SEO
Bard Vs ChatGPT Vs Claude
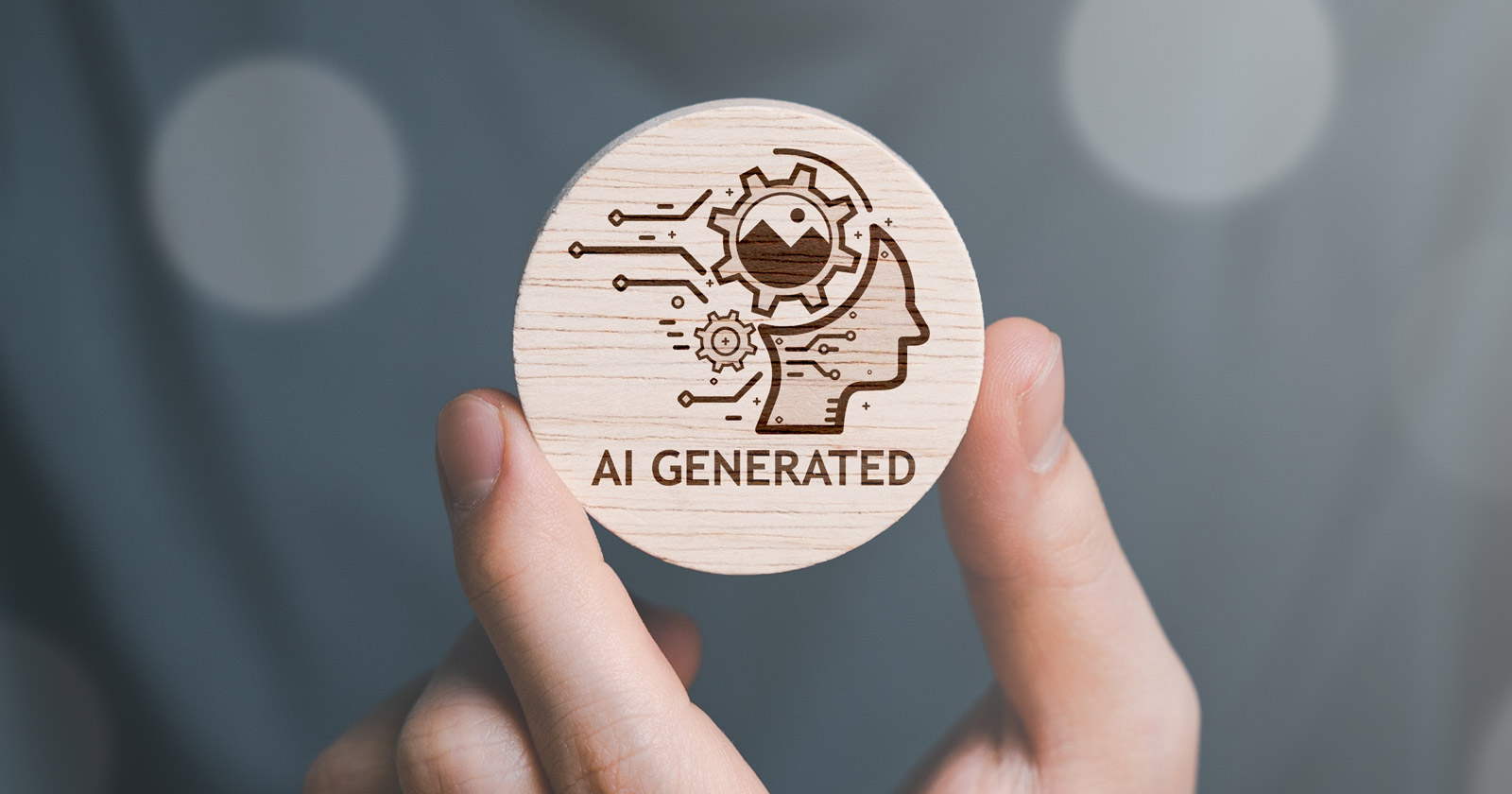
Researchers tested the idea that an AI model may have an advantage in self-detecting its own content because the detection was leveraging the same training and datasets. What they didn’t expect to find was that out of the three AI models they tested, the content generated by one of them was so undetectable that even the AI that generated it couldn’t detect it.
The study was conducted by researchers from the Department of Computer Science, Lyle School of Engineering at Southern Methodist University.
