MARKETING
Here’s Optimizely’s Automatic Sample Ratio Mismatch Detection
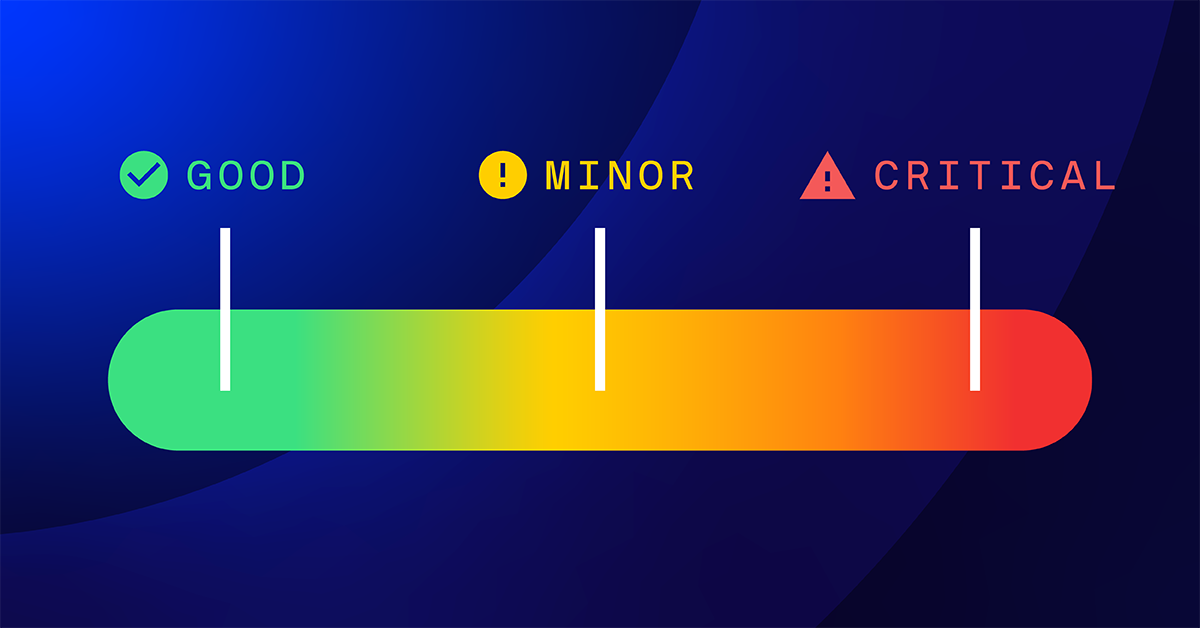
Optimizely Experiment’s automatic sample ratio mismatch (SRM) detection delivers peace of mind to experimenters. It reduces a user’s exposure time to bad experiences by rapidly detecting any experiment deterioration.
This deterioration is caused by unexpected imbalances of visitors to a variation in an experiment. Most importantly, this auto SRM detection empowers product managers, marketers, engineers, and experimentation teams to confidently launch more experiments.
How Optimizely Experiment’s stats engine and automatic sample rate mismatch detection work together
The sample ratio mismatch actslike the bouncer at the door who has a mechanical counter, checking guests’ tickets (users) and telling them which room they get to party in.
Stats engine is like the party host who is always checking the vibes (behavior) of the guests as people come into the room.
If SRM does its job right, then stats engine can confidently tell which party room is better and direct more traffic to the winning variation (the better party) sooner.
Why would I want Optimizely Experiment’s SRM detection?
It’s equally important to ensure Optimizely Experiment users know their experiment results are trustworthy and have the tools to understand what an imbalance can mean for their results and how to prevent it.
Uniquely, Optimizely Experiment goes further by combining the power of automatic visitor imbalance detection with an insightful experiment health indicator. This experiment health indicator plays double duty by letting our customers know when all is well and there is no imbalance present.
Then, when just-in-time insight is needed to protect your business decisions, Optimizely also delivers just-in-time alerts that help our customers recognize the severity of, diagnose, and recover from errors.
Why should I care about sample ratio mismatch (SRM)?
Just like a fever is a symptom of many illnesses, a SRM is a symptom of a variety of data quality issues. Ignoring a SRM without knowing the root cause may result in a bad feature appearing to be good and being shipped out to users, or vice versa. Finding an experiment with an unknown source of traffic imbalance lets you turn it off quickly and reduce the blast radius.
