MARKETING
How to start using AI in product development
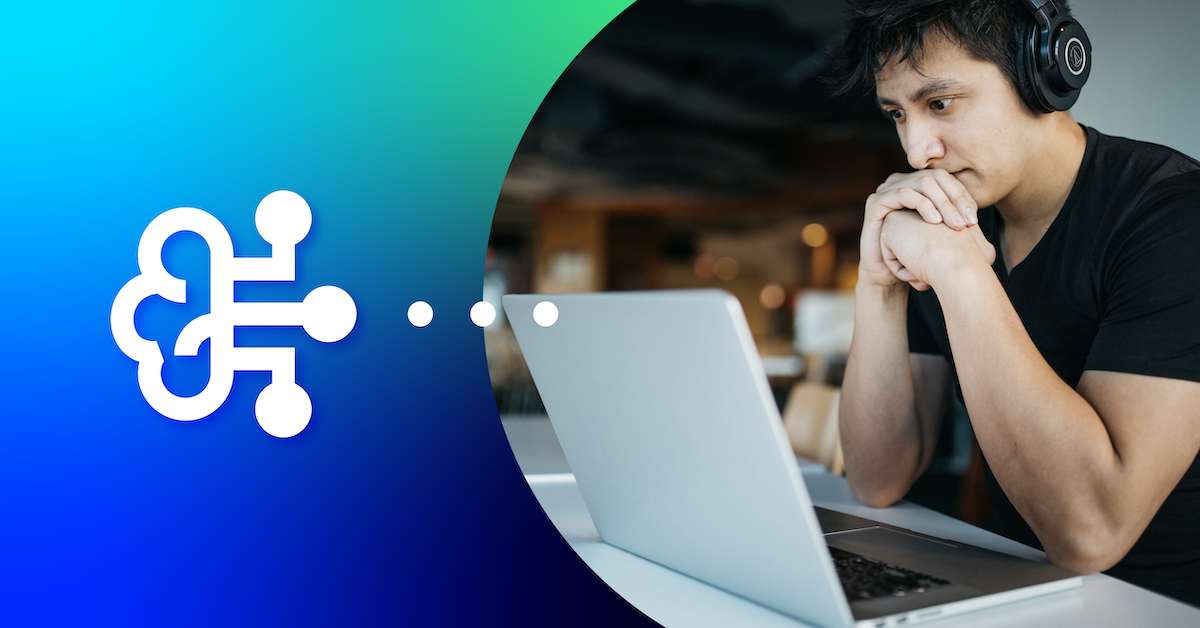
When you go out there to build a product, you want people to use it. As per userpilot, only 17% of users actually use the SaaS products they’re given. Fewer people using your product means they’ll miss out on seeing the value of your product and are unlikely to renew their subscription.
So, building a great product and finding the market fit is essential in any product development lifecycle. Still, understanding potential users, getting deeper insights from customer data, and building prototypes — all take more time than the market is willing to offer you.
Products often go out without much brainstorming or just go out too late. As per a report by Undo, debugging software failures costs roughly $61 billion annually, indicating inadequate testing. However, I sense that the process is going to become more data-driven and easier with the continuous advancements in AI.
And here’s how.
AI is not the solution itself
Yes, AI cannot do everything for you.
Gif source: Giphy.com
AI tools are there to help you build better features and products faster, but they won’t do an end-to-end job for you. As a product team, you should evaluate your product development process for AI readiness. It means assessing the existing infrastructure, availability of quality data, and the technical capabilities of your team.
Involving AI doesn’t make the process much different from a standard one. Following your standard product development practices and understanding user requirements is still paramount. It can reveal opportunities for intelligent automation or personalized experiences.
For example, AI could help you craft an outline for a Product Requirements Document (PRD) based on the user needs you input.
Image source: Chat GPT
From there, it’ll be your responsibility to collect data, think of details, and create the final outline. AI could help you with a lot of steps. You can:
- Automate repetitive tasks like basic product design, generative design, product plans, PDP outlines, etc.
- Use a recommendation engine to personalize the user experience. Cake designs based on preferences.
- Manage inventory, resource allocation and pricing based on data.
- And a lot more
Products ideas should be based on the interests of your users. As consumers continue to change their behavior and interests, AI will adjust the market research in real-time ensuring you’re not wasting dollars on delivering an irrelevant experience to your customers.
