SEO
Visualizing Hot Topics Using Python To Analyze News Sitemaps
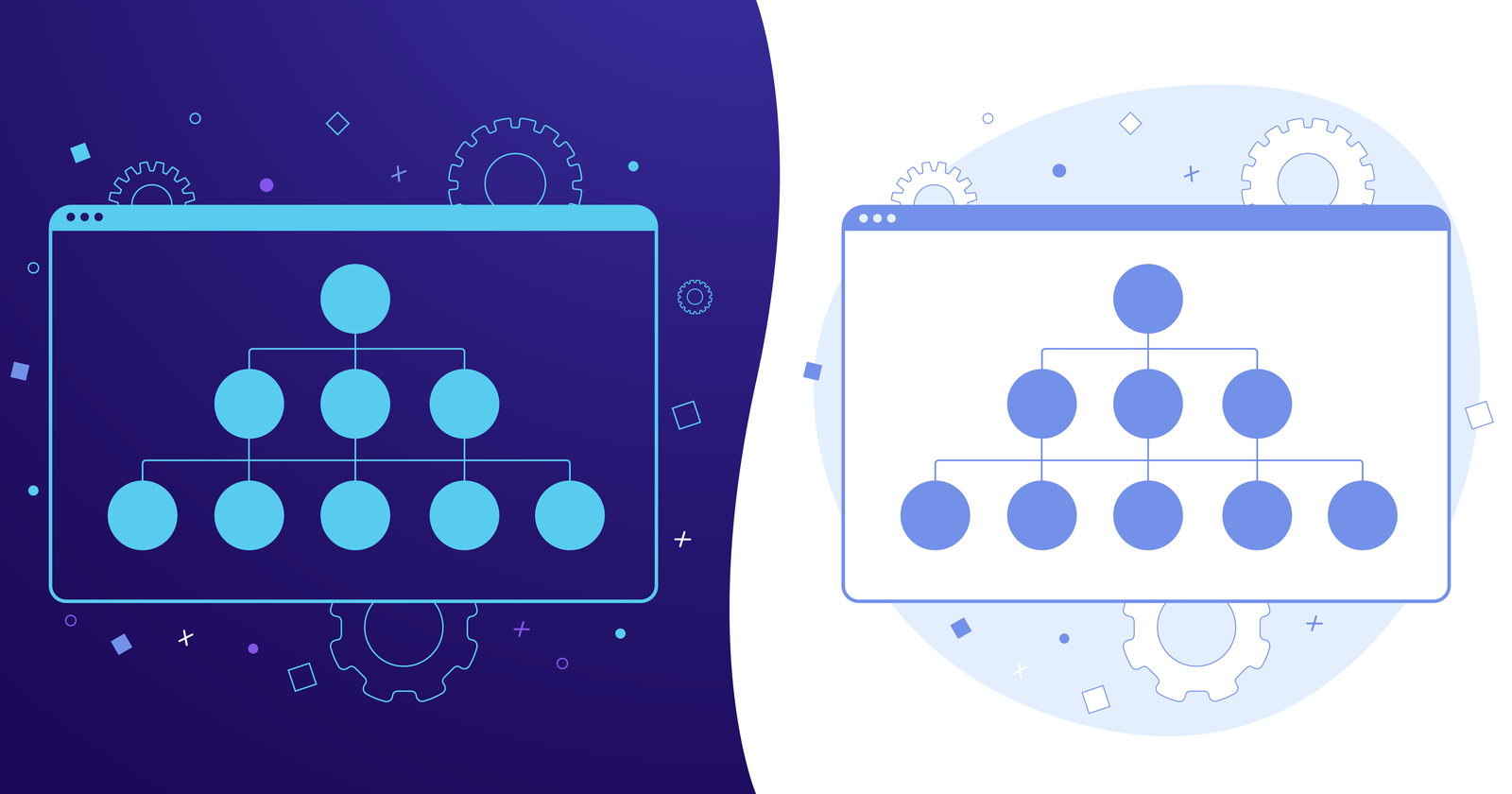
News sitemaps use different and unique sitemap protocols to provide more information for the news search engines.
A news sitemap contains the news published in the last 48 hours.
News sitemap tags include the news publication’s title, language, name, genre, publication date, keywords, and even stock tickers.
How can you use these sitemaps to your advantage for content research and competitive analysis?
In this Python tutorial, you’ll learn a 10-step process for analyzing news sitemaps and visualizing topical trends discovered therein.
Housekeeping Notes To Get Us Started
This tutorial was written during Russia’s invasion of Ukraine.
Using machine learning, we can even label news sources and articles according to which news source is “objective” and which news source is “sarcastic.”
But to keep things simple, we will focus on topics with frequency analysis.
We will use more than 10 global news sources across the U.S. and U.K.
Note: We would like to include Russian news sources, but they do not have a proper news sitemap. Even if they had, they block the external requests.
Comparing the word occurrence of “invasion” and “liberation” from Western and Eastern news sources shows the benefit of distributional frequency text analysis methods.
What You Need To Analyze News Content With Python
The related Python libraries for auditing a news sitemap to understand the news source’s content strategy are listed below:
- Advertools.
- Pandas.
- Plotly Express, Subplots, and Graph Objects.
- Re (Regex).
- String.
- NLTK (Corpus, Stopwords, Ngrams).
- Unicodedata.
- Matplotlib.
- Basic Python Syntax Understanding.
10 Steps For News Sitemap Analysis With Python
All set up? Let’s get to it.
1. Take The News URLs From News Sitemap
We chose the “The Guardian,” “New York Times,” “Washington Post,” “Daily Mail,” “Sky News,” “BBC,” and “CNN” to examine the News URLs from the News Sitemaps.
df_guardian = adv.sitemap_to_df("http://www.theguardian.com/sitemaps/news.xml") df_nyt = adv.sitemap_to_df("https://www.nytimes.com/sitemaps/new/news.xml.gz") df_wp = adv.sitemap_to_df("https://www.washingtonpost.com/arcio/news-sitemap/") df_bbc = adv.sitemap_to_df("https://www.bbc.com/sitemaps/https-index-com-news.xml") df_dailymail = adv.sitemap_to_df("https://www.dailymail.co.uk/google-news-sitemap.xml") df_skynews = adv.sitemap_to_df("https://news.sky.com/sitemap-index.xml") df_cnn = adv.sitemap_to_df("https://edition.cnn.com/sitemaps/cnn/news.xml")
2. Examine An Example News Sitemap With Python
I have used BBC as an example to demonstrate what we just extracted from these news sitemaps.
df_bbc
The BBC Sitemap has the columns below.
df_bbc.columns

The general data structures of these columns are below.
df_bbc.info()

The BBC doesn’t use the “news_publication” column and others.