AI Content Detection
Many AI detectors are trained to look for the telltale signals of AI generated content. These signals are called “artifacts” which are generated because of the underlying transformer technology. But other artifacts are unique to each foundation model (the Large Language Model the AI is based on).
These artifacts are unique to each AI and they arise from the distinctive training data and fine tuning that is always different from one AI model to the next.
The researchers discovered evidence that it’s this uniqueness that enables an AI to have a greater success in self-identifying its own content, significantly better than trying to identify content generated by a different AI.
Bard has a better chance of identifying Bard-generated content and ChatGPT has a higher success rate identifying ChatGPT-generated content, but…
The researchers discovered that this wasn’t true for content that was generated by Claude. Claude had difficulty detecting content that it generated. The researchers shared an idea of why Claude was unable to detect its own content and this article discusses that further on.
This is the idea behind the research tests:
“Since every model can be trained differently, creating one detector tool to detect the artifacts created by all possible generative AI tools is hard to achieve.
Here, we develop a different approach called self-detection, where we use the generative model itself to detect its own artifacts to distinguish its own generated text from human written text.
This would have the advantage that we do not need to learn to detect all generative AI models, but we only need access to a generative AI model for detection.
This is a big advantage in a world where new models are continuously developed and trained.”
Methodology
The researchers tested three AI models:
- ChatGPT-3.5 by OpenAI
- Bard by Google
- Claude by Anthropic
All models used were the September 2023 versions.
A dataset of fifty different topics was created. Each AI model was given the exact same prompts to create essays of about 250 words for each of the fifty topics which generated fifty essays for each of the three AI models.
Each AI model was then identically prompted to paraphrase their own content and generate an additional essay that was a rewrite of each original essay.
They also collected fifty human generated essays on each of the fifty topics. All of the human generated essays were selected from the BBC.
The researchers then used zero-shot prompting to self-detect the AI generated content.
Zero-shot prompting is a type of prompting that relies on the ability of AI models to complete tasks for which they haven’t specifically trained to do.
The researchers further explained their methodology:
“We created a new instance of each AI system initiated and posed with a specific query: ‘If the following text matches its writing pattern and choice of words.’ The procedure is
repeated for the original, paraphrased, and human essays, and the results are recorded.We also added the result of the AI detection tool ZeroGPT. We do not use this result to compare performance but as a baseline to show how challenging the detection task is.”
They also noted that a 50% accuracy rate is equal to guessing which can be regarded as essentially a level of accuracy that is a failure.
Results: Self-Detection
It must be noted that the researchers acknowledged that their sample rate was low and said that they weren’t making claims that the results are definitive.
Below is a graph showing the success rates of AI self-detection of the first batch of essays. The red values represent the AI self-detection and the blue represents how well the AI detection tool ZeroGPT performed.
Results Of AI Self-Detection Of Own Text Content
Bard did fairly well at detecting its own content and ChatGPT also performed similarly well at detecting its own content.
ZeroGPT, the AI detection tool detected the Bard content very well and performed slightly less better in detecting ChatGPT content.
ZeroGPT essentially failed to detect the Claude-generated content, performing worse than the 50% threshold.
Claude was the outlier of the group because it was unable to to self-detect its own content, performing significantly worse than Bard and ChatGPT.
The researchers hypothesized that it may be that Claude’s output contains less detectable artifacts, explaining why both Claude and ZeroGPT were unable to detect the Claude essays as AI-generated.
So, although Claude was unable to reliably self-detect its own content, that turned out to be a sign that the output from Claude was of a higher quality in terms of outputting less AI artifacts.
ZeroGPT performed better at detecting Bard-generated content than it did in detecting ChatGPT and Claude content. The researchers hypothesized that it could be that Bard generates more detectable artifacts, making Bard easier to detect.
So in terms of self-detecting content, Bard may be generating more detectable artifacts and Claude is generating less artifacts.
Results: Self-Detecting Paraphrased Content
The researchers hypothesized that AI models would be able to self-detect their own paraphrased text because the artifacts that are created by the model (as detected in the original essays) should also be present in the rewritten text.
However the researchers acknowledged that the prompts for writing the text and paraphrasing are different because each rewrite is different than the original text which could consequently lead to a different self-detection results for the self-detection of paraphrased text.
