MARKETING
Balancing Creativity With Caution When Using AI to Create Content
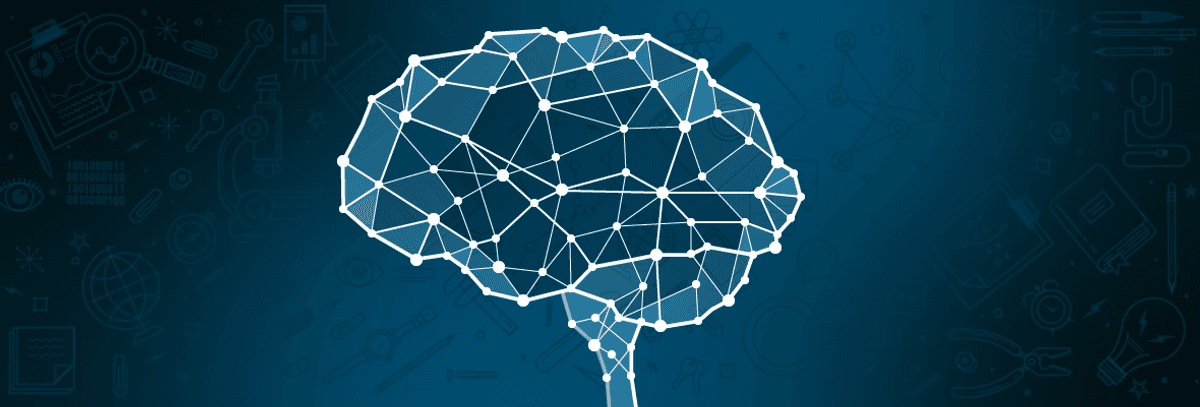
The author’s views are entirely their own (excluding the unlikely event of hypnosis) and may not always reflect the views of Moz.
I’m the kind of writer who hates to write but loves having written. Leading a marketing consultancy, where 99% of my work involves writing, only amplifies this conundrum.
If this statement resonates with you, you’ll understand the allure of generative artificial intelligence (AI) tools like ChatGPT for marketers, whether they are client-side or agency-side. These technologies have the potential to simplify an arduous writing process, helping writers skip the torture of the blank page and fast-forward to the gratification of a published article. It’s a junk food promise, satisfaction without effort.
I first dipped my toes into the world of generative AI in November 2022 and was initially captivated by the quick wins ChatGPT seemed to offer. Here was a tool that could churn out paragraph after paragraph of seemingly well-crafted copy at lightning speed. It was easy to envision how this might revolutionize my work and allow me to become a prose powerhouse. But the more I played with a number of large language models (LLM)/generative AI tools, the more I became aware of the risks. Especially as someone who works with clients and has a duty to provide them with well-researched, well-articulated, and credible advice.
This article is my attempt to provide guardrails and advice for marketers who are rightfully skeptical of the AI revolution.
Some basic rules everyone should be following
Whether you’re using generative AI tools to create content for yourself, your employer, or a client, there are some basic tenants to follow.
Safeguard proprietary information
Never, ever input proprietary or sensitive data into the AI model, including company data and IP that is not freely available in the public domain. This also includes client-specific information like private datasets, business strategies, internal reports, customer information, and other confidential materials. Several companies, including Amazon, have restricted employees from using tools like GitHub Co-Pilot and ChatGPT due to fears AI could lead to a potential leak of confidential data due to the potential for inputs to be stored and used as training data.
I’d go one step further and always replace the subject’s name with a pseudonym. If you need to use real data for context, replace all personally identifiable information (PII) and sensitive business information with anonymized or fictional substitutes.
Consent is critical
Before using any client data, ensure you have the necessary permissions. Sharing data with an AI model can be considered data sharing and can violate confidentiality agreements and data protection laws, so you do need to tread carefully and should not assume you have consent to share information. Get legal advice if you need it.
Most clients and businesses will be aware people use generative AI now as part of their work. If you can be transparent about how you use AI tools and how you approach consent and data sharing, you can go a long way toward demonstrating that you understand and have mitigated any risks.
Rigorously review outputs
Always review the generated content for accidental inclusion of sensitive data. AI models might infer from the data provided and unintentionally generate 3rd party sensitive content based on their extant dataset.
You should also thoroughly review outputs to ensure they don’t unintentionally reference proprietary or sensitive client/business information.
Avoid intellectual property infringements
When using Midjourney to create imagery or ChatGPT to create copy, avoid using “in the style of X” prompts which direct the model to imitate an individual’s work. This could violate copyright laws and is frankly extremely lazy, even when you’re referencing historical artists whose work is no longer protected by copyright. A recent example of the backlash of using generative AI has been discussed by artists on imitating their style. However, you can absolutely leverage client brand tone of voice directions to guide copy outputs.
In addition to not replicating the style of specific authors or artists, respect all intellectual property rights. This includes text, images, designs, or any other content that may be subject to copyright.
Don’t mindlessly trust outputs
Full Fact CEO Will Moy recently told the UK Online Harms and Disinformation inquiry on misinformation and trusted voices that “the ability to flood public debate with automatically generated text, images, video and datasets that provide apparently credible evidence for almost any proposition is a game changer in terms of what trustworthy public debate looks like, and the ease of kicking up so much dust that no one can see what is going on. That is a very well-established disinformation tactic.”
