SEO
How It Works & Who It’s For
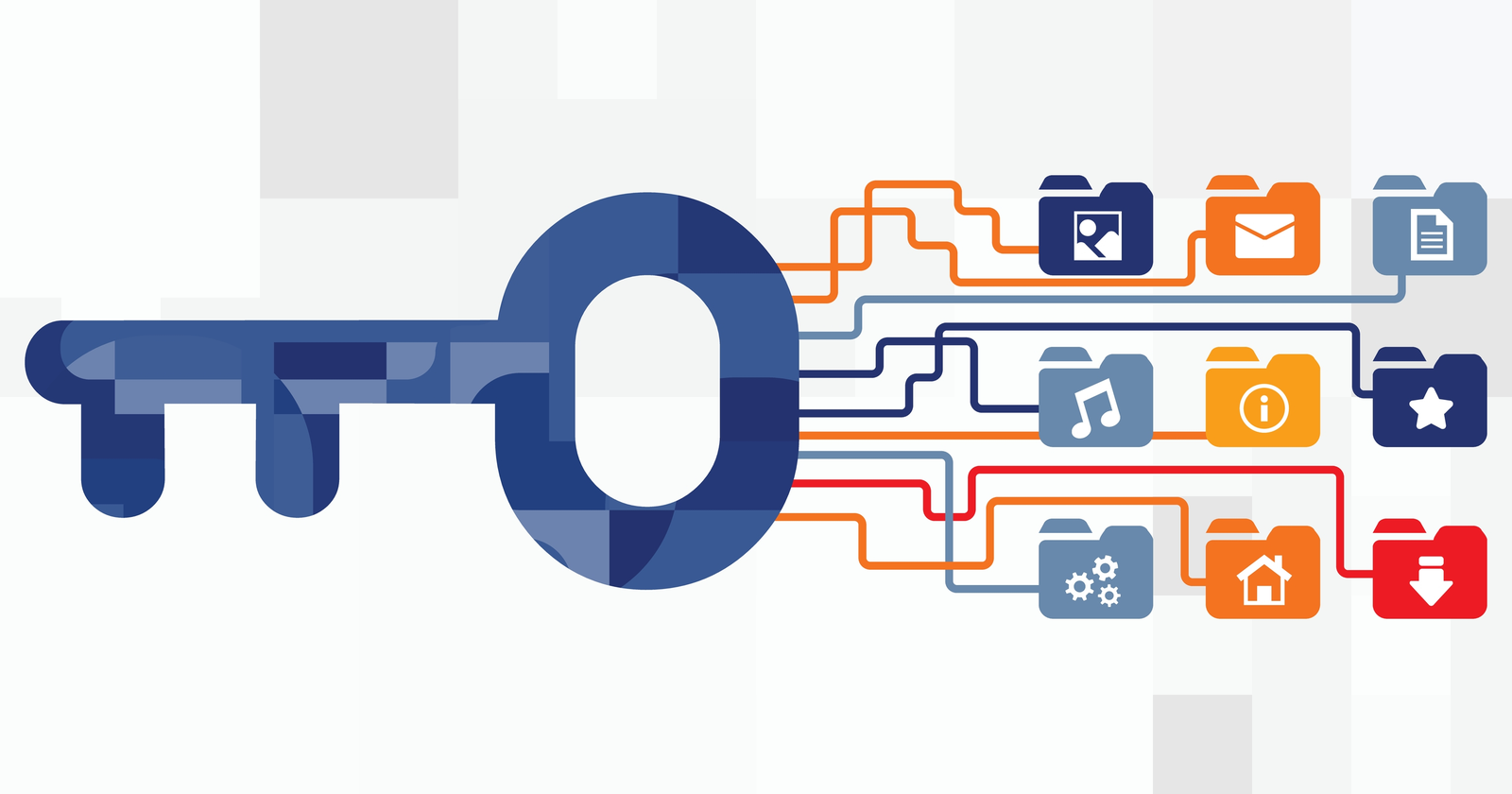
For simple user queries, a search engine can reliably find the correct content using keyword matching alone.
A “red toaster” query pulls up all of the products with “toaster” in the title or description, and red in the color attribute.
Add synonyms like maroon for red, and you can match even more toasters.
But things start to become more difficult quickly: You have to add these synonyms yourself, and your search will also bring up toaster ovens.
This is where semantic search comes in.
Semantic search attempts to apply user intent and the meaning (or semantics) of words and phrases to find the right content.
It goes beyond keyword matching by using information that might not be present immediately in the text (the keywords themselves) but is closely tied to what the searcher wants.