The results of the self-detection of paraphrased text was indeed different from the self-detection of the original essay test.
- Bard was able to self-detect the paraphrased content at a similar rate.
- ChatGPT was not able to self-detect the paraphrased content at a rate much higher than the 50% rate (which is equal to guessing).
- ZeroGPT performance was similar to the results in the previous test, performing slightly worse.
Perhaps the most interesting result was turned in by Anthropic’s Claude.
Claude was able to self-detect the paraphrased content (but it was not able to detect the original essay in the previous test).
It’s an interesting result that Claude’s original essays apparently had so few artifacts to signal that it was AI generated that even Claude was unable to detect it.
Yet it was able to self-detect the paraphrase while ZeroGPT could not.
The researchers remarked on this test:
“The finding that paraphrasing prevents ChatGPT from self-detecting while increasing Claude’s ability to self-detect is very interesting and may be the result of the inner workings of these two transformer models.”
Screenshot of Self-Detection of AI Paraphrased Content
These tests yielded almost unpredictable results, particularly with regard to Anthropic’s Claude and this trend continued with the test of how well the AI models detected each others content, which had an interesting wrinkle.
Results: AI Models Detecting Each Other’s Content
The next test showed how well each AI model was at detecting the content generated by the other AI models.
If it’s true that Bard generates more artifacts than the other models, will the other models be able to easily detect Bard-generated content?
The results show that yes, Bard-generated content is the easiest to detect by the other AI models.
Regarding detecting ChatGPT generated content, both Claude and Bard were unable to detect it as AI-generated (justa as Claude was unable to detect it).
ChatGPT was able to detect Claude-generated content at a higher rate than both Bard and Claude but that higher rate was not much better than guessing.
The finding here is that all of them weren’t so good at detecting each others content, which the researchers opined may show that self-detection was a promising area of study.
Here is the graph that shows the results of this specific test:
At this point it should be noted that the researchers don’t claim that these results are conclusive about AI detection in general. The focus of the research was testing to see if AI models could succeed at self-detecting their own generated content. The answer is mostly yes, they do a better job at self-detecting but the results are similar to what was found with ZEROGpt.
The researchers commented:
“Self-detection shows similar detection power compared to ZeroGPT, but note that the goal of this study is not to claim that self-detection is superior to other methods, which would require a large study to compare to many state-of-the-art AI content detection tools. Here, we only investigate the models’ basic ability of self detection.”
Conclusions And Takeaways
The results of the test confirm that detecting AI generated content is not an easy task. Bard is able to detect its own content and paraphrased content.
ChatGPT can detect its own content but works less well on its paraphrased content.
Claude is the standout because it’s not able to reliably self-detect its own content but it was able to detect the paraphrased content, which was kind of weird and unexpected.
Detecting Claude’s original essays and the paraphrased essays was a challenge for ZeroGPT and for the other AI models.
The researchers noted about the Claude results:
“This seemingly inconclusive result needs more consideration since it is driven by two conflated causes.
1) The ability of the model to create text with very few detectable artifacts. Since the goal of these systems is to generate human-like text, fewer artifacts that are harder to detect means the model gets closer to that goal.
2) The inherent ability of the model to self-detect can be affected by the used architecture, the prompt, and the applied fine-tuning.”
The researchers had this further observation about Claude:
“Only Claude cannot be detected. This indicates that Claude might produce fewer detectable artifacts than the other models.
The detection rate of self-detection follows the same trend, indicating that Claude creates text with fewer artifacts, making it harder to distinguish from human writing”.
But of course, the weird part is that Claude was also unable to self-detect its own original content, unlike the other two models which had a higher success rate.
The researchers indicated that self-detection remains an interesting area for continued research and propose that further studies can focus on larger datasets with a greater diversity of AI-generated text, test additional AI models, a comparison with more AI detectors and lastly they suggested studying how prompt engineering may influence detection levels.
