MARKETING
3 Questions About AI in Content: What? So What? Now What?
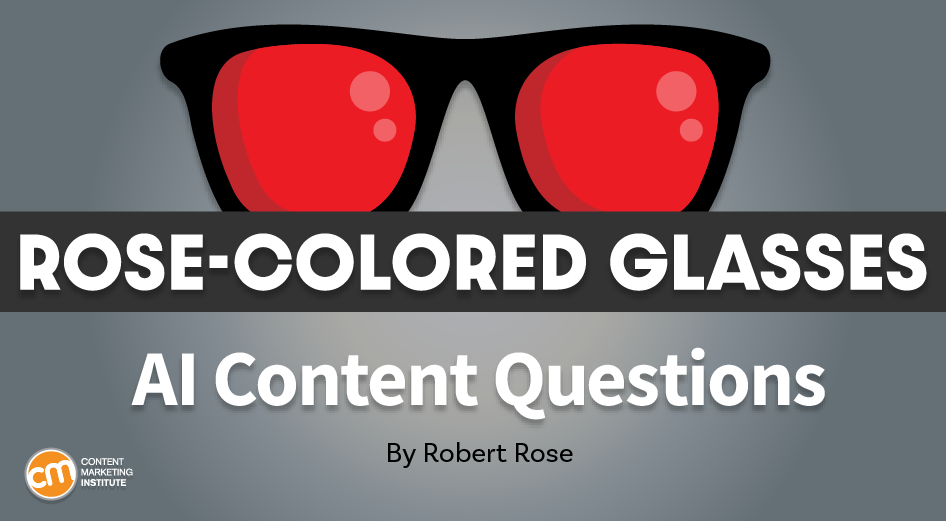
In the United States, Thanksgiving will give us the needed break to take a collective breath.
I don’t know about you, but getting my bearings around the disruptions of generative AI presents an extreme challenge. Innovations come so quickly that once we think we have our arms around it, something new appears.
Almost one year into seeing what generative AI can do for content creation and marketing strategies, OpenAI has introduced custom GPTs for those who pay for access.
You can build custom ChatGPT applications to use the tool’s newest capabilities to do things specifically valuable to you. For example, your company could upload 10 years of blog articles and instruct the custom GPT to use the knowledge gained from the content to formulate answers to questions on the blogs’ topics. In theory, you get the depth and breadth of ChatGPT’s large language learning model focused on your knowledge base and able to take specific actions, such as sending an email or automating a task.
Impressive. But sheesh. What does that do to your plans to integrate tools into your marketing workflow? It seems like one of a hundred things that you’re supposed to pay attention to right now.
Time to reflect
If your time frees up this week either because of the holiday or because the Americans are on holiday, take a moment and reflect on these disruptions to your current marketing and content efforts.
A little more than 20 years ago, a nursing professor at Swansea University published a helpful framework for self-reflection and communication. His exercise has helped me in times of disruption, and perhaps it can be for you as well.
Answer a few questions that fall into three stages – what, so what, and now what?
- What? Describe what has happened simply and objectively – without judgment or interpretation. Some helpful prompts: What happened? What did you observe? What events occurred? What is the current situation?
- So what? Answer questions about what you know now that you didn’t know. You can introduce emotions. Some helpful prompts: What did you learn? What difference have the events made? Answer as yourself or within the context of your team or company.
If it’s just you, potential questions could be: Did what happened clarify an interest? Did you hear or feel anything that surprised you? How is your experience different than what you expected? What do these events mean to you?
If you answer on behalf of a team or group, you can ask the self-questions along with these prompts: What do these events suggest to you about this group? How might the group work better or worse with these events? How were decisions made or not made based on these events?
- Now what? Reflect on your future actions based on the first two steps. These broader implications react to what happened. Questions center on defining and looking at the root cause: What would contribute to a successful response? What would be in the way of successfully navigating through this? What learning has now occurred, and how can I/we apply this learning?
Ask your team to do this same exercise. When you meet back up, create a workshop or team gathering where you discuss the answers and determine where opportunities may exist.