3. Find The Most Used Words In URLs From News Publications
To see the most used words in the news sites’ URLs, we need to use “str,” “explode”, and “split” methods.
df_dailymail["loc"].str.split("/").str[5].str.split("-").explode().value_counts().to_frame()
loc |
|
---|---|
article |
176 |
Russian |
50 |
Ukraine |
50 |
says |
38 |
reveals |
38 |
... |
... |
readers |
1 |
Red |
1 |
Cross |
1 |
provide |
1 |
weekend.html |
1 |
5445 rows × 1 column
We see that for the “Daily Mail,” “Russia and Ukraine” are the main topic.
4. Find The Most Used Language In News Publications
The URL structure or the “language” section of the news publication can be used to see the most used languages in news publications.
In this sample, we used “BBC” to see their language prioritization.
df_bbc["publication_language"].head(20).value_counts().to_frame()
publication_language | |
en |
698 |
fa |
52 |
sr |
52 |
ar |
47 |
mr |
43 |
hi |
43 |
gu |
41 |
ur |
35 |
pt |
33 |
te |
31 |
ta |
31 |
cy |
30 |
ha |
29 |
tr |
28 |
es |
25 |
sw |
22 |
cpe |
22 |
ne |
21 |
pa |
21 |
yo |
20 |
20 rows × 1 column
To reach out to the Russian population via Google News, every western news source should use the Russian language.
Some international news institutions started to perform this perspective.
If you are a news SEO, it’s helpful to watch Russian language publications from competitors to distribute the objective news to Russia and compete within the news industry.
5. Audit The News Titles For Frequency Of Words
We used BBC to see the “news titles” and which words are more frequent.
df_bbc["news_title"].str.split(" ").explode().value_counts().to_frame()
news_title |
|
---|---|
to |
232 |
in |
181 |
- |
141 |
of |
140 |
for |
138 |
... |
... |
ፊልም |
1 |
ብላክ |
1 |
ባንኪ |
1 |
ጕሒላ |
1 |
niile |
1 |
11916 rows × 1 columns
The problem here is that we have “every type of word in the news titles,” such as “contextless stop words.”
We need to clean these types of non-categorical terms to understand their focus better.
from nltk.corpus import stopwords stop = stopwords.words('english') df_bbc_news_title_most_used_words = df_bbc["news_title"].str.split(" ").explode().value_counts().to_frame() pat = r'b(?:{})b'.format('|'.join(stop)) df_bbc_news_title_most_used_words.reset_index(drop=True, inplace=True) df_bbc_news_title_most_used_words["without_stop_words"] = df_bbc_news_title_most_used_words["words"].str.replace(pat,"") df_bbc_news_title_most_used_words.drop(df_bbc_news_title_most_used_words.loc[df_bbc_news_title_most_used_words["without_stop_words"]==""].index, inplace=True) df_bbc_news_title_most_used_words

We have removed most of the stop words with the help of the “regex” and “replace” method of Pandas.
The second concern is removing the “punctuations.”
For that, we will use the “string” module of Python.
import string df_bbc_news_title_most_used_words["without_stop_word_and_punctation"] = df_bbc_news_title_most_used_words['without_stop_words'].str.replace('[{}]'.format(string.punctuation), '') df_bbc_news_title_most_used_words.drop(df_bbc_news_title_most_used_words.loc[df_bbc_news_title_most_used_words["without_stop_word_and_punctation"]==""].index, inplace=True) df_bbc_news_title_most_used_words.drop(["without_stop_words", "words"], axis=1, inplace=True) df_bbc_news_title_most_used_words
news_title |
without_stop_word_and_punctation |
|
---|---|---|
Ukraine |
110 |
Ukraine |
v |
83 |
v |
de |
61 |
de |
Ukraine: |
60 |
Ukraine |
da |
51 |
da |
... |
... |
... |
ፊልም |
1 |
ፊልም |
ብላክ |
1 |
ብላክ |
ባንኪ |
1 |
ባንኪ |
ጕሒላ |
1 |
ጕሒላ |
niile |
1 |
niile |
11767 rows × 2 columns
Or, use “df_bbc_news_title_most_used_words[“news_title”].to_frame()” to take a more clear picture of data.
news_title |
|
---|---|
Ukraine |
110 |
v |
83 |
de |
61 |
Ukraine: |
60 |
da |
51 |
... |
... |
ፊልም |
1 |
ብላክ |
1 |
ባንኪ |
1 |
ጕሒላ |
1 |
niile |
1 |
11767 rows × 1 columns
We see 11,767 unique words in the URLs of the BBC, and Ukraine is the most popular, with 110 occurrences.
There are different Ukraine-related phrases from the data frame, such as “Ukraine:.”
The “NLTK Tokenize” can be used to unite these types of different variations.
The next section will use a different method to unite them.
Note: If you want to make things easier, use Advertools as below.
adv.word_frequency(df_bbc["news_title"],phrase_len=2, rm_words=adv.stopwords.keys())
The result is below.

“adv.word_frequency” has the attributes “phrase_len” and “rm_words” to determine the length of the phrase occurrence and remove the stop words.
You may tell me, why didn’t I use it in the first place?
I wanted to show you an educational example with “regex, NLTK, and the string” so that you can understand what’s happening behind the scenes.
6. Visualize The Most Used Words In News Titles
To visualize the most used words in the news titles, you can use the code block below.
df_bbc_news_title_most_used_words["news_title"] = df_bbc_news_title_most_used_words["news_title"].astype(int) df_bbc_news_title_most_used_words["without_stop_word_and_punctation"] = df_bbc_news_title_most_used_words["without_stop_word_and_punctation"].astype(str) df_bbc_news_title_most_used_words.index = df_bbc_news_title_most_used_words["without_stop_word_and_punctation"] df_bbc_news_title_most_used_words["news_title"].head(20).plot(title="The Most Used Words in BBC News Titles")

You realize that there is a “broken line.”
Do you remember the “Ukraine” and “Ukraine:” in the data frame?
When we remove the “punctuation,” the second and first values become the same.
That’s why the line graph says that Ukraine appeared 60 times and 110 times separately.
To prevent such a data discrepancy, use the code block below.
df_bbc_news_title_most_used_words_1 = df_bbc_news_title_most_used_words.drop_duplicates().groupby('without_stop_word_and_punctation', sort=False, as_index=True).sum() df_bbc_news_title_most_used_words_1
news_title |
|
---|---|
without_stop_word_and_punctation |
|
Ukraine |
175 |
v |
83 |
de |
61 |
da |
51 |
и |
41 |
... |
... |
ፊልም |
1 |
ብላክ |
1 |
ባንኪ |
1 |
ጕሒላ |
1 |
niile |
1 |
11109 rows × 1 columns
The duplicated rows are dropped, and their values are summed together.
