SEO
Is This Dataset Used For Google’s AI Search?
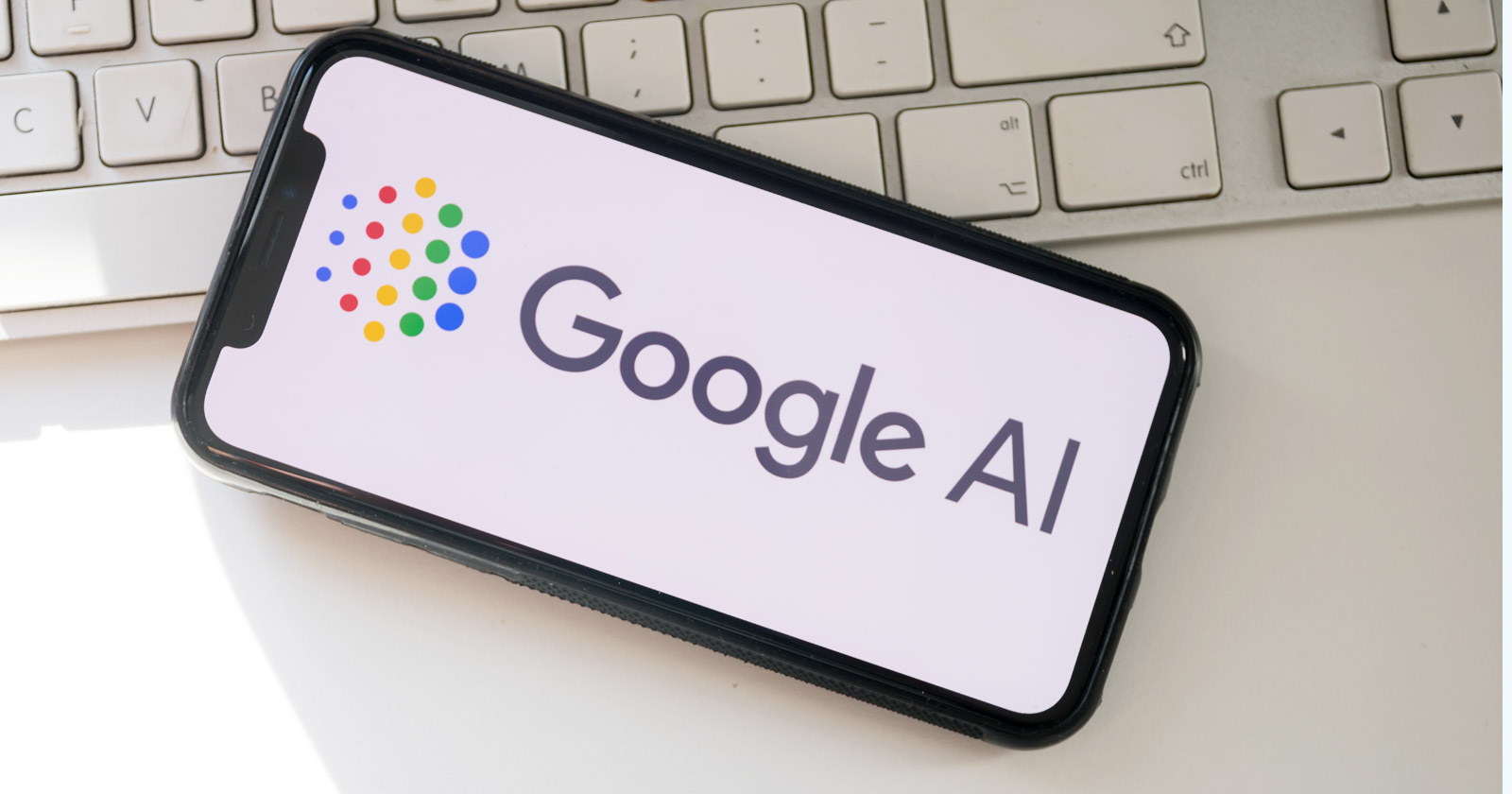
Google published a research paper on a new kind of dataset for training a language model to retrieve sentences that exactly answer a question within an open-ended dialogue.
We don’t know if Google is using this dataset. But researchers claim it outperforms models trained on other datasets.
Many research papers, like the one published for LaMDA don’t mention specific contexts of how it could be used.
For example, the LaMDA research paper (PDF) vaguely concludes:
“LaMDA is a step closer to practical and safe open-ended dialog systems, which can in turn unlock a wide range of useful applications.”
This research paper states the problem they are solving is how to create a dataset for training a machine for an open-ended dialogue by selecting a sentence from a webpage.
Why This Dataset is Important
What makes this research paper of interest is that the researchers conclude that it could be used for factually grounding generative AI output, like what is seen in Google’s new Search Generative Experience.
Given that the research paper was presented at an Information Retrieval conference (Proceedings of the 45th International ACM SIGIR Conference on Research and Development), it’s fairly safe to guess that this algorithm is related to information retrieval, which means search.
One last thing to note is that the research on this new kind of dataset was presented last year in 2022 but it has apparently gone unnoticed… Until now.
What Google Set Out to Achieve With the New Dataset
The researchers explain what they are focused on:
“In this paper we focus on open-ended dialogues: two parties converse in turns on any number of topics with no restrictions to the topic shifts and type of discussion on each topic.