Real reflections aren’t hot takes
If you find yourself thinking that process is basic, well, you’re right. These three questions – and the provocations that come from them – mirror a progression you’ve all tried to work through a problem. However, you don’t often do it for big disruptions in the moment. It’s just too easy to jump to the third step, “now what,” and confuse it with “what’s next.” You get overwhelmed by all the actions you can take.
You can see this challenge happening with the disruption of generative AI.
Check out this article that reflects on the disruption of generative AI in the video game industry. To make the case, it leverages Bain & Company research that “more than half of video game development process will be supported by generative AI within the next five to 10 years.” It uses “what happened” to make a case for “what’s next.” The author didn’t even bother to ask “so what” to reach the conclusion: “Microsoft wants AI to solve problems that game makers say they won’t actually have.”
If you reflect on what the Bain research actually said, you can see it’s almost the opposite of the Microsoft conclusion. The research plainly says few executives believe AI will reduce development costs. They say AI will not significantly impact talent and “do not believe it will replace the creative spark necessary for game development.”
By misinterpreting what happened and not asking, “So what,” the author jumped to predicting what’s next, which is almost useless to make any productive change to address what’s really happening.
This is why working through this process is helpful.
Now, to be clear, hot takes are fun. I’m not suggesting you do away with predictions or the occasional response. Hot takes are a great way to start the conversation, not to finish them.
Take the time – and the process – to work it out. It’s not perfect. It’s also not meant to be a fail-safe way to predict the future. The three-question stages are meant to help you balance facts and feelings to make more productive and satisfying responses to the disruptions you face.
The process is meant to change your future, not by helping you see it more clearly but by helping you clearly see how you change it.
It’s your story. Have a wonderful, reflective Thanksgiving, and tell it well.
Cover image by Joseph Kalinowski/Content Marketing Institute
MARKETING
YouTube Ad Specs, Sizes, and Examples [2024 Update]
![YouTube Ad Specs, Sizes, and Examples [2024 Update] YouTube Ad Specs, Sizes, and Examples](https://articles.entireweb.com/wp-content/uploads/2024/06/YouTube-Ad-Specs-Sizes-and-Examples.jpg)
Introduction
With billions of users each month, YouTube is the world’s second largest search engine and top website for video content. This makes it a great place for advertising. To succeed, advertisers need to follow the correct YouTube ad specifications. These rules help your ad reach more viewers, increasing the chance of gaining new customers and boosting brand awareness.
Types of YouTube Ads
Video Ads
- Description: These play before, during, or after a YouTube video on computers or mobile devices.
- Types:
- In-stream ads: Can be skippable or non-skippable.
- Bumper ads: Non-skippable, short ads that play before, during, or after a video.
Display Ads
- Description: These appear in different spots on YouTube and usually use text or static images.
- Note: YouTube does not support display image ads directly on its app, but these can be targeted to YouTube.com through Google Display Network (GDN).
Companion Banners
- Description: Appears to the right of the YouTube player on desktop.
- Requirement: Must be purchased alongside In-stream ads, Bumper ads, or In-feed ads.
In-feed Ads
- Description: Resemble videos with images, headlines, and text. They link to a public or unlisted YouTube video.
Outstream Ads
- Description: Mobile-only video ads that play outside of YouTube, on websites and apps within the Google video partner network.
Masthead Ads
- Description: Premium, high-visibility banner ads displayed at the top of the YouTube homepage for both desktop and mobile users.
YouTube Ad Specs by Type
Skippable In-stream Video Ads
- Placement: Before, during, or after a YouTube video.
- Resolution:
- Horizontal: 1920 x 1080px
- Vertical: 1080 x 1920px
- Square: 1080 x 1080px
- Aspect Ratio:
- Horizontal: 16:9
- Vertical: 9:16
- Square: 1:1
- Length:
- Awareness: 15-20 seconds
- Consideration: 2-3 minutes
- Action: 15-20 seconds
Non-skippable In-stream Video Ads
- Description: Must be watched completely before the main video.
- Length: 15 seconds (or 20 seconds in certain markets).