Now, let’s visualize it again.
7. Extract Most Popular N-Grams From News Titles
Extracting n-grams from the news titles or normalizing the URL words and forming n-grams for understanding the overall topicality is useful to understand which news publication approaches which topic. Here’s how.
import nltk import unicodedata import re def text_clean(content):
lemmetizer = nltk.stem.WordNetLemmatizer() stopwords = nltk.corpus.stopwords.words('english') content = (unicodedata.normalize('NFKD', content) .encode('ascii', 'ignore') .decode('utf-8', 'ignore') .lower()) words = re.sub(r'[^ws]', '', content).split() return [lemmetizer.lemmatize(word) for word in words if word not in stopwords]
raw_words = text_clean(''.join(str(df_bbc['news_title'].tolist())))
raw_words[:10]
OUTPUT>>> ['oneminute', 'world', 'news', 'best', 'generation', 'make', 'agyarkos', 'dream', 'fight', 'card']
The output shows we have “lemmatized” all the words in the news titles and put them in a list.
The list comprehension provides a quick shortcut for filtering every stop word easily.
Using “nltk.corpus.stopwords.words(“english”)” provides all the stop words in English.
But you can add extra stop words to the list to expand the exclusion of words.
The “unicodedata” is to canonicalize the characters.
The characters that we see are actually Unicode bytes like “U+2160 ROMAN NUMERAL ONE” and the Roman Character “U+0049 LATIN CAPITAL LETTER I” are actually the same.
The “unicodedata.normalize” distinguishes the character differences so that the lemmatizer can differentiate the different words with similar characters from each other.
pd.set_option("display.max_colwidth",90) bbc_bigrams = (pd.Series(ngrams(words, n = 2)).value_counts())[:15].sort_values(ascending=False).to_frame() bbc_trigrams = (pd.Series(ngrams(words, n = 3)).value_counts())[:15].sort_values(ascending=False).to_frame()
Below, you will see the most popular “n-grams” from BBC News.

To simply visualize the most popular n-grams of a news source, use the code block below.
bbc_bigrams.plot.barh(color="red", width=.8,figsize=(10 , 7))
“Ukraine, war” is the trending news.
You can also filter the n-grams for “Ukraine” and create an “entity-attribute” pair.

Crawling these URLs and recognizing the “person type entities” can give you an idea about how BBC approaches newsworthy situations.
But it is beyond “news sitemaps.” Thus, it is for another day.
To visualize the popular n-grams from news source’s sitemaps, you can create a custom python function as below.
def ngram_visualize(dataframe:pd.DataFrame, color:str="blue") -> pd.DataFrame.plot: dataframe.plot.barh(color=color, width=.8,figsize=(10 ,7)) ngram_visualize(ngram_extractor(df_dailymail))
The result is below.

To make it interactive, add an extra parameter as below.
def ngram_visualize(dataframe:pd.DataFrame, backend:str, color:str="blue", ) -> pd.DataFrame.plot: if backend=="plotly": pd.options.plotting.backend=backend return dataframe.plot.bar() else: return dataframe.plot.barh(color=color, width=.8,figsize=(10 ,7))
ngram_visualize(ngram_extractor(df_dailymail), backend="plotly")
As a quick example, check below.
8. Create Your Own Custom Functions To Analyze The News Source Sitemaps
When you audit news sitemaps repeatedly, there will be a need for a small Python package.
Below, you can find four different quick Python function chain that uses every previous function as a callback.
To clean a textual content item, use the function below.
def text_clean(content): lemmetizer = nltk.stem.WordNetLemmatizer() stopwords = nltk.corpus.stopwords.words('english') content = (unicodedata.normalize('NFKD', content) .encode('ascii', 'ignore') .decode('utf-8', 'ignore') .lower()) words = re.sub(r'[^ws]', '', content).split() return [lemmetizer.lemmatize(word) for word in words if word not in stopwords]
To extract the n-grams from a specific news website’s sitemap’s news titles, use the function below.
def ngram_extractor(dataframe:pd.DataFrame|pd.Series): if "news_title" in dataframe.columns: return dataframe_ngram_extractor(dataframe, ngram=3, first=10)
Use the function below to turn the extracted n-grams into a data frame.
def dataframe_ngram_extractor(dataframe:pd.DataFrame|pd.Series, ngram:int, first:int): raw_words = text_clean(''.join(str(dataframe['news_title'].tolist()))) return (pd.Series(ngrams(raw_words, n = ngram)).value_counts())[:first].sort_values(ascending=False).to_frame()
To extract multiple news websites’ sitemaps, use the function below.
def ngram_df_constructor(df_1:pd.DataFrame, df_2:pd.DataFrame): df_1_bigrams = dataframe_ngram_extractor(df_1, ngram=2, first=500) df_1_trigrams = dataframe_ngram_extractor(df_1, ngram=3, first=500) df_2_bigrams = dataframe_ngram_extractor(df_2, ngram=2, first=500) df_2_trigrams = dataframe_ngram_extractor(df_2, ngram=3, first=500) ngrams_df = { "df_1_bigrams":df_1_bigrams.index, "df_1_trigrams": df_1_trigrams.index, "df_2_bigrams":df_2_bigrams.index, "df_2_trigrams": df_2_trigrams.index, } dict_df = (pd.DataFrame({ key:pd.Series(value) for key, value in ngrams_df.items() }).reset_index(drop=True) .rename(columns={"df_1_bigrams":adv.url_to_df(df_1["loc"])["netloc"][1].split("www.")[1].split(".")[0] + "_bigrams", "df_1_trigrams":adv.url_to_df(df_1["loc"])["netloc"][1].split("www.")[1].split(".")[0] + "_trigrams", "df_2_bigrams": adv.url_to_df(df_2["loc"])["netloc"][1].split("www.")[1].split(".")[0] + "_bigrams", "df_2_trigrams": adv.url_to_df(df_2["loc"])["netloc"][1].split("www.")[1].split(".")[0] + "_trigrams"})) return dict_df
Below, you can see an example use case.
ngram_df_constructor(df_bbc, df_guardian)

Only with these nested four custom python functions can you do the things below.
- Easily, you can visualize these n-grams and the news website counts to check.
- You can see the focus of the news websites for the same topic or different topics.
- You can compare their wording or the vocabulary for the same topics.
- You can see how many different sub-topics from the same topics or entities are processed in a comparative way.
I didn’t put the numbers for the frequencies of the n-grams.
But, the first ranked ones are the most popular ones from that specific news source.
To examine the next 500 rows, click here.
