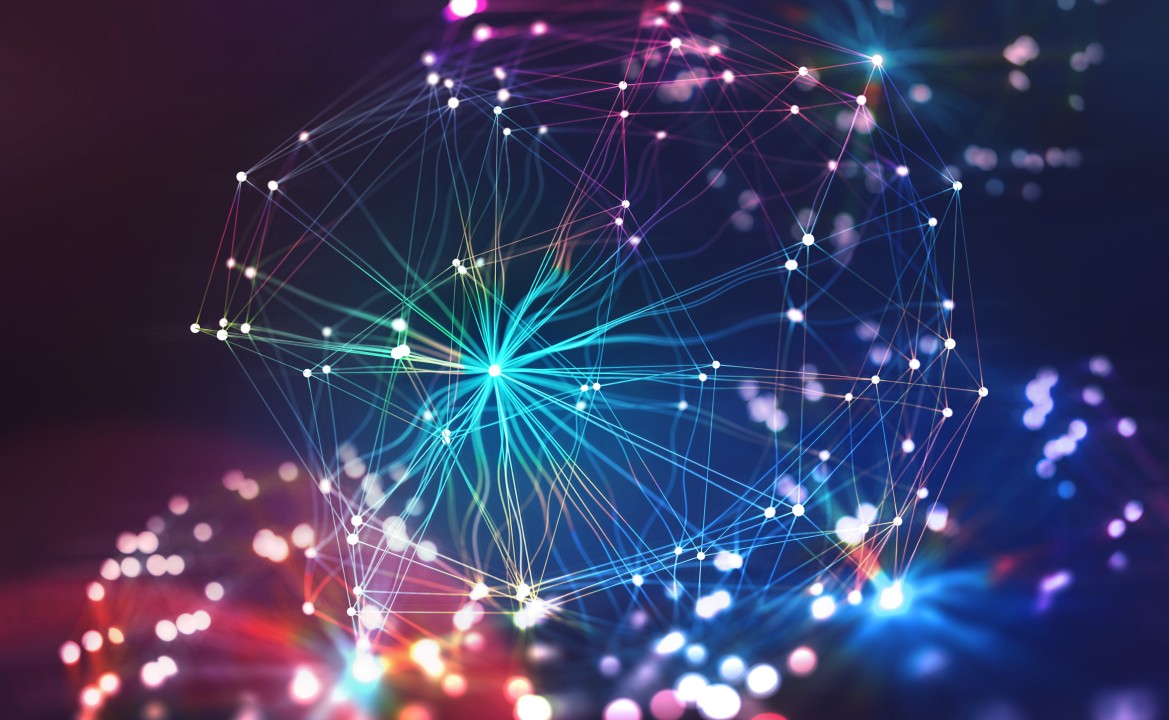
Artificial intelligence (AI) has a long history of oscillating between two somewhat contradictory poles.
On one side, exemplified by Noam Chomsky, Marvin Minsky, Seymour Papert, and many others, is the idea that cognitive intelligence was algorithmic in nature – that there were a set of fundamental precepts that formed the foundation of language, and by extension, intelligence. On the other side were people like Donald Hebb, Frank Rosenblatt, Wesley Clarke, Henry Kelly, Arthur Bryson, Jr., and others, most not even as remotely well known, who developed over time gradient descent, genetic algorithms, back propagation and other pieces of what would become known as neural networks.
The rivalry between the two camps was fierce, and for a while, after Minsky and Papert’s fairly damning analysis of Rosenblatt’s Perceptron, one of the first neural model, it looked like the debate had been largely settled in the direction of the algorithmic approach. In hindsight, the central obstacle that both sides faced (and one that would put artificial intelligence research into a deep winter for more than a decade) was that both underestimated how much computing power would be needed for either one of the models to actually bear fruit, and it would take another fifty years (and an increase of computing factor by twenty-one orders of magnitude, around 1 quadrillion times) before computers and networks reached a point where either of these technologies was feasible.
As it turns out, both sides were actually right in some areas and wrong in others. Neural networks (and machine learning) became very effective in dealing with many problems that had been seen as central in 1964: image recognition, auto-classification, natural language processing, and systems modeling, among other areas. The ability to classify, in particular, was a critical step forward, especially given the deluge of content (from Twitter posts to movies) that benefit from this.
At the same time, however, there are echoes of Minsky and Papert’s arguments about the Perceptron in the current debate about machine learning – discoverability and verifiability are both proving to be remarkably elusive problems to solve. If it is not possible to determine why a given solution is correct, then it means that there are significant hidden variables that aren’t being properly modeled, and not knowing the limits of those variables – the places where you have discontinuities and singularities, make the model far more questionable when applied to anything but its own training data.
Additionally, you replace the problem of human intervention in developing logical (and sometimes social) structures with the often time and people-intensive operation of finding and curating large amounts of data, and it can be argued that the latter operation is in fact just a thinly disguised (and arguable less efficient) version of the former.