For example, finding a sweater with the query “sweater” or even “sweeter” is no problem for keyword search, while the queries “warm clothing” or “how can I keep my body warm in the winter?” are better served by semantic search.
As you can imagine, attempting to go beyond the surface-level information embedded in the text is a complex endeavor.
It has been attempted by many and incorporates a lot of different components.
Additionally, as with anything that shows great promise, semantic search is a term that is sometimes used for search that doesn’t truly live up to the name.
To understand whether semantic search is applicable to your business and how you can best take advantage, it helps to understand how it works, and the components that comprise semantic search.
What Are The Elements Of Semantic Search?
Semantic search applies user intent, context, and conceptual meanings to match a user query to the corresponding content.
It uses vector search and machine learning to return results that aim to match a user’s query, even when there are no word matches.
These components work together to retrieve and rank results based on meaning.
One of the most fundamental pieces is that of context.
Context
The context in which a search happens is important for understanding what a searcher is trying to find.
Context can be as simple as the locale (an American searching for “football” wants something different compared to a Brit searching the same thing) or much more complex.
An intelligent search engine will use the context on both a personal level and a group level.
The personal level influencing of results is called, appropriately enough, personalization.
Personalization will use that individual searcher’s affinities, previous searches, and previous interactions to return the content that is best suited to the current query.
It is applicable to all kinds of searching, but semantic search can go even further.
On a group level, a search engine can re-rank results using information about how all searchers interact with search results, such as which results are clicked on most often, or even seasonality of when certain results are more popular than others.
Again, this displays how semantic search can bring in intelligence to search, in this case, intelligence via user behavior.
Semantic search can also leverage the context within the text.
We’ve already discussed that synonyms are useful in all kinds of search, and can improve keyword search by expanding the matches for queries to related content.
But we know as well that synonyms are not universal – sometimes two words are equivalent in one context, and not in another.
When someone searches for “football players”, what are the right results?
The answer will be different in Kent, Ohio than in Kent, United Kingdom.
A query like “tampa bay football players”, however, probably doesn’t need to know where the searcher is located.
Adding a blanket synonym that made football and soccer equivalent would have led to a poor experience when that searcher saw the Tampa Bay Rowdies soccer club next to Ron Gronkowski.
(Of course, if we know that the searcher would have preferred to see the Tampa Bay Rowdies, the search engine can take that into account!)
This is an example of query understanding via semantic search.
User Intent
The ultimate goal of any search engine is to help the user be successful in completing a task.
That task might be to read news articles, buy clothing, or find a document.
The search engine needs to figure out what the user wants to do, or what the user intent is.
We can see this when searching on an ecommerce website.
As the user types the query “jordans”, the search automatically filters on the category, “Shoes.”
This anticipates that the user intent is to find shoes, and not jordan almonds (which would be in the “Food & Snacks” category).
By getting ahead of the user intent, the search engine can return the most relevant results, and not distract the user with items that match textually, but not relevantly.
This can be all the more relevant when applying a sort on top of the search, like price from lowest to highest.
This is an example of query categorization.
Categorizing the query and limiting the results set will ensure that only relevant results appear.
Difference Between Keyword And Semantic Search
We have already seen ways in which semantic search is intelligent, but it’s worth looking more at how it is different from keyword search.
While keyword search engines also bring in natural language processing to improve this word-to-word matching – through methods such as using synonyms, removing stop words, ignoring plurals – that processing still relies on matching words to words.
But semantic search can return results where there is no matching text, but anyone with knowledge of the domain can see that there are plainly good matches.
This ties into the big difference between keyword search and semantic search, which is how matching between query and records occurs.
To simplify things some, keyword search occurs by matching on text.
“Soap” will always match “soap” or “soapy ”, because of the overlap in textual quality.
More specifically, there are enough matching letters (or characters) to tell the engine that a user searching for one will want the other.
That same matching will also tell the engine that the query soap is a more likely match for the word “soup” than the word “detergent.”
That is unless the owner of the search engine has told the engine ahead of time that soap and detergent are equivalents, in which case the search engine will “pretend” that detergent is actually soap when it is determining similarity.
Keyword-based search engines can also use tools like synonyms, alternatives, or query word removal – all types of query expansion and relaxation – to help with this information retrieval task.
NLP and NLU tools like typo tolerance, tokenization, and normalization also work to improve retrieval.
While these all help to provide improved results, they can fall short with more intelligent matching, and matching on concepts.