9. Extract The Most Used News Keywords From News Sitemaps
When it comes to news keywords, they are surprisingly still active on Google.
For example, Microsoft Bing and Google do not think that “meta keywords” are a useful signal anymore, unlike Yandex.
But, news keywords from the news sitemaps are still used.
Among all these news sources, only The Guardian uses the news keywords.
And understanding how they use news keywords to provide relevance is useful.
df_guardian["news_keywords"].str.split().explode().value_counts().to_frame().rename(columns={"news_keywords":"news_keyword_occurence"})
You can see the most used words in the news keywords for The Guardian.
news_keyword_occurence |
|
---|---|
news, |
250 |
World |
142 |
and |
142 |
Ukraine, |
127 |
UK |
116 |
... |
... |
Cumberbatch, |
1 |
Dune |
1 |
Saracens |
1 |
Pearson, |
1 |
Thailand |
1 |
1409 rows × 1 column
The visualization is below.
(df_guardian["news_keywords"].str.split().explode().value_counts() .to_frame().rename(columns={"news_keywords":"news_keyword_occurence"}) .head(25).plot.barh(figsize=(10,8), title="The Guardian Most Used Words in News Keywords", xlabel="News Keywords", legend=False, ylabel="Count of News Keyword"))

The “,” at the end of the news keywords represent whether it is a separate value or part of another.
I suggest you not remove the “punctuations” or “stop words” from news keywords so that you can see their news keyword usage style better.
For a different analysis, you can use “,” as a separator.
df_guardian["news_keywords"].str.split(",").explode().value_counts().to_frame().rename(columns={"news_keywords":"news_keyword_occurence"})
The result difference is below.
news_keyword_occurence |
|
---|---|
World news |
134 |
Europe |
116 |
UK news |
111 |
Sport |
109 |
Russia |
90 |
... |
... |
Women's shoes |
1 |
Men's shoes |
1 |
Body image |
1 |
Kae Tempest |
1 |
Thailand |
1 |
1080 rows × 1 column
Focus on the “split(“,”).”
(df_guardian["news_keywords"].str.split(",").explode().value_counts() .to_frame().rename(columns={"news_keywords":"news_keyword_occurence"}) .head(25).plot.barh(figsize=(10,8), title="The Guardian Most Used Words in News Keywords", xlabel="News Keywords", legend=False, ylabel="Count of News Keyword"))
You can see the result difference for visualization below.

From “Chelsea” to “Vladamir Putin” or “Ukraine War” and “Roman Abramovich,” most of these phrases align with the early days of Russia’s Invasion of Ukraine.
Use the code block below to visualize two different news website sitemaps’ news keywords interactively.
df_1 = df_guardian["news_keywords"].str.split(",").explode().value_counts().to_frame().rename(columns={"news_keywords":"news_keyword_occurence"}) df_2 = df_nyt["news_keywords"].str.split(",").explode().value_counts().to_frame().rename(columns={"news_keywords":"news_keyword_occurence"}) fig = make_subplots(rows = 1, cols = 2) fig.add_trace( go.Bar(y = df_1["news_keyword_occurence"][:6].index, x = df_1["news_keyword_occurence"], orientation="h", name="The Guardian News Keywords"), row=1, col=2 ) fig.add_trace( go.Bar(y = df_2["news_keyword_occurence"][:6].index, x = df_2["news_keyword_occurence"], orientation="h", name="New York Times News Keywords"), row=1, col=1 ) fig.update_layout(height = 800, width = 1200, title_text="Side by Side Popular News Keywords") fig.show() fig.write_html("news_keywords.html")
You can see the result below.
To interact with the live chart, click here.
In the next section, you will find two different subplot samples to compare the n-grams of the news websites.
10. Create Subplots For Comparing News Sources
Use the code block below to put the news sources’ most popular n-grams from the news titles to a sub-plot.
import matplotlib.pyplot as plt import pandas as pd df1 = ngram_extractor(df_bbc) df2 = ngram_extractor(df_skynews) df3 = ngram_extractor(df_dailymail) df4 = ngram_extractor(df_guardian) df5 = ngram_extractor(df_nyt) df6 = ngram_extractor(df_cnn) nrow=3 ncol=2 df_list = [df1 ,df2, df3, df4, df5, df6] #df6 titles = ["BBC News Trigrams", "Skynews Trigrams", "Dailymail Trigrams", "The Guardian Trigrams", "New York Times Trigrams", "CNN News Ngrams"] fig, axes = plt.subplots(nrow, ncol, figsize=(25,32)) count=0 i = 0 for r in range(nrow): for c in range(ncol): (df_list[count].plot.barh(ax = axes[r,c], figsize = (40, 28), title = titles[i], fontsize = 10, legend = False, xlabel = "Trigrams", ylabel = "Count")) count+=1 i += 1
You can see the result below.

The example data visualization above is entirely static and doesn’t provide any interactivity.
Lately, Elias Dabbas, creator of Advertools, has shared a new script to take the article count, n-grams, and their counts from the news sources.
Check here for a better, more detailed, and interactive data dashboard.
The example above is from Elias Dabbas, and he demonstrates how to take the total article count, most frequent words, and n-grams from news websites in an interactive way.
Final Thoughts On News Sitemap Analysis With Python
This tutorial was designed to provide an educational Python coding session to take the keywords, n-grams, phrase patterns, languages, and other kinds of SEO-related information from news websites.
News SEO heavily relies on quick reflexes and always-on article creation.
Tracking your competitors’ angles and methods for covering a topic shows how the competitors have quick reflexes for the search trends.
Creating a Google Trends Dashboard and News Source Ngram Tracker for a comparative and complementary news SEO analysis would be better.
In this article, from time to time, I have put custom functions or advanced for loops, and sometimes, I have kept things simple.
Beginners to advanced Python practitioners can benefit from it to improve their tracking, reporting, and analyzing methodologies for news SEO and beyond.