For example, the eCommerce industry is using AI to set perfect delivery conditions and solve a $470 billion problem of assuring customers of accurate delivery timelines at checkout. AI even predicts delivery issues that could arise from external factors like bad weather, etc.
In the future, this would mean more than simply adding AI to a product. It would mean a different iteration of the product for every user or set of users. Human creativity will be used to create multiple products from one iteration. It sounds crazy and it is. It also creates a learning challenge. A lot of businesses are confused about investing in the current state of AI. They don’t know whether to wait or what aspects of AI to invest in right now.
Getting started with AI and product development
When thinking of building products, it’s easy to come up with a lot of ideas. But something that a lot of people don’t think about before idea generation is the difference between strategic hypothesis and functional hypothesis. This is exactly where AI can help you.
Using AI to augment customer research
I know we all need to spend more time getting to know our customers. It’s not enough to say I want to sell to a younger audience, watch a few TikToks, and start building a product. Customer feedback is not just about one individual. Every potential customer can have different preferences.
So, what you can do is use AI for data analysis and break down your research into a functional hypothesis. Here data will help your product development team learn:
- What kind of products you can build for your target audience
- Which messaging and content will resonate with this audience even if you’re using chatbots
- Test optimum price point will be relevant to your target audience
This way, every step of product development will have a wider context to the bigger question you’re trying to answer.
And I understand, it’s easier said than done. Each new product development process requires set of product features and modules, and connecting them is hard. Getting approvals from stakeholders is hard as everyone has different opinions. But all this and more is where context-based insights can help you. When it comes to your users, what will provide value regardless of whether you use AI or not, is including customer information and feedback.
Bayes Esports is an excellent example of a company leveraging AI in product development to develop real-world solutions for esports audiences.
Image source: Bayes Esports
Remember, the products you want to build will only succeed if they solve a real problem and data can uncover the actual issues.
A team from the University of Hawaii was working on a project to save the endangered seabird. It used AI to analyze 600 hours of audio to detect how many times the birds would collide with the power lines.
Here the analyzed data was used to create an action plan to reduce the collisions and save the birds.
Image source: Nature dot com
The right use cases for AI
AI cannot do everything for you. You can’t just think of a feature, and expect it to be created directly. Maybe that’d be possible in the future. For now, product development still needs your creativity, direction, data, and hypothesis. Depending on your business, figure out which areas could get the highest value from the use of AI.
For example, you have a manufacturing business. AI can accurately predict future demand based on historical data, seasonality, and market trends at a scale. It’ll help you optimize inventory, production schedules, and more. Let’s say you run an online clothing business. But the return rates are at an all-time high, causing losses. You can use AI to recommend size options to customers or identify customers who return more than usual, creating a different delivery experience for them.
Another Example: Requirement gathering is one of the first stages in the product development lifecycle. It requires significant interaction between project members. However, the process is manual and time-consuming, often missing out on key details. IBM developed Watson AI to effectively assist each step of the process.
Image source: IBM Watson
Knowing if your team is ready for AI
You might have a lot of questions running in your mind:
- How much can I trust AI sources?
- How much of it do I need for ideation?
- How much is good or bad for product management?
There are risks of both investing and not investing in AI technology. To start, there are certain privacy and data leakage concerns. Let’s talk about that first. Data and insights are often subjective interpretations and carry some form of cognitive bias. So. when you’re looking at research to build new products, you’ll have other assumptions in your head. Your product story and why you’re building it the way you are will also be driven by your interpretation of the data. That is why no matter if you use AI or analytic tools for data, remember to test. Experimentation is the only way to validate your approach.
When it comes to content or workflows, generative AI is prone to providing plagiarized or biased information. The hypothesis can feel right to one person and wrong to the other. Be aware of the AI ethical issues and set a process in your organization to tackle them.
Here’s a guide to get you started: AI ethical considerations
Benefits of AI-driven product development
1. Create products that the audience will want to buy
One of the most frustrating things is hearing product teams go ahead and build products thinking they would be easily adopted. It’s very easy to be compelled by research ideas, but it’s when you test ideas, you find out what works and what doesn’t. You simply can’t guess what feature or product will work.
For example, ever since touchscreens came into fashion, even the physical buttons on dashboards have been replaced by touch buttons. In reality, this has proven to be a dangerous proposition for drivers struggling to find key functions without having to look away while driving their cars. So much so that companies around the world are bringing physical buttons back for basic functions.