- Resolution:
- Horizontal: 1920 x 1080px
- Vertical: 1080 x 1920px
- Square: 1080 x 1080px
- Aspect Ratio:
- Horizontal: 16:9
- Vertical: 9:16
- Square: 1:1
Bumper Ads
- Length: Maximum 6 seconds.
- File Format: MP4, Quicktime, AVI, ASF, Windows Media, or MPEG.
- Resolution:
- Horizontal: 640 x 360px
- Vertical: 480 x 360px
In-feed Ads
- Description: Show alongside YouTube content, like search results or the Home feed.
- Resolution:
- Horizontal: 1920 x 1080px
- Vertical: 1080 x 1920px
- Square: 1080 x 1080px
- Aspect Ratio:
- Horizontal: 16:9
- Square: 1:1
- Length:
- Awareness: 15-20 seconds
- Consideration: 2-3 minutes
- Headline/Description:
- Headline: Up to 2 lines, 40 characters per line
- Description: Up to 2 lines, 35 characters per line
Display Ads
- Description: Static images or animated media that appear on YouTube next to video suggestions, in search results, or on the homepage.
- Image Size: 300×60 pixels.
- File Type: GIF, JPG, PNG.
- File Size: Max 150KB.
- Max Animation Length: 30 seconds.
Outstream Ads
- Description: Mobile-only video ads that appear on websites and apps within the Google video partner network, not on YouTube itself.
- Logo Specs:
- Square: 1:1 (200 x 200px).
- File Type: JPG, GIF, PNG.
- Max Size: 200KB.
Masthead Ads
- Description: High-visibility ads at the top of the YouTube homepage.
- Resolution: 1920 x 1080 or higher.
- File Type: JPG or PNG (without transparency).
Conclusion
YouTube offers a variety of ad formats to reach audiences effectively in 2024. Whether you want to build brand awareness, drive conversions, or target specific demographics, YouTube provides a dynamic platform for your advertising needs. Always follow Google’s advertising policies and the technical ad specs to ensure your ads perform their best. Ready to start using YouTube ads? Contact us today to get started!
MARKETING
Why We Are Always ‘Clicking to Buy’, According to Psychologists
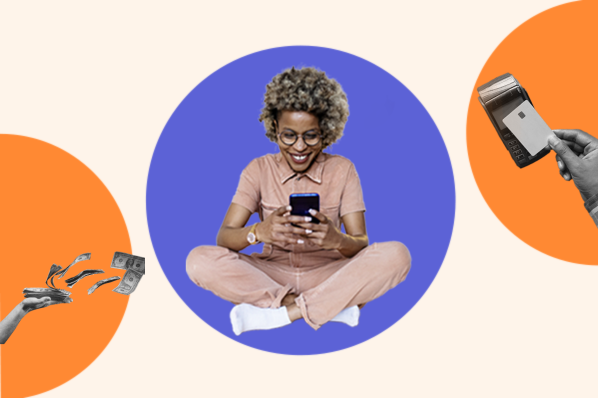
Amazon pillows.