In addition, the dialogue is not grounded to a specific document, in contrast to the setting used in some previous work…
The task we address is retrieving sentences from some document corpus that contain information useful for generating (either automatically or by humans) the next turn in the dialogue.
We note that the dialogue turns can be questions, queries, arguments, statements, etc.”
A New Kind of Dataset For Language Model Training
The problem the researchers are solving is how to retrieve a sentence from a webpage as the answer to an open-ended question, a type of question that needs more than a yes or no answer.
The research paper explains that what is missing to make that ability happen in a machine is an appropriate conversational dataset.
They explain that existing datasets are used for two reasons:
- To evaluate dialogue responses by a generative AI but not for use in training it to actually retrieve the relevant information for that response.
- Datasets for use by a search engine or question answering, focused on a single passage of a question and answer.
They explain the shortcomings of existing datasets:
“…in most of these datasets, the returned search results are not viewed as part of the dialogue.
…in both conversational passage retrieval and conversational QA datasets, there is a user asking questions or queries that reflect explicit intents with information needs, as opposed to natural dialogues where intents may be only implicitly represented, e.g., in affirmative statements.
To sum, existing conversational datasets do not combine natural human-human conversations with relevance annotations for sentences retrieved from a large document corpus.
We therefore constructed such a dataset…”
How the New Dataset Was Created
The researchers created a dataset that can be used to train an algorithm that can retrieve a sentence that is the correct response in an open-ended dialogue.
The dataset consists of Reddit conversations that were matched to answers from Wikipedia, plus human annotations (relevance ratings), of those question and answer pairs.
Reddit data was downloaded from Pushshift.io, an archive of Reddit conversations (Pushshift FAQ).
The research paper explains:
“To address a broader scope of this task where any type of dialogue can be used, we constructed a dataset that includes openended dialogues from Reddit, candidate sentences from Wikipedia for each dialogue and human annotations for the sentences.
The dataset includes 846 dialogues created from Reddit threads.
For each dialogue, 50 sentences were retrieved from Wikipedia using an unsupervised initial retrieval method.
These sentences were judged by crowd workers for relevance, that is, whether they contained information useful for generating the next turn in the dialogue.”
The dataset they created is available at GitHub.
Example of a dialogue question:
“Which came first, the chicken or the egg?”
An example of an irrelevant answer:
“Domesticated chickens have been around for about 10,000 years. Eggs have been around for hundreds of millions of years.”
An example of a correct webpage sentence that can be used for answer is:
“Put more simply by Neil deGrasse Tyson:
‘Which came first: the chicken or the egg? The egg-laid by a bird that was not a chicken.’”
Retrieval Methodology
For the retrieval part they cite prior research in language models and other methods and settle on weak supervision approach.
They explain:
“Fine-tuning of retrieval models requires relevance labels for training examples in a target task.
These are sometimes scarce or unavailable.
One approach to circumvent this is to automatically generate labels and train a weakly supervised model on these annotations.
…We follow the weak supervision paradigm in our model training, with a novel weak Reddit annotator for retrieval in a dialogue context.”
Is the Dataset Successful?
Google and other organizations publish many research papers that demonstrate varying levels of success.
Some research concludes with limited success, moving the state of the art by just a little if at all.
The research papers that are of interest (to me) are the ones that are clearly successful and outperform the current state of the art.
That’s the case with the development of this dataset for training a language model to retrieve sentences that accurately serve as a turn in an open-ended dialogue.
They state how a BERT model trained with this dataset becomes even more powerful.
They write:
“Indeed, while RANKBERTMS outperforms all non-fine-tuned models, the RANKBERTMS→R model, which was further fine-tuned using our weakly supervised training set, improves the performance.
This method attains the highest performance with all performance gains over other methods being statistically significant.
This finding also demonstrates the effectiveness of our weak annotator and weakly supervised training set, showing that performance can be improved without manual annotation for training.”
Elsewhere the researchers report:
“We show that a neural ranker which was fined-tuned using our weakly supervised training set outperforms all other tested models, including a neural ranker fine-tuned on the MS Marco passage retrieval dataset.”
They also write that as successful as this approach is, they are interested in furthering the state of the art even more than they already have.
The research paper concludes:
“In future work, we would like to devise BERT-based retrieval models that are trained based on weak supervision alone, using a pre-trained BERT, without the need for large annotated training sets like MS Marco.
We would also like to ground generative language models with our retrieval models and study the conversations that emerge from such grounding.”
Could This Approach Be In Use?
Google rarely confirms when specific research is used. There are some cases, such as with BERT, where Google confirms they are using it.
But in general the standard response is that just because Google publishes a research paper or a patent doesn’t mean that they are using it in their search algorithm.
That said, the research paper, which dates from mid-2022, indicated that a future direction was to study how generative language models (which is like Bard and Google’s Search Generative Experience) can be grounded with it.
An AI generative chat experience can result in the AI output making things up, what is technically known as hallucinating.
Grounding means anchoring the AI chat output with facts, typically from online sources, to help prevent hallucinations.
Bing uses a system called the Bing Orchestrator that checks webpages to ground the GPT output in facts.
Grounding the AI output helps keep it grounded to facts, which is something that this dataset may be capable of doing, in addition to selecting sentences from webpages as part of an answer.
Read the Research Paper:
Abstract Webpage: A Dataset for Sentence Retrieval for Open-Ended Dialogues
Actual Research Paper: A Dataset for Sentence Retrieval for Open-Ended Dialogues
Featured image by Shutterstock/Camilo Concha