Read the original research paper and the abstract here:
AI Content Self-Detection for Transformer-based Large Language Models
Featured Image by Shutterstock/SObeR 9426
SEO
Big Update To Google’s Ranking Drop Documentation
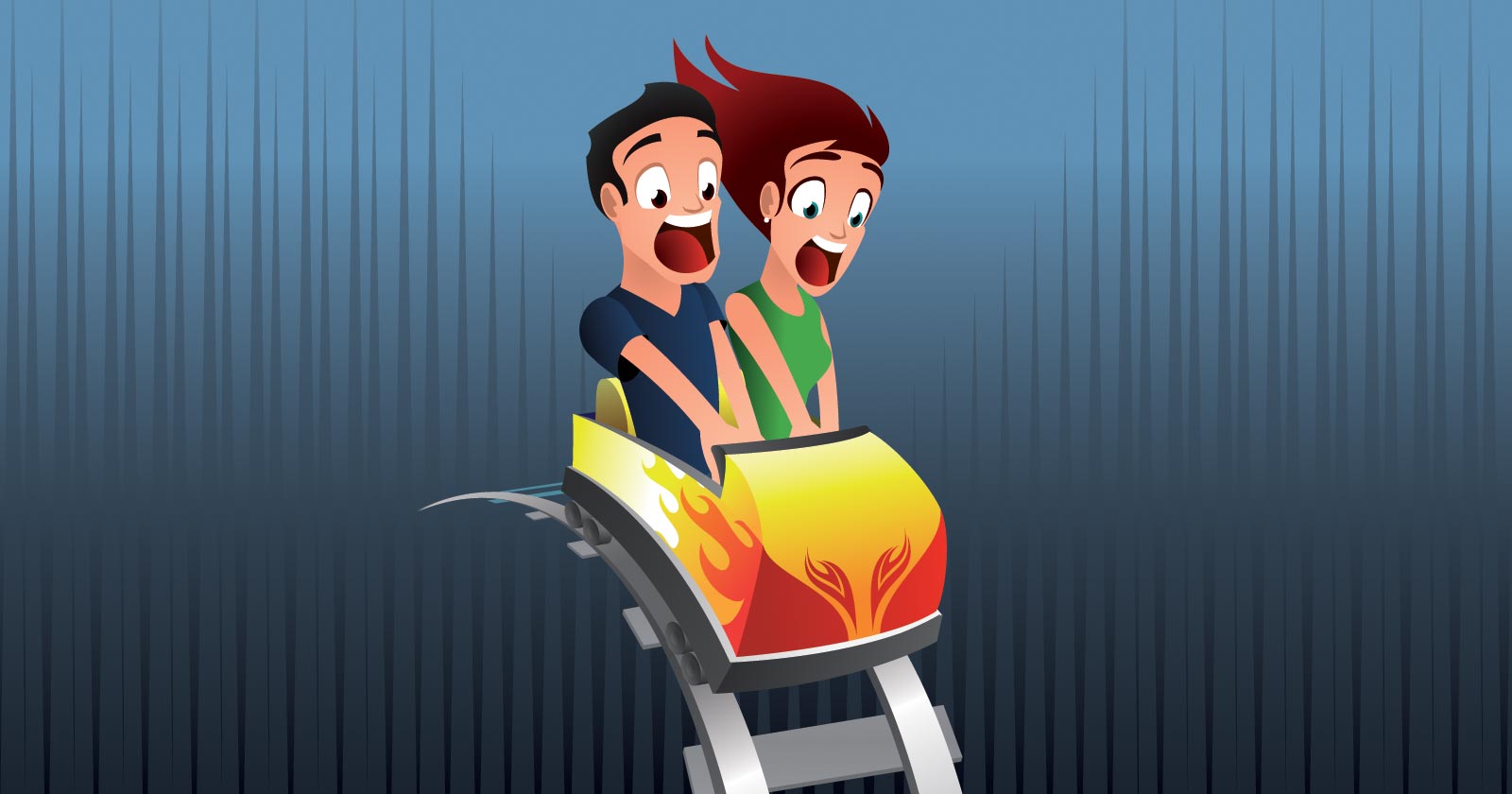
Google updated their guidance with five changes on how to debug ranking drops. The new version contains over 400 more words that address small and large ranking drops. There’s room to quibble about some of the changes but overall the revised version is a step up from what it replaced.
Change# 1: Downplays Fixing Traffic Drops
The opening sentence was changed so that it offers less hope for bouncing back from an algorithmic traffic drop. Google also joined two sentences into one sentence in the revised version of the documentation.
The documentation previously said that most traffic drops can be reversed and that identifying the reasons for a drop aren’t straightforward. The part about most of them can be reversed was completely removed.