More resources:
Featured Image: BestForBest/Shutterstock
SEO
How to Revive an Old Blog Article for SEO
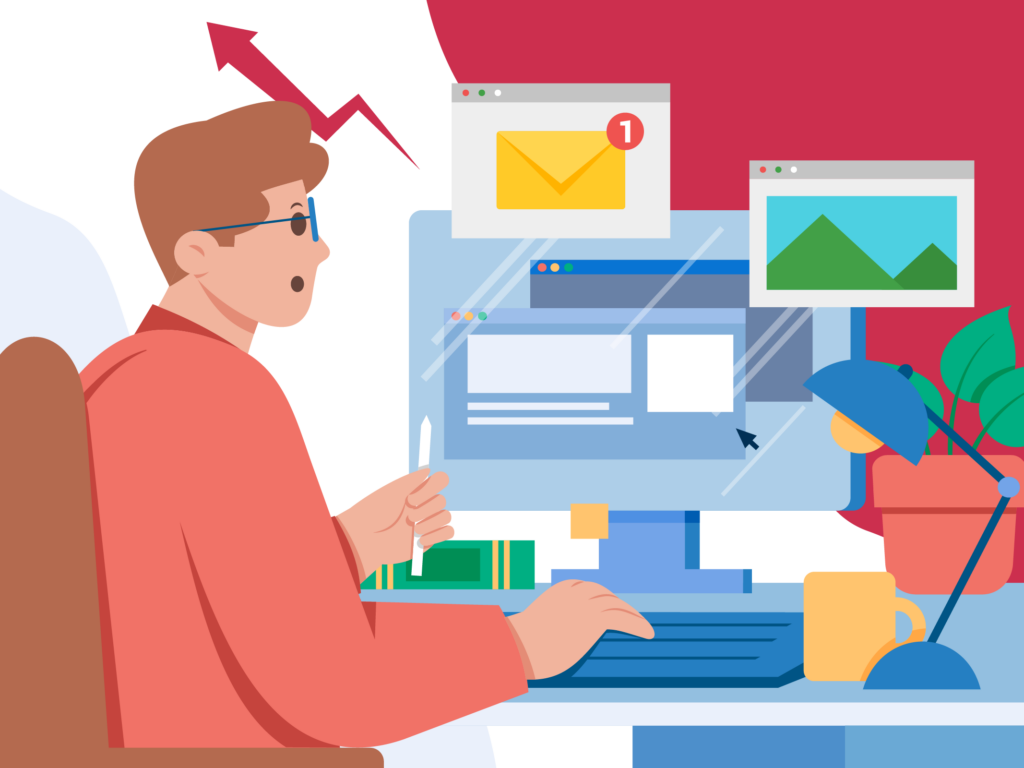
Quick question: What do you typically do with your old blog posts? Most likely, the answer is: Not much.
If that’s the case, you’re not alone. Many of us in SEO and content marketing tend to focus on continuously creating new content, rather than leveraging our existing blog posts.
However, here’s the reality—Google is becoming increasingly sophisticated in evaluating content quality, and we need to adapt accordingly. Just as it’s easier to encourage existing customers to make repeat purchases, updating old content on your website is a more efficient and sustainable strategy in the long run.
Ways to Optimize Older Content
Some of your old content might not be optimized for SEO very well, rank for irrelevant keywords, or drive no traffic at all. If the quality is still decent, however, you should be able to optimize it properly with little effort.
Refresh Content
If your blog post contains a specific year or mentions current events, it may become outdated over time. If the rest of the content is still relevant (like if it’s targeting an evergreen topic), simply updating the date might be all you need to do.
Rewrite Old Blog Posts
When the content quality is low (you might have greatly improved your writing skills since you’ve written the post) but the potential is still there, there’s not much you can do apart from rewriting an old blog post completely.
This is not a waste—you’re saving time on brainstorming since the basic structure is already in place. Now, focus on improving the quality.
Delete Old Blog Posts
You might find a blog post that just seems unusable. Should you delete your old content? It depends. If it’s completely outdated, of low quality, and irrelevant to any valuable keywords for your website, it’s better to remove it.
Once you decide to delete the post, don’t forget to set up a 301 redirect to a related post or page, or to your homepage.
Promote Old Blog Posts
Sometimes all your content needs is a bit of promotion to start ranking and getting traffic again. Share it on your social media, link to it from a new post – do something to get it discoverable again to your audience. This can give it the boost it needs to attract organic links too.
Which Blog Posts Should You Update?
Deciding when to update or rewrite blog posts is a decision that relies on one important thing: a content audit.
Use your Google Analytics to find out which blog posts used to drive tons of traffic, but no longer have the same reach. You can also use Google Search Console to find out which of your blog posts have lost visibility in comparison to previous months. I have a guide on website analysis using Google Analytics and Google Search Console you can follow.
If you use keyword tracking tools like SE Ranking, you can also use the data it provides to come up with a list of blog posts that have dropped in the rankings.
Make data-driven decisions to identify which blog posts would benefit from these updates – i.e., which ones still have the chance to recover their keyword rankings and organic traffic.
With Google’s helpful content update, which emphasizes better user experiences, it’s crucial to ensure your content remains relevant, valuable, and up-to-date.
How To Update Old Blog Posts for SEO
Updating articles can be an involved process. Here are some tips and tactics to help you get it right.
Author’s Note: I have a Comprehensive On-Page SEO Checklist you might also be interested in following while you’re doing your content audit.
Conduct New Keyword Research
Updating your post without any guide won’t get you far. Always do your keyword research to understand how users are searching for your given topic.
Proper research can also show you relevant questions and sections that can be added to the blog post you’re updating or rewriting. Make sure to take a look at the People Also Ask (PAA) section that shows up when you search for your target keyword. Check out other websites like Answer The Public, Reddit, and Quora to see what users are looking for too.
Look for New Ranking Opportunities
When trying to revive an old blog post for SEO, keep an eye out for new SEO opportunities (e.g., AI Overview, featured snippets, and related search terms) that didn’t exist when you first wrote your blog post. Some of these features can be targeted by the new content you will add to your post, if you write with the aim to be eligible for it.
Rewrite Headlines and Meta Tags
If you want to attract new readers, consider updating your headlines and meta tags.
Your headlines and meta tags should fulfill these three things:
- Reflect the rewritten and new content you’ve added to the blog post.
- Be optimized for the new keywords it’s targeting (if any).
- Appeal to your target audience – who may have changed tastes from when the blog post was originally made.
Remember that your meta tags in particular act like a brief advertisement for your blog post, since this is what the user first sees when your blog post is shown in the search results page.
Take a look at your blog post’s click-through rate on Google Search Console – if it falls below 2%, it’s definitely time for new meta tags.
Replace Outdated Information and Statistics
Updating blog content with current studies and statistics enhances the relevance and credibility of your post. By providing up-to-date information, you help your audience make better, well-informed decisions, while also showing that your content is trustworthy.
Tighten or Expand Ideas
Your old content might be too short to provide real value to users – or you might have rambled on and on in your post. It’s important to evaluate whether you need to make your content more concise, or if you need to elaborate more.
Keep the following tips in mind as you refine your blog post’s ideas:
- Evaluate Helpfulness: Measure how well your content addresses your readers’ pain points. Aim to follow the E-E-A-T model (Experience, Expertise, Authoritativeness, Trustworthiness).
- Identify Missing Context: Consider whether your content needs more detail or clarification. View it from your audience’s perspective and ask if the information is complete, or if more information is needed.