Semantic Search Matches On Concepts
Because semantic search is matching on concepts, the search engine can no longer determine whether records are relevant based on how many characters two words share.
Again, think about “soap” versus “soup” versus “detergent.”
Or more complex queries, like “laundry cleaner”, “remove stains clothing”, or “how do I get grass stains out of denim?”
You can even include things like image searching!
A real-world analogy of this would be a customer asking an employee where a “toilet unclogged” is located.
An employee with only a pure keyword-esque understanding of the request would fail it unless the store explicitly refers to their plungers, drain cleaners, and toilet augers as “toilet uncloggers.”
But, we would hope, the employee is wise enough to make the connection between the various terms and direct the customer to the right aisle.
(Perhaps the employee knows the different terms, or synonyms, a customer can use for any given product).
A succinct way of summarizing what semantic search does is to say that semantic search brings increased intelligence to match on concepts more than words, through the use of vector search.
With this intelligence, semantic search can perform in a more human-like manner, like a searcher finding dresses and suits when searching fancy, with not a jean in sight.
What Is Semantic Search Not?
By now, semantic search should be clear as a powerful method for improving search quality.
As such, you should not be surprised to learn that the meaning of semantic search has been applied more and more broadly.
Often, these search experiences don’t always warrant the name.
And while there is no official definition of semantic search, we can say that it is search that goes beyond traditional keyword-based search.
It does this by incorporating real-world knowledge to derive user intent based on the meaning of queries and content.
This leads to the conclusion that semantic search is not simply about applying NLP and adding synonyms to an index.
It’s true, tokenization does require some real-world knowledge about language construction, and synonyms apply understanding of conceptual matches.
However, they lack, in most cases, an artificial intelligence that is required for search to rise to the level of semantic.
Powered By Vector Search
It is this last bit that makes semantic search both powerful and difficult.
Generally, with the term semantic search, there is an implicit understanding that there is some level of machine learning involved.
Almost as often, this also involves vector search.
Vector search works by encoding details about an item into vectors and then comparing vectors to determine which are most similar.
Again, even a simple example can help.
Take two phrases: “Toyota Prius” and “steak.”
And now let’s compare those to “hybrid.”
Which of the first two are more similar?
Neither would match textually, but you probably would say that “Toyota Prius” is the more similar of the two.
You can say this because you know that a “Prius” is a type of hybrid vehicle because you have seen “Toyota Prius” in a similar context as the word hybrid, such as “Toyota Prius is a hybrid worth considering,” or “hybrid vehicles like the Toyota Prius.”
You’re pretty sure, however, you’ve never seen “steak” and ”hybrid” in such close quarters.
Plotting Vectors To Find Similarity
This is generally how vector search works as well.
A machine learning model takes thousands or millions of examples from the web, books, or other sources and uses this information to then make predictions.
Of course, it is not feasible for the model to go through comparisons one-by-one ( “Are Toyota Prius and hybrid seen together often? How about hybrid and steak?”) and so what happens instead is that the models will encode patterns that it notices about the different phrases.
It’s similar to how you might look at a phrase and say, “this one is positive” or “that one includes a color.”
Except in machine learning the language model doesn’t work so transparently (which is also why language models can be difficult to debug).
These encodings are stored in a vector or a long list of numeric values.
Then, vector search uses math to calculate how similar different vectors are.
Another way to think about the similarity measurements that vector search does is to imagine the vectors plotted out.
This is mind-blowingly difficult if you try to think of a vector plotted into hundreds of dimensions.
If you instead imagine a vector plotted into three dimensions, the principle is the same.
These vectors form a line when plotted, and the question is: which of these lines are closest to each other?
The lines for “steak” and “beef” will be closer than the lines for “steak” and “car” , and so are more similar.
This principle is called a vector, or cosine, similarity.
Vector similarity has a lot of applications.
It can make recommendations based on the previously purchased products, find the most similar image, and can determine which items best match semantically when compared to a user’s query.
Conclusion
Semantic search is a powerful tool for search applications that have come to the forefront with the rise of powerful deep learning models and the hardware to support them.
While we’ve touched on a number of different common applications here, there are even more that use vector search and AI.
Even image search or extracting metadata from images can fall under semantic search.
We’re in exciting times!
And, yet, its application is still early and its known powerfulness can lend itself to a misappropriation of the term.
There are many components in a semantic search pipeline, and getting each one correct is important.
When done correctly, semantic search will use real-world knowledge, especially through machine learning and vector similarity, to match a user query to the corresponding content.
More resources:
Featured Image: magic pictures/Shutterstock
You must be logged in to post a comment Login