The algorithmic side of things, on the other hand, is not necessarily faring that much better. There are in fact two facets to the algorithmic approach – analytical and semantic. The analytical approach, which can be identified as being currently defined as Data Science, involves the use of statistical analysis (or stochastics) to determine distributions and probabilities. Stochastics’ strength arguably comes in that it can be used to determine, for a sufficiently large dataset, the likelihood of specific events occurring can be established to within a certain margin of error. However, stochastics is shifting from traditional statistical analysis to the use of Bayesian networks, in which individual variables (features) can be analyzed through graph analysis.
Semantics, on the other hand, is the utilization of network graphs connecting assertions, as well as the ability to make additional assertions (via modeling) about the assertions themselves, a process known as reification. Semantics lends itself well to more traditional modeling approaches, precisely because traditional (relational) modeling is a closed subset of the semantics model, while at the same time providing the power inherent in document-object-modeling languages (DOMs) such as exemplified by XML or JSON.
Significantly, a Bayesian network can be rendered as a semantic graph with reification, as can a decision tree. Indeed, a SPARQL query is isomorphic to a decision tree in every way that counts, as each node in a decision tree is essentially the intersection of two datasets based upon the presence of specific patterns or constraints (Hint: you want to build a compliance testing system? Use SPARQL!).
The history of software is both full of purists and less full of pragmatists. Purists put a stake in the ground regarding their own particular set of tools and languages: C++ vs. Java, Imperative vs. Declarative, SQL vs NoSQL, Perl vs. … well, just about anything, when you get right down to it. Pragmatists usually try to find a middle ground, picking and choosing the best where they can and covering their ears to all of the sturm and drang of the religious wars when they can’t. Most purists ultimately become pragmatists over the years, but because most programmers tend to become program management over the years, the actual impact of such learning is minimal.
Right now, because the incarnations of all three of these areas – neural networks, Bayesians, and semantics – are relatively new, there is a strong tendency to want to see one’s tool of choice as being the best for all potential situations. However, I’d argue that each of these are ultimately graphs or tools to work with graphs, and it is this underlying commonality that I believe will lead to a broader unification. For instance,
- A machine learning pipeline is a classifier. If the labels of the classifier in the middle correspond to a given ontology, then once a given entity has been classified, a representation of that entity semantically can be assigned to the relevant patterns, shapes, classes, or rules.
- A machine learning system is not an index, but as my kids would say, it is index-adjacent (what a very graph-like phrase). In essence, what you’re doing is creating a map between an instance of an unknown type and its associated class(es). The plural term is important here because a class is not a thing, it is only a labeled pattern, with inheritance, in turn, being the identification of common features between two such patterns. This map is also occasionally referred to as an inverse query, in that rather than retrieving all items that satisfy the query, you are in essence retrieving the (named) patterns that the query utilizes for one of those items.
- It is possible (and relatively simple, to be honest) to create classifiers in SPARQL. This is because SPARQL essentially is looking for the existence of triple patterns, not just in terms of property existence, but in terms of often secondary and tertiary relationships. SHACL, an RDF schematic language, can be thought of as a tool for generating SPARQL based upon specific SHACL constructs (among other things) and those patterns can be very subtle.
- In a similar fashion, I believe that graph analytics will end up becoming as (or even more) important compared to relational data analytics, primarily because graphs make it much easier to add multiple layers of abstraction and discoverability to any kind of stochastic process, resolving many of the same issues that machine learning tools today struggle with.
- The inverse of this process is also feasible. SPARQL can be utilized with incoming streams to create a graph that serves to build training data for machine language services. Because such training data will already have been labeled and identified within the context of existing ontologies, the benefit of such a process is that the resulting classifiers already have all the pieces necessary for explainability – data provenance and annotations, established identifiers, event timestamps, and more.
- One other important point – SPARQL is able to change the graphs that it works with. Inferences, in which new assertions are created based upon patterns found in existing assertions, become especially important once you incorporate service calls that allow for the processing of external content directly within the SPARQL calls themselves. One of the next major evolution points for SPARQL will be in its ability to retrieve, manipulate and produce JSON as intermediate core objects (software vendors, please take note) or as sources for RDF.
- This means that a future version of SPARQL no longer has to store tabular data as RDF, but instead could store it as JSON then utilize that JSON (and associated analytics functions) to create far more sophisticated inferences with a much smaller processing footprint. For an analogous operation, take a look at the XProc XML pipeline processing languages then realize that the differences between the XSLT/XQuery pipelines and the RDF/SPARQL/SHACL pipelines are mostly skin deep.
This last point is very, very important because as the latest iterations of the Agile / DevOPS / MLOps model show, pipelines and transformations are the future. By being able to work with chained transformations (especially ones where the specific pipes within that transformation are determined based upon context rather than set a prior) such pipelines begin to look increasingly like organic cognitive processes.
You must be logged in to post a comment Login