- Interview Experts: Speak with industry experts or thought leaders to get fresh insights. This will help support your writing, and provide unique points that enhance the value of your content.
- Use Better Examples: Examples help simplify complex concepts. Add new examples or improve existing ones to strengthen your points.
- Add New Sections if Needed: If your content lacks depth or misses a key point, add new sections to cover these areas more thoroughly.
- Remove Fluff: Every sentence should contribute to the overall narrative. Eliminate unnecessary content to make your post more concise.
- Revise Listicles: Update listicle items based on SEO recommendations and content quality. Add or remove headings to stay competitive with higher-ranking posts.
Improve Visuals and Other Media
No doubt that there are tons of old graphics and photos in your blog posts that can be improved with the tools we have today. Make sure all of the visuals used in your content are appealing and high quality.
Update Internal and External Links
Are your internal and external links up to date? They need to be for your SEO and user experience. Outdated links can lead to broken pages or irrelevant content, frustrating readers and hurting your site’s performance.
You need to check for any broken links on your old blog posts, and update them ASAP. Updating your old blog posts can also lead to new opportunities to link internally to other blog posts and pages, which may not have been available when the post was originally published.
Optimize for Conversions
When updating content, the ultimate goal is often to increase conversions. However, your conversion goals may have changed over the years.
So here’s what you need to check in your updated blog post. First, does the call-to-action (CTA) still link to the products or services you want to promote? If not, update it to direct readers to the current solution or offer.
Second, consider where you can use different conversion strategies. Don’t just add a CTA at the end of the post.
Last, make sure that the blog post leverages product-led content. It’s going to help you mention your products and services in a way that feels natural, without being too pushy. Being subtle can be a high ROI tactic for updated posts.
Key Takeaway
Reviving old blog articles for SEO is a powerful strategy that can breathe new life into your content and boost your website’s visibility. Instead of solely focusing on creating new posts, taking the time to refresh existing content can yield impressive results, both in terms of traffic and conversions.
By implementing these strategies, you can transform old blog posts into valuable resources that attract new readers and retain existing ones. So, roll up your sleeves, dive into your archives, and start updating your content today—your audience and search rankings will thank you!
SEO
How Compression Can Be Used To Detect Low Quality Pages
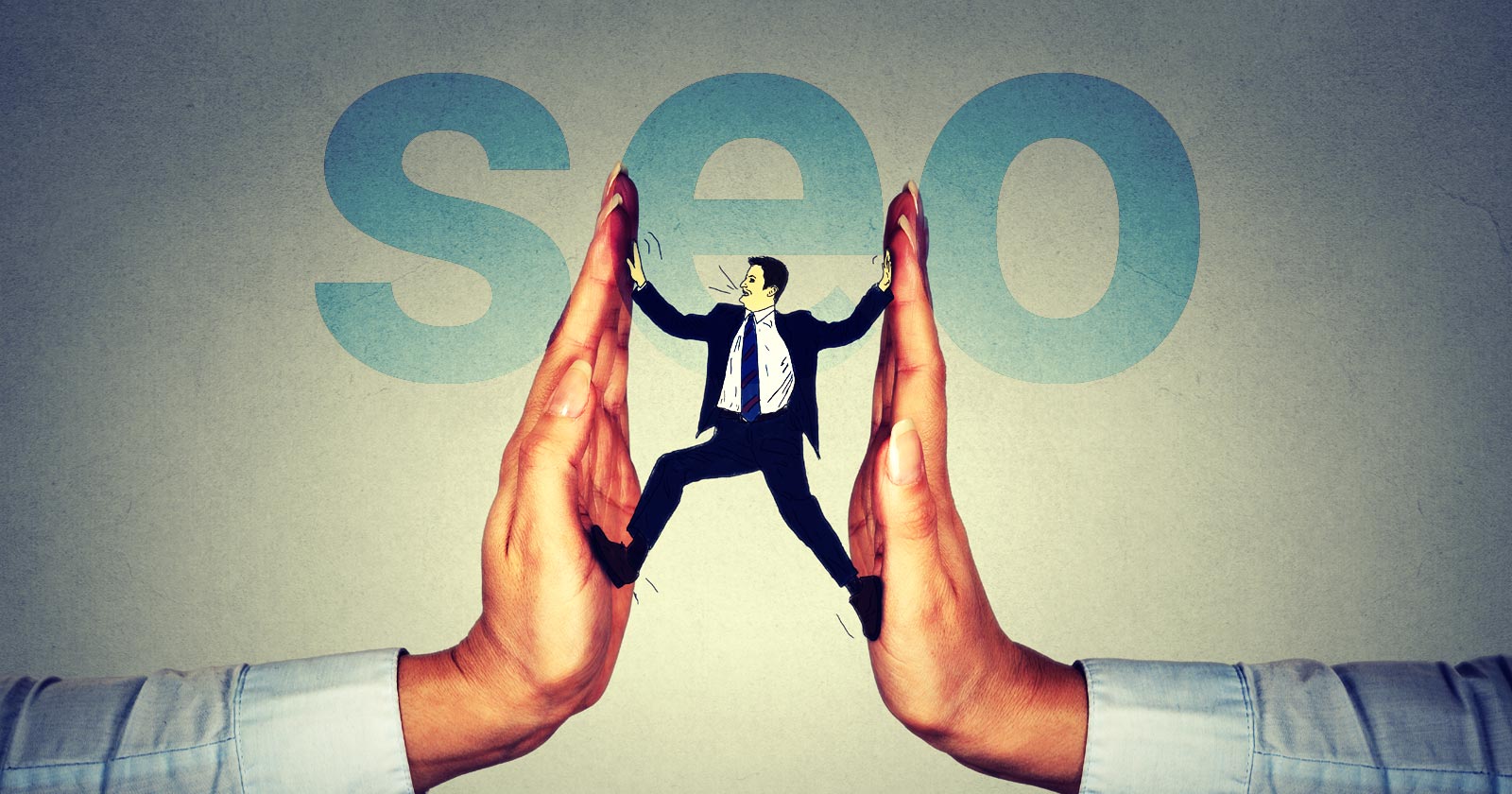
The concept of Compressibility as a quality signal is not widely known, but SEOs should be aware of it. Search engines can use web page compressibility to identify duplicate pages, doorway pages with similar content, and pages with repetitive keywords, making it useful knowledge for SEO.
Although the following research paper demonstrates a successful use of on-page features for detecting spam, the deliberate lack of transparency by search engines makes it difficult to say with certainty if search engines are applying this or similar techniques.
What Is Compressibility?
In computing, compressibility refers to how much a file (data) can be reduced in size while retaining essential information, typically to maximize storage space or to allow more data to be transmitted over the Internet.