As per PC Mag, Hyundai even vowed to keep physical buttons in the future.
Image source: PC Mag
When you create a prototype of your product, Artificial Intelligence can capture how users are using it. This data will be super helpful in deciding the best possible direction for your business. Understanding real customer needs that are hiding behind known problems is how AI systems can help you create products that the audience will want to buy.
For example, when foodies wanted to experience food from a different city, Zomato a food delivery company in India, started Intercity Legends. The idea was simple. Utilize the airports and existing delivery infrastructure to deliver a customer’s favorite food from another city. What more? Zomato used AI and data to identify peoples’ favorite foods and combined them with stories. Like butter chicken was connected to its invention story in the streets of Old Delhi by a man who moved to India post-partition.
Image source: Zomato
2. Days of prototyping, done in hours
Creating prototypes, first pagers, and more is the most time-consuming part of a product development lifecycle. It typically takes a week to create the first version, test it, and then do the following versions.
Imagine building prototypes without having to spend weeks. AI can help you do this in hours or minutes even. When you’re building a product, some features are just necessities and don’t drive ROI. You just want to be there and don’t need the most glamorous version. With AI, you can build it quickly and ship it after testing.
Because AI can learn from previous models you built, you can replicate this process on a larger scale.
Ruben Hassid keeps creating images using AI tools and regularly shares his work on LinkedIn.
Image source: LinkedIn
3. Reclaim your time for strategic planning and for yourself
AI in the form of machine learning has been here for years. The old algorithms could turn inputs into outputs, learn from the pattern, and apply it to unseen data. However, the new models are not just learning patterns but also trying to learn the thinking behind any process.
It could be learning how to build a particular feature from scratch. This may seem scary, but you can use it to your advantage. You can be more creative as you don’t have to build different versions of the same product for different users. AI can do that for you. What you can do is spend time creating more powerful products.
You can do anything with the extra time. Watch TV, take a nap, or do nothing 🙂
Why tying AI and experimentation is essential
During a product development lifecycle, product teams lay out the why, what, and when part of building a product. So, if you’re working in a product team, your job will come into play for making decisions not building AI models itself. For example, based on the data, choosing what qualifies to move into production.
That is why you need to start experimenting. A test-and-learn approach eliminates the spray-and-pray method, so customer impact can be quantified at every step. After all, AI or not, customer impact is what drives business goals.
Leadership doesn’t care whether your engagement metrics are working fine, but they do care about how to innovate products, generate more profit, and leave the competition behind. Think of AI and experimentation as friends who can tinker with your products or features, but it’s you who’ll drive the vision.
You can deliver value from every experiment, which will guide your products toward the right path. If you already have an experimentation program running, here’s what will help you:
- Have clear guidance on when to experiment or not
- When you learn something, remember to de-risk changes
- Have uniform experimentation templates and reporting
- Run a culture of experimentation model with office hours
- Deliver Consolidated reporting to the business including dashboards
- Conduct peer review of experiments
And if you want to learn more about improving product delivery through experimentation, check out this article.
Start experimenting with AI
You’ll start moving faster in your product development cycle and deliver products that the audience will love. I highlighted a few examples above – customer research being key – but explore other use cases as they come up.
For now, I’ll leave you with a few key takeaways:
- You do the strategic thinking and let AI-powered functionalities do the dirty manual work for you.
- You can’t rationalize customer behavior. Testing is the way to know what they really want and implement the right strategy.
- How you think customers will use your product and how they actually do often don’t match. AI can help you bridge this gap.
- A product succeeds when it solves a problem experienced by a mass, and context-based insights are what can uncover and validate that problem.
Going forward, I see AI & experimentation aligning perfectly in terms of spirit and decision-making. Remember to test and learn as ultimately anything you can do to understand your user will help you build better products. And hopefully, you’ll either get a promotion, or time back along the way. There’s the dream 🙂
MARKETING
YouTube Ad Specs, Sizes, and Examples [2024 Update]
![YouTube Ad Specs, Sizes, and Examples [2024 Update] YouTube Ad Specs, Sizes, and Examples](https://articles.entireweb.com/wp-content/uploads/2024/06/YouTube-Ad-Specs-Sizes-and-Examples.jpg)
Introduction
With billions of users each month, YouTube is the world’s second largest search engine and top website for video content. This makes it a great place for advertising. To succeed, advertisers need to follow the correct YouTube ad specifications. These rules help your ad reach more viewers, increasing the chance of gaining new customers and boosting brand awareness.