MARKETING
A deeper dive into data, personalization and Copilots
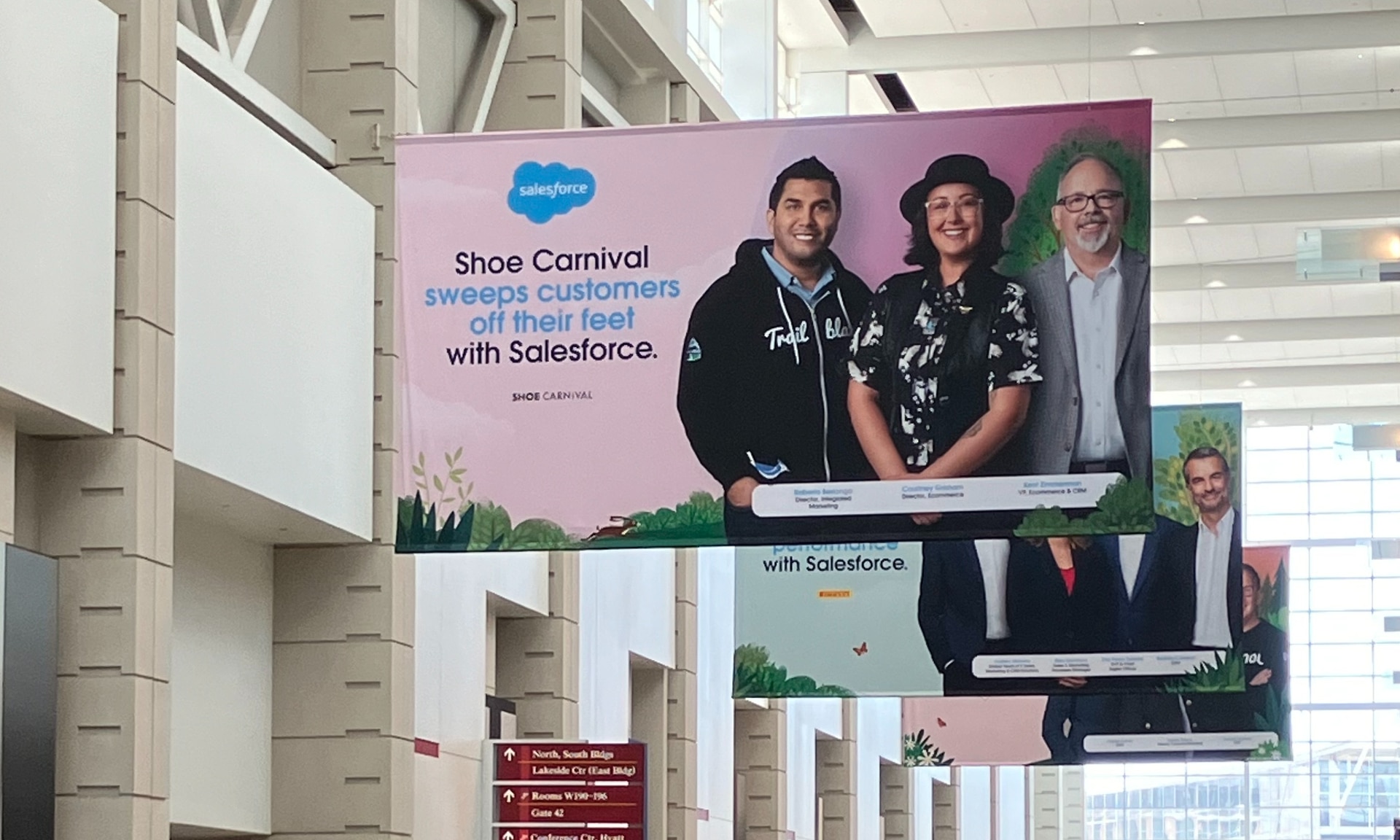
Salesforce launched a collection of new, generative AI-related products at Connections in Chicago this week. They included new Einstein Copilots for marketers and merchants and Einstein Personalization.
To better understand, not only the potential impact of the new products, but the evolving Salesforce architecture, we sat down with Bobby Jania, CMO, Marketing Cloud.
Dig deeper: Salesforce piles on the Einstein Copilots
Salesforce’s evolving architecture
It’s hard to deny that Salesforce likes coming up with new names for platforms and products (what happened to Customer 360?) and this can sometimes make the observer wonder if something is brand new, or old but with a brand new name. In particular, what exactly is Einstein 1 and how is it related to Salesforce Data Cloud?
“Data Cloud is built on the Einstein 1 platform,” Jania explained. “The Einstein 1 platform is our entire Salesforce platform and that includes products like Sales Cloud, Service Cloud — that it includes the original idea of Salesforce not just being in the cloud, but being multi-tenancy.”
Data Cloud — not an acquisition, of course — was built natively on that platform. It was the first product built on Hyperforce, Salesforce’s new cloud infrastructure architecture. “Since Data Cloud was on what we now call the Einstein 1 platform from Day One, it has always natively connected to, and been able to read anything in Sales Cloud, Service Cloud [and so on]. On top of that, we can now bring in, not only structured but unstructured data.”