TL/DR Of Compression
Compression replaces repeated words and phrases with shorter references, reducing the file size by significant margins. Search engines typically compress indexed web pages to maximize storage space, reduce bandwidth, and improve retrieval speed, among other reasons.
This is a simplified explanation of how compression works:
- Identify Patterns:
A compression algorithm scans the text to find repeated words, patterns and phrases - Shorter Codes Take Up Less Space:
The codes and symbols use less storage space then the original words and phrases, which results in a smaller file size. - Shorter References Use Less Bits:
The “code” that essentially symbolizes the replaced words and phrases uses less data than the originals.
A bonus effect of using compression is that it can also be used to identify duplicate pages, doorway pages with similar content, and pages with repetitive keywords.
Research Paper About Detecting Spam
This research paper is significant because it was authored by distinguished computer scientists known for breakthroughs in AI, distributed computing, information retrieval, and other fields.
Marc Najork
One of the co-authors of the research paper is Marc Najork, a prominent research scientist who currently holds the title of Distinguished Research Scientist at Google DeepMind. He’s a co-author of the papers for TW-BERT, has contributed research for increasing the accuracy of using implicit user feedback like clicks, and worked on creating improved AI-based information retrieval (DSI++: Updating Transformer Memory with New Documents), among many other major breakthroughs in information retrieval.
Dennis Fetterly
Another of the co-authors is Dennis Fetterly, currently a software engineer at Google. He is listed as a co-inventor in a patent for a ranking algorithm that uses links, and is known for his research in distributed computing and information retrieval.
Those are just two of the distinguished researchers listed as co-authors of the 2006 Microsoft research paper about identifying spam through on-page content features. Among the several on-page content features the research paper analyzes is compressibility, which they discovered can be used as a classifier for indicating that a web page is spammy.
Detecting Spam Web Pages Through Content Analysis
Although the research paper was authored in 2006, its findings remain relevant to today.
Then, as now, people attempted to rank hundreds or thousands of location-based web pages that were essentially duplicate content aside from city, region, or state names. Then, as now, SEOs often created web pages for search engines by excessively repeating keywords within titles, meta descriptions, headings, internal anchor text, and within the content to improve rankings.
Section 4.6 of the research paper explains:
“Some search engines give higher weight to pages containing the query keywords several times. For example, for a given query term, a page that contains it ten times may be higher ranked than a page that contains it only once. To take advantage of such engines, some spam pages replicate their content several times in an attempt to rank higher.”
The research paper explains that search engines compress web pages and use the compressed version to reference the original web page. They note that excessive amounts of redundant words results in a higher level of compressibility. So they set about testing if there’s a correlation between a high level of compressibility and spam.
They write:
“Our approach in this section to locating redundant content within a page is to compress the page; to save space and disk time, search engines often compress web pages after indexing them, but before adding them to a page cache.
…We measure the redundancy of web pages by the compression ratio, the size of the uncompressed page divided by the size of the compressed page. We used GZIP …to compress pages, a fast and effective compression algorithm.”
High Compressibility Correlates To Spam
The results of the research showed that web pages with at least a compression ratio of 4.0 tended to be low quality web pages, spam. However, the highest rates of compressibility became less consistent because there were fewer data points, making it harder to interpret.
Figure 9: Prevalence of spam relative to compressibility of page.
The researchers concluded:
“70% of all sampled pages with a compression ratio of at least 4.0 were judged to be spam.”
But they also discovered that using the compression ratio by itself still resulted in false positives, where non-spam pages were incorrectly identified as spam:
“The compression ratio heuristic described in Section 4.6 fared best, correctly identifying 660 (27.9%) of the spam pages in our collection, while misidentifying 2, 068 (12.0%) of all judged pages.
Using all of the aforementioned features, the classification accuracy after the ten-fold cross validation process is encouraging:
95.4% of our judged pages were classified correctly, while 4.6% were classified incorrectly.
More specifically, for the spam class 1, 940 out of the 2, 364 pages, were classified correctly. For the non-spam class, 14, 440 out of the 14,804 pages were classified correctly. Consequently, 788 pages were classified incorrectly.”
The next section describes an interesting discovery about how to increase the accuracy of using on-page signals for identifying spam.
Insight Into Quality Rankings
The research paper examined multiple on-page signals, including compressibility. They discovered that each individual signal (classifier) was able to find some spam but that relying on any one signal on its own resulted in flagging non-spam pages for spam, which are commonly referred to as false positive.
The researchers made an important discovery that everyone interested in SEO should know, which is that using multiple classifiers increased the accuracy of detecting spam and decreased the likelihood of false positives. Just as important, the compressibility signal only identifies one kind of spam but not the full range of spam.
The takeaway is that compressibility is a good way to identify one kind of spam but there are other kinds of spam that aren’t caught with this one signal. Other kinds of spam were not caught with the compressibility signal.
This is the part that every SEO and publisher should be aware of:
“In the previous section, we presented a number of heuristics for assaying spam web pages. That is, we measured several characteristics of web pages, and found ranges of those characteristics which correlated with a page being spam. Nevertheless, when used individually, no technique uncovers most of the spam in our data set without flagging many non-spam pages as spam.
For example, considering the compression ratio heuristic described in Section 4.6, one of our most promising methods, the average probability of spam for ratios of 4.2 and higher is 72%. But only about 1.5% of all pages fall in this range. This number is far below the 13.8% of spam pages that we identified in our data set.”
So, even though compressibility was one of the better signals for identifying spam, it still was unable to uncover the full range of spam within the dataset the researchers used to test the signals.
Combining Multiple Signals
The above results indicated that individual signals of low quality are less accurate. So they tested using multiple signals. What they discovered was that combining multiple on-page signals for detecting spam resulted in a better accuracy rate with less pages misclassified as spam.
The researchers explained that they tested the use of multiple signals:
“One way of combining our heuristic methods is to view the spam detection problem as a classification problem. In this case, we want to create a classification model (or classifier) which, given a web page, will use the page’s features jointly in order to (correctly, we hope) classify it in one of two classes: spam and non-spam.”
These are their conclusions about using multiple signals:
“We have studied various aspects of content-based spam on the web using a real-world data set from the MSNSearch crawler. We have presented a number of heuristic methods for detecting content based spam. Some of our spam detection methods are more effective than others, however when used in isolation our methods may not identify all of the spam pages. For this reason, we combined our spam-detection methods to create a highly accurate C4.5 classifier. Our classifier can correctly identify 86.2% of all spam pages, while flagging very few legitimate pages as spam.”