Types of YouTube Ads
Video Ads
- Description: These play before, during, or after a YouTube video on computers or mobile devices.
- Types:
- In-stream ads: Can be skippable or non-skippable.
- Bumper ads: Non-skippable, short ads that play before, during, or after a video.
Display Ads
- Description: These appear in different spots on YouTube and usually use text or static images.
- Note: YouTube does not support display image ads directly on its app, but these can be targeted to YouTube.com through Google Display Network (GDN).
Companion Banners
- Description: Appears to the right of the YouTube player on desktop.
- Requirement: Must be purchased alongside In-stream ads, Bumper ads, or In-feed ads.
In-feed Ads
- Description: Resemble videos with images, headlines, and text. They link to a public or unlisted YouTube video.
Outstream Ads
- Description: Mobile-only video ads that play outside of YouTube, on websites and apps within the Google video partner network.
Masthead Ads
- Description: Premium, high-visibility banner ads displayed at the top of the YouTube homepage for both desktop and mobile users.
YouTube Ad Specs by Type
Skippable In-stream Video Ads
- Placement: Before, during, or after a YouTube video.
- Resolution:
- Horizontal: 1920 x 1080px
- Vertical: 1080 x 1920px
- Square: 1080 x 1080px
- Aspect Ratio:
- Horizontal: 16:9
- Vertical: 9:16
- Square: 1:1
- Length:
- Awareness: 15-20 seconds
- Consideration: 2-3 minutes
- Action: 15-20 seconds
Non-skippable In-stream Video Ads
- Description: Must be watched completely before the main video.
- Length: 15 seconds (or 20 seconds in certain markets).
- Resolution:
- Horizontal: 1920 x 1080px
- Vertical: 1080 x 1920px
- Square: 1080 x 1080px
- Aspect Ratio:
- Horizontal: 16:9
- Vertical: 9:16
- Square: 1:1
Bumper Ads
- Length: Maximum 6 seconds.
- File Format: MP4, Quicktime, AVI, ASF, Windows Media, or MPEG.
- Resolution:
- Horizontal: 640 x 360px
- Vertical: 480 x 360px
In-feed Ads
- Description: Show alongside YouTube content, like search results or the Home feed.
- Resolution:
- Horizontal: 1920 x 1080px
- Vertical: 1080 x 1920px
- Square: 1080 x 1080px
- Aspect Ratio:
- Horizontal: 16:9
- Square: 1:1
- Length:
- Awareness: 15-20 seconds
- Consideration: 2-3 minutes
- Headline/Description:
- Headline: Up to 2 lines, 40 characters per line
- Description: Up to 2 lines, 35 characters per line
Display Ads
- Description: Static images or animated media that appear on YouTube next to video suggestions, in search results, or on the homepage.
- Image Size: 300×60 pixels.
- File Type: GIF, JPG, PNG.
- File Size: Max 150KB.
- Max Animation Length: 30 seconds.
Outstream Ads
- Description: Mobile-only video ads that appear on websites and apps within the Google video partner network, not on YouTube itself.
- Logo Specs:
- Square: 1:1 (200 x 200px).
- File Type: JPG, GIF, PNG.
- Max Size: 200KB.
Masthead Ads
- Description: High-visibility ads at the top of the YouTube homepage.
- Resolution: 1920 x 1080 or higher.
- File Type: JPG or PNG (without transparency).
Conclusion
YouTube offers a variety of ad formats to reach audiences effectively in 2024. Whether you want to build brand awareness, drive conversions, or target specific demographics, YouTube provides a dynamic platform for your advertising needs. Always follow Google’s advertising policies and the technical ad specs to ensure your ads perform their best. Ready to start using YouTube ads? Contact us today to get started!
MARKETING
Why We Are Always ‘Clicking to Buy’, According to Psychologists
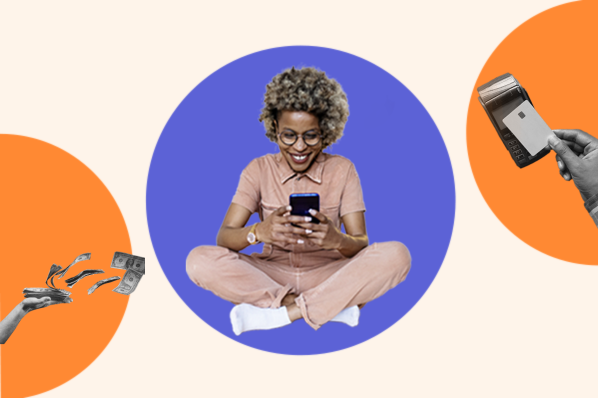
Amazon pillows.