Then what is the connection between a “mismatch” and “sample ratio”?
When we get ready to launch an experiment, we assign a traffic split of users for Optimizely Experiment to distribute to each variation. We expect the assigned traffic split to reasonably match up with the actual traffic split in a live experiment. An experiment is exposed to an SRM imbalance when there is a statistically significant difference between the expected and the actual assigned traffic splits of visitors to an experiment’s variations.
1. A mismatch doesn’t mean an imperfect match
Remember: A bonified imbalance requires a statistically significant result of the difference in visitors. Don’t expect a picture-perfect, identical, exact match of the launch-day traffic split to your in-production traffic split. There will always be some ever-so-slight deviation.
Not every traffic disparity automatically signifies that an experiment is useless. Because Optimizely deeply values our customers’ time and energy, we developed a new statistical test that continuously monitors experiment results and detects harmful SRMs as early as possible. All while still controlling for crying wolf over false positives (AKA when we conclude there is a surprising difference between a test variation and the baseline when there is no real difference).
2. Going under the hood of Optimizely Experiment’s SRM detection algorithm
Optimizely Experiment’s automatic SRM detection feature employs a sequential Bayesian multinomial test (say that 5 times fast!), named sequential sample ratio mismatch. Optimizely statisticians Michael Lindon and Alen Malek pioneered this method, and it is a new contribution to the field of Sequential Statistics. Optimizely Experiment’s sample ratio mismatch detection harmonizes sequential and Bayesian methodologies by continuously checking traffic counts and testing for any significant imbalance in a variation’s visitor counts. The algorithm’s construction is Bayesian inspired to account for an experiment’s optional stopping and continuation while delivering sequential guarantees of Type-I error probabilities.
3. Beware of chi-eap alternatives!
The most popular freely available SRM calculators employ the chi-square test. We highly recommend a careful review of the mechanics of chi-square testing. The main issue with the chi-squared method is that problems are discovered only after collecting all the data. This is arguably far too late and goes against why most clients want SRM detention in the first place. In our blog post “A better way to test for sample ratio mismatches (or why I don’t use a chi-squared test)”, we go deeper into chi-square mechanics and how what we built accounts for the gaps left behind by the alternatives.
Common causes of an SRM
1. Redirects & Delays
A SRM usually results from some visitors closing out and leaving the page before the redirect finishes executing. Because we only send the decision events once they arrive on the page and Optimizely Experiment loads, we can’t count these visitors in our results page unless they return at some point and send an event to Optimizely Experiment.
A SRM can emerge in the case of anything that would cause Optimizely Experiment’s event calls to delay or not fire, such as variation code changes. It also occurs when redirect experiments shuttle visitors to a different domain. This occurrence is exacerbated by slow connection times.
2. Force-bucketing
If a user first gets bucketed in the experiment and then that decision is used to force-bucket them in a subsequent experiment, then the results of that subsequent experiment will become imbalanced.
Here’s an example:
Variation A provides a wildly different user experience than Variation B.
Visitors bucketed into Variation A have a great experience, and many of them continue to log in and land into the subsequent experiment where they’re force-bucketed into Variation A.
But, visitors who were bucketed into Variation B aren’t having a good experience. Only a few users log in and land into a subsequent experiment where they will be force-bucketed into Variation B.
Well, now you have many more visitors in Variation A than in Variation B.
3. Site has its own redirects
Some sites have their own redirects (for example, 301s) that, combined with our redirects, can result in a visitor landing on a page without the snippet. This causes pending decision events to get locked in localStorage and Optimizely Experiment never receives or counts them.
4. Hold/send events API calls are housed outside of the snippet
Some users include hold/send events in project JS. However, others include it in other scripts on the page, such as in vendor bundles or analytics tracking scripts. This represents another script that must be properly loaded for the decisions to fire appropriately. Implementation or loading rates may differ across variations, particularly in the case of redirects.
Interested?
If you’re already an Optimizely Experiment customer and you’d like to learn more about how automatic SRM detection benefits your A/B tests, check out our knowledge base documentation:
For further details you can always reach out to your customer success manager but do take a moment to review our documentation first!
If you’re not a customer, get started with us here!
And if you’d like to dig deeper into the engine that powers Optimizely experimentation, you can check out our page faster decisions you can trust for digital experimentation.