As members of a democratic society striving for transparent public discourse, we must recognize our role in counteracting the ease with which AI can be harnessed to disseminate disinformation that could materially damage our way of life. The responsibility of fostering an informed society lies not only with fact-checkers and official authorities but also with us as content creators, curators, and consumers of information.
There are two significant issues with large language models such as ChatGPT. The first is hallucination – which refers to the generation of outputs that are not based on input data or that significantly deviate from factual information present in the input. Secondly, the models are only as good as the data they are trained on – if the training data contains misinformation, the model can learn and replicate it.
Sadly there isn’t a technological solution to verifying if outputs are factually correct. Automated fact-checking has been around for some time now, and while it is making significant strides in verifying a select range of basic factual assertions with available authoritative data, it still has its limitations. As of yet, there is no tool that can fully automate the checking of outputs from another tool with 100% accuracy.
The challenge lies in context – the complexity and contextual sensitivity required for comprehensive fact-checking is still beyond the scope of fully automated systems. Subtle changes in a claim’s wording, timing, or context can make it more or less reasonable. Even a perfectly accurate statistic can misinform when the correlation is mistaken for causation (for example, by year, the number of people drowned in pools correlates with the number of films featuring Nicholas Cage).
So how can we use our human powers of reasoning and decision-making to ensure that facts and figures are verified and used in the correct context?
Verify sources, figures, and facts with multiple third-party trusted sources
Refrain from taking the information presented at face value. Make a habit of cross-checking any facts, figures, or sources presented in AI-generated content with multiple trusted sources. This could include reputable news outlets, government databases, or academic journals.
Don’t trust links generated by Generative AI tools; find your own
While AI models like ChatGPT may suggest links related to the topic, verifying these before using them is crucial. Ensure that the links are active, the domains are reputable, and the specific pages are relevant and reliable. In many cases, it’s best to find your own sources from established, trustworthy sites that you’re familiar with.
Use fact-checking websites
Websites like Full Fact, Snopes, or FactCheck.org can be invaluable when verifying facts. They provide detailed analyses of claims, often referencing their sources, and can help you separate fact from fiction.
Get up-to-date data
The accuracy of data is often time-sensitive. What was true a year ago may not hold today. When using data in your content, always check the date it was published or collected. Try to use the most recent and relevant data available, and remember ChatGPT’s training data has the cut off-date of September 2021. So, if you ask where the Queen of England currently resides, it will tell you Buckingham Palace.
Even using the most up-to-date model, such as ChatGPT 4, does not guarantee data or accuracy will be improved. While ChatGPT 4 is better at synthesizing information from multiple sources, OpenAI still admits its hallucination rate is similar to previous models.
Still unsure? How to deal with uncertain information
When encountering uncertain or unverified information, it’s essential to exercise caution and transparency.
If you come across dubious or unsupported facts, consider excluding them to maintain credibility. However, if the information is key to your topic but its validity is unclear, it’s important to express this uncertainty to your audience, presenting any alternate perspectives if available. If possible, consult subject matter experts in the relevant field to gain further insight and possibly resolve the ambiguity. (Also, remember the expertise element of E-E-A-T – it’s in your interest to cite expert opinions.)
Speaking of expert opinions, it’s important to verify that the expert you’re quoting is credible. Think like Google here – is the individual mentioned on other high-quality websites? Do they have relevant qualifications, are they cited in professional journals or publications? You are responsible for fact-checking the status of the fact checker.
How should we be using AI then?
So far, I’ve explained how you can reduce risk when using AI tools and how to prevent the dissemination of misinformation. After all this, you might feel like tools like ChatGPT sound more trouble than they’re worth. After all, considering the due diligence required, you might question if it’s easier to simply create the content unaided. There is an element of truth to this perspective.
However, as a marketing advisor and consultant, instead of treating AI as a tool to create the raw material, I’m using it to improve my creativity and efficiency in three ways. You’ll note that none of these involve asking the technology to come up with something from scratch.
Acceleration
During the initial stages of the creative process, my first batch of ideas often lacks originality or spark. This is something I hate about writing; it can take me a long time to get into the flow of it.
A wise creative writing tutor once told me that the first 30 minutes of writing is about getting the crap ideas out of your head to make way for the good ones. That’s why it feels so painful. Since AI-generated content like ChatGPT is based on existing material, using a deterministic engine to return the most probable result, I use it to quickly generate these “bad” ideas, effectively taking the reductive concepts off the table. If ChatGPT can come up with it, it’s probably not a novel or interesting idea.
Reflection
Another way I use AI to enhance my creativity is by reflecting on my own creative output. For example, after writing an article or developing a piece of work, I often use AI to summarise the key points or arguments I’ve made, which I can then review for completeness. This helps me ensure that I haven’t missed anything important and that my messaging is consistent and coherent. Additionally, AI can help me identify gaps in my arguments or inconsistencies in my messaging. This process is akin to “rubber-ducking” my copy at scale. Interestingly I still prefer to pass things by a human editor for a full review once I’m happy.
Variation
I also use AI to generate variations of my original content, giving me different perspectives on presenting my ideas. By exploring alternative phrasings, sentence structures, or even entire paragraph arrangements, I can identify more engaging and impactful ways to convey my message. I don’t typically copy and paste the variants word for word, but cherry-pick the best bits from the outputs. Sometimes that’s just a word.