MARKETING
A deeper dive into data, personalization and Copilots
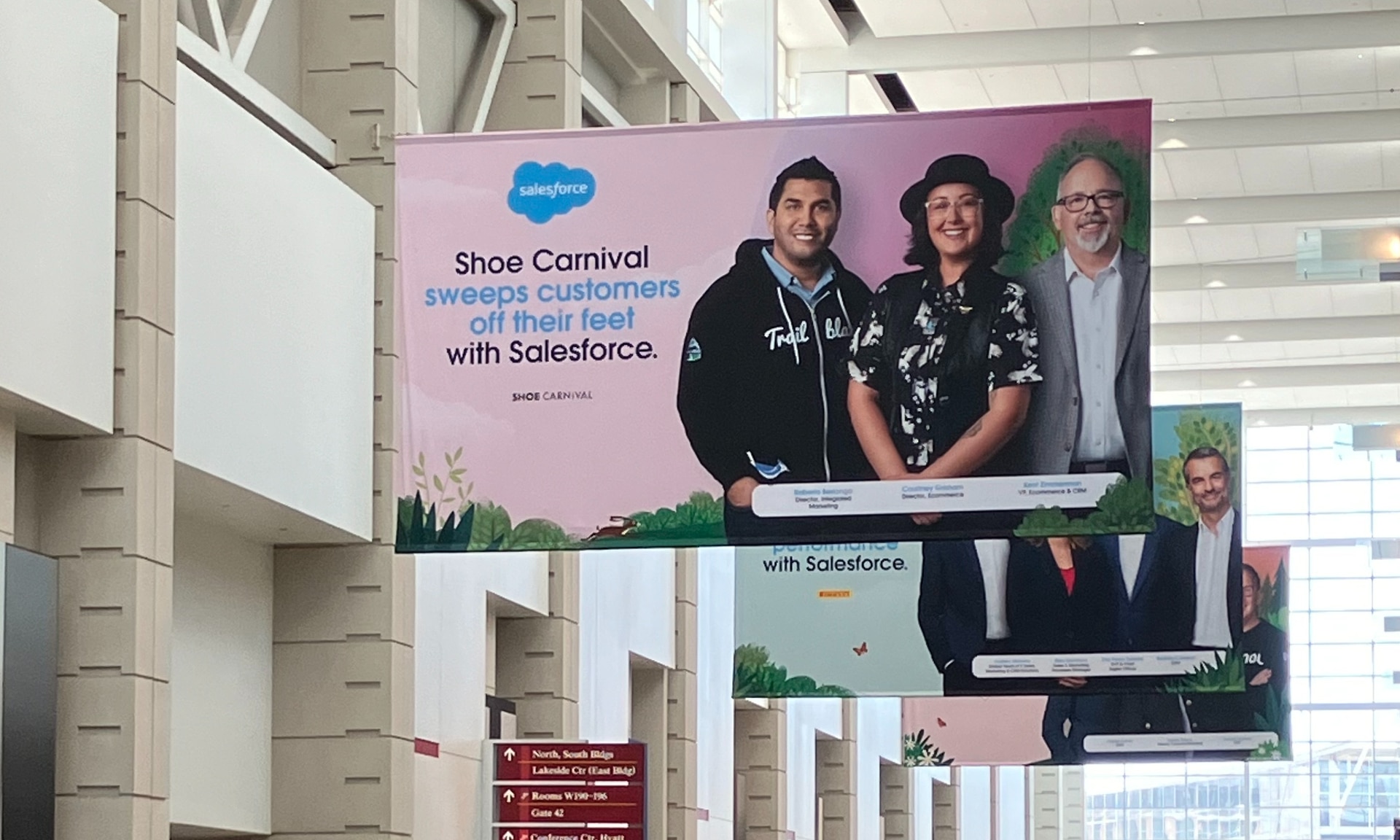
Salesforce launched a collection of new, generative AI-related products at Connections in Chicago this week. They included new Einstein Copilots for marketers and merchants and Einstein Personalization.