Key Insight:
Misidentifying “very few legitimate pages as spam” was a significant breakthrough. The important insight that everyone involved with SEO should take away from this is that one signal by itself can result in false positives. Using multiple signals increases the accuracy.
What this means is that SEO tests of isolated ranking or quality signals will not yield reliable results that can be trusted for making strategy or business decisions.
Takeaways
We don’t know for certain if compressibility is used at the search engines but it’s an easy to use signal that combined with others could be used to catch simple kinds of spam like thousands of city name doorway pages with similar content. Yet even if the search engines don’t use this signal, it does show how easy it is to catch that kind of search engine manipulation and that it’s something search engines are well able to handle today.
Here are the key points of this article to keep in mind:
- Doorway pages with duplicate content is easy to catch because they compress at a higher ratio than normal web pages.
- Groups of web pages with a compression ratio above 4.0 were predominantly spam.
- Negative quality signals used by themselves to catch spam can lead to false positives.
- In this particular test, they discovered that on-page negative quality signals only catch specific types of spam.
- When used alone, the compressibility signal only catches redundancy-type spam, fails to detect other forms of spam, and leads to false positives.
- Combing quality signals improves spam detection accuracy and reduces false positives.
- Search engines today have a higher accuracy of spam detection with the use of AI like Spam Brain.
Read the research paper, which is linked from the Google Scholar page of Marc Najork:
Detecting spam web pages through content analysis
Featured Image by Shutterstock/pathdoc
SEO
New Google Trends SEO Documentation
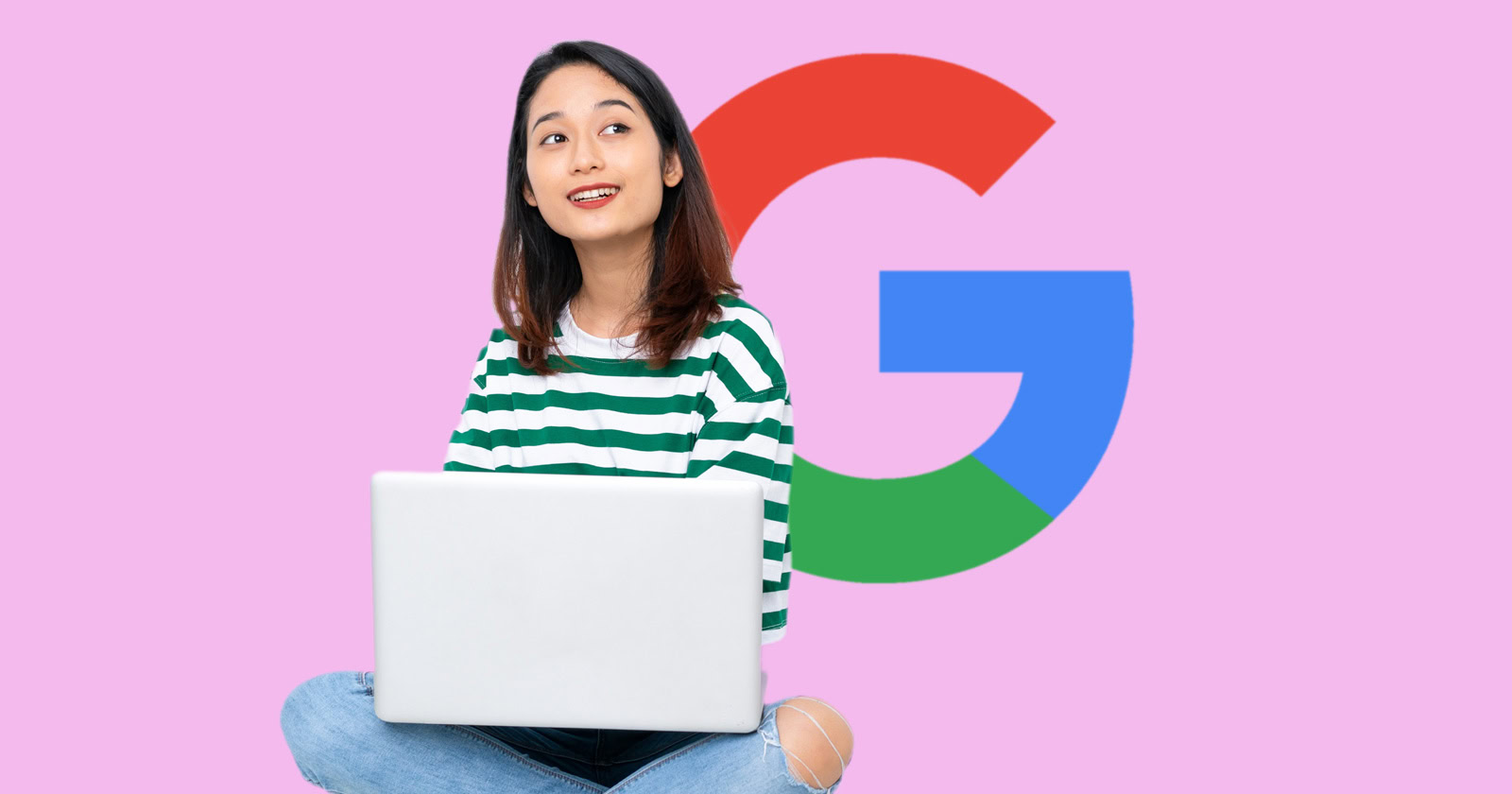
Google Search Central published new documentation on Google Trends, explaining how to use it for search marketing. This guide serves as an easy to understand introduction for newcomers and a helpful refresher for experienced search marketers and publishers.
The new guide has six sections:
- About Google Trends
- Tutorial on monitoring trends
- How to do keyword research with the tool
- How to prioritize content with Trends data
- How to use Google Trends for competitor research
- How to use Google Trends for analyzing brand awareness and sentiment
The section about monitoring trends advises there are two kinds of rising trends, general and specific trends, which can be useful for developing content to publish on a site.
Using the Explore tool, you can leave the search box empty and view the current rising trends worldwide or use a drop down menu to focus on trends in a specific country. Users can further filter rising trends by time periods, categories and the type of search. The results show rising trends by topic and by keywords.
To search for specific trends users just need to enter the specific queries and then filter them by country, time, categories and type of search.
The section called Content Calendar describes how to use Google Trends to understand which content topics to prioritize.
Google explains:
“Google Trends can be helpful not only to get ideas on what to write, but also to prioritize when to publish it. To help you better prioritize which topics to focus on, try to find seasonal trends in the data. With that information, you can plan ahead to have high quality content available on your site a little before people are searching for it, so that when they do, your content is ready for them.”
Read the new Google Trends documentation:
Get started with Google Trends
Featured Image by Shutterstock/Luis Molinero
You must be logged in to post a comment Login