That’s a significant progression from the position, several years ago, when Salesforce had stitched together a platform around various acquisitions (ExactTarget, for example) that didn’t necessarily talk to each other.
“At times, what we would do is have a kind of behind-the-scenes flow where data from one product could be moved into another product,” said Jania, “but in many of those cases the data would then be in both, whereas now the data is in Data Cloud. Tableau will run natively off Data Cloud; Commerce Cloud, Service Cloud, Marketing Cloud — they’re all going to the same operational customer profile.” They’re not copying the data from Data Cloud, Jania confirmed.
Another thing to know is tit’s possible for Salesforce customers to import their own datasets into Data Cloud. “We wanted to create a federated data model,” said Jania. “If you’re using Snowflake, for example, we more or less virtually sit on your data lake. The value we add is that we will look at all your data and help you form these operational customer profiles.”
Let’s learn more about Einstein Copilot
“Copilot means that I have an assistant with me in the tool where I need to be working that contextually knows what I am trying to do and helps me at every step of the process,” Jania said.
For marketers, this might begin with a campaign brief developed with Copilot’s assistance, the identification of an audience based on the brief, and then the development of email or other content. “What’s really cool is the idea of Einstein Studio where our customers will create actions [for Copilot] that we hadn’t even thought about.”
Here’s a key insight (back to nomenclature). We reported on Copilot for markets, Copilot for merchants, Copilot for shoppers. It turns out, however, that there is just one Copilot, Einstein Copilot, and these are use cases. “There’s just one Copilot, we just add these for a little clarity; we’re going to talk about marketing use cases, about shoppers’ use cases. These are actions for the marketing use cases we built out of the box; you can build your own.”
It’s surely going to take a little time for marketers to learn to work easily with Copilot. “There’s always time for adoption,” Jania agreed. “What is directly connected with this is, this is my ninth Connections and this one has the most hands-on training that I’ve seen since 2014 — and a lot of that is getting people using Data Cloud, using these tools rather than just being given a demo.”
What’s new about Einstein Personalization
Salesforce Einstein has been around since 2016 and many of the use cases seem to have involved personalization in various forms. What’s new?
“Einstein Personalization is a real-time decision engine and it’s going to choose next-best-action, next-best-offer. What is new is that it’s a service now that runs natively on top of Data Cloud.” A lot of real-time decision engines need their own set of data that might actually be a subset of data. “Einstein Personalization is going to look holistically at a customer and recommend a next-best-action that could be natively surfaced in Service Cloud, Sales Cloud or Marketing Cloud.”
Finally, trust
One feature of the presentations at Connections was the reassurance that, although public LLMs like ChatGPT could be selected for application to customer data, none of that data would be retained by the LLMs. Is this just a matter of written agreements? No, not just that, said Jania.
“In the Einstein Trust Layer, all of the data, when it connects to an LLM, runs through our gateway. If there was a prompt that had personally identifiable information — a credit card number, an email address — at a mimum, all that is stripped out. The LLMs do not store the output; we store the output for auditing back in Salesforce. Any output that comes back through our gateway is logged in our system; it runs through a toxicity model; and only at the end do we put PII data back into the answer. There are real pieces beyond a handshake that this data is safe.”
-
SEARCHENGINES5 days ago
Billions Of Google goo.gl URLs To 404 In The Future
-
SEO7 days ago
26 Common SEO Myths, Debunked
-
SEARCHENGINES4 days ago
Daily Search Forum Recap: July 22, 2024
-
SEARCHENGINES6 days ago
Google Core Update Coming, Ranking Volatility, Bye Search Notes, AI Overviews, Ads & More
-
SEO5 days ago
11 Copyscape Alternatives To Check Plagiarism
-
SEO6 days ago
Google Warns Of Last Chance To Export Notes Search Data
-
AFFILIATE MARKETING5 days ago
The Top 5 AI Tools That Can Revolutionize Your Workflow and Boost Productivity
-
SEARCHENGINES3 days ago
Daily Search Forum Recap: July 23, 2024