To better understand, not only the potential impact of the new products, but the evolving Salesforce architecture, we sat down with Bobby Jania, CMO, Marketing Cloud.
Dig deeper: Salesforce piles on the Einstein Copilots
Salesforce’s evolving architecture
It’s hard to deny that Salesforce likes coming up with new names for platforms and products (what happened to Customer 360?) and this can sometimes make the observer wonder if something is brand new, or old but with a brand new name. In particular, what exactly is Einstein 1 and how is it related to Salesforce Data Cloud?
“Data Cloud is built on the Einstein 1 platform,” Jania explained. “The Einstein 1 platform is our entire Salesforce platform and that includes products like Sales Cloud, Service Cloud — that it includes the original idea of Salesforce not just being in the cloud, but being multi-tenancy.”
Data Cloud — not an acquisition, of course — was built natively on that platform. It was the first product built on Hyperforce, Salesforce’s new cloud infrastructure architecture. “Since Data Cloud was on what we now call the Einstein 1 platform from Day One, it has always natively connected to, and been able to read anything in Sales Cloud, Service Cloud [and so on]. On top of that, we can now bring in, not only structured but unstructured data.”
That’s a significant progression from the position, several years ago, when Salesforce had stitched together a platform around various acquisitions (ExactTarget, for example) that didn’t necessarily talk to each other.
“At times, what we would do is have a kind of behind-the-scenes flow where data from one product could be moved into another product,” said Jania, “but in many of those cases the data would then be in both, whereas now the data is in Data Cloud. Tableau will run natively off Data Cloud; Commerce Cloud, Service Cloud, Marketing Cloud — they’re all going to the same operational customer profile.” They’re not copying the data from Data Cloud, Jania confirmed.
Another thing to know is tit’s possible for Salesforce customers to import their own datasets into Data Cloud. “We wanted to create a federated data model,” said Jania. “If you’re using Snowflake, for example, we more or less virtually sit on your data lake. The value we add is that we will look at all your data and help you form these operational customer profiles.”
Let’s learn more about Einstein Copilot
“Copilot means that I have an assistant with me in the tool where I need to be working that contextually knows what I am trying to do and helps me at every step of the process,” Jania said.
For marketers, this might begin with a campaign brief developed with Copilot’s assistance, the identification of an audience based on the brief, and then the development of email or other content. “What’s really cool is the idea of Einstein Studio where our customers will create actions [for Copilot] that we hadn’t even thought about.”
Here’s a key insight (back to nomenclature). We reported on Copilot for markets, Copilot for merchants, Copilot for shoppers. It turns out, however, that there is just one Copilot, Einstein Copilot, and these are use cases. “There’s just one Copilot, we just add these for a little clarity; we’re going to talk about marketing use cases, about shoppers’ use cases. These are actions for the marketing use cases we built out of the box; you can build your own.”
It’s surely going to take a little time for marketers to learn to work easily with Copilot. “There’s always time for adoption,” Jania agreed. “What is directly connected with this is, this is my ninth Connections and this one has the most hands-on training that I’ve seen since 2014 — and a lot of that is getting people using Data Cloud, using these tools rather than just being given a demo.”
What’s new about Einstein Personalization
Salesforce Einstein has been around since 2016 and many of the use cases seem to have involved personalization in various forms. What’s new?
“Einstein Personalization is a real-time decision engine and it’s going to choose next-best-action, next-best-offer. What is new is that it’s a service now that runs natively on top of Data Cloud.” A lot of real-time decision engines need their own set of data that might actually be a subset of data. “Einstein Personalization is going to look holistically at a customer and recommend a next-best-action that could be natively surfaced in Service Cloud, Sales Cloud or Marketing Cloud.”
Finally, trust
One feature of the presentations at Connections was the reassurance that, although public LLMs like ChatGPT could be selected for application to customer data, none of that data would be retained by the LLMs. Is this just a matter of written agreements? No, not just that, said Jania.
“In the Einstein Trust Layer, all of the data, when it connects to an LLM, runs through our gateway. If there was a prompt that had personally identifiable information — a credit card number, an email address — at a mimum, all that is stripped out. The LLMs do not store the output; we store the output for auditing back in Salesforce. Any output that comes back through our gateway is logged in our system; it runs through a toxicity model; and only at the end do we put PII data back into the answer. There are real pieces beyond a handshake that this data is safe.”