Here is the original two sentences:
“A drop in organic Search traffic can happen for several reasons, and most of them can be reversed. It may not be straightforward to understand what exactly happened to your site”
Now there’s no hope offered for “most of them can be reversed” and more emphasis on understanding what happened is not straightforward.
This is the new guidance
“A drop in organic Search traffic can happen for several reasons, and it may not be straightforward to understand what exactly happened to your site.”
Change #2 Security Or Spam Issues
Google updated the traffic graph illustrations so that they precisely align with the causes for each kind of traffic decline.
The previous version of the graph was labeled:
“Site-level technical issue (Manual Action, strong algorithmic changes)”
The problem with the previous label is that manual actions and strong algorithmic changes are not technical issues and the new version fixes that issue.
The updated version now reads:
“Large drop from an algorithmic update, site-wide security or spam issue”
Change #3 Technical Issues
There’s one more change to a graph label, also to make it more accurate.
This is how the previous graph was labeled:
“Page-level technical issue (algorithmic changes, market disruption)”
The updated graph is now labeled:
“Technical issue across your site, changing interests”
Now the graph and label are more specific as a sitewide change and “changing interests” is more general and covers a wider range of changes than market disruption. Changing interests includes market disruption (where a new product makes a previous one obsolete or less desirable) but it also includes products that go out of style or loses their trendiness.
Change #4 Google Adds New Guidance For Algorithmic Changes
The biggest change by far is their brand new section for algorithmic changes which replaces two smaller sections, one about policy violations and manual actions and a second one about algorithm changes.
The old version of this one section had 108 words. The updated version contains 443 words.
A section that’s particularly helpful is where the guidance splits algorithmic update damage into two categories.
Two New Categories:
- Small drop in position? For example, dropping from position 2 to 4.
- Large drop in position? For example, dropping from position 4 to 29.
The two new categories are perfect and align with what I’ve seen in the search results for sites that have lost rankings. The reasons for dropping up and down within the top ten are different from the reasons why a site drops completely out of the top ten.
I don’t agree with the guidance for large drops. They recommend reviewing your site for large drops, which is good advice for some sites that have lost rankings. But in other cases there’s nothing wrong with the site and this is where less experienced SEOs tend to be unable to fix the problems because there’s nothing wrong with the site. Recommendations for improving EEAT, adding author bios or filing link disavows do not solve what’s going on because there’s nothing wrong with the site. The problem is something else in some of the cases.
Here is the new guidance for debugging search position drops:
“Algorithmic update
Google is always improving how it assesses content and updating its search ranking and serving algorithms accordingly; core updates and other smaller updates may change how some pages perform in Google Search results. We post about notable improvements to our systems on our list of ranking updates page; check it to see if there’s anything that’s applicable to your site.If you suspect a drop in traffic is due to an algorithmic update, it’s important to understand that there might not be anything fundamentally wrong with your content. To determine whether you need to make a change, review your top pages in Search Console and assess how they were ranking:
Small drop in position? For example, dropping from position 2 to 4.
Large drop in position? For example, dropping from position 4 to 29.Keep in mind that positions aren’t static or fixed in place. Google’s search results are dynamic in nature because the open web itself is constantly changing with new and updated content. This constant change can cause both gains and drops in organic Search traffic.
Small drop in position
A small drop in position is when there’s a small shift in position in the top results (for example, dropping from position 2 to 4 for a search query). In Search Console, you might see a noticeable drop in traffic without a big change in impressions.Small fluctuations in position can happen at any time (including moving back up in position, without you needing to do anything). In fact, we recommend avoiding making radical changes if your page is already performing well.
Large drop in position
A large drop in position is when you see a notable drop out of the top results for a wide range of terms (for example, dropping from the top 10 results to position 29).In cases like this, self-assess your whole website overall (not just individual pages) to make sure it’s helpful, reliable and people-first. If you’ve made changes to your site, it may take time to see an effect: some changes can take effect in a few days, while others could take several months. For example, it may take months before our systems determine that a site is now producing helpful content in the long term. In general, you’ll likely want to wait a few weeks to analyze your site in Search Console again to see if your efforts had a beneficial effect on ranking position.
Keep in mind that there’s no guarantee that changes you make to your website will result in noticeable impact in search results. If there’s more deserving content, it will continue to rank well with our systems.”
Change #5 Trivial Changes
The rest of the changes are relatively trivial but nonetheless makes the documentation more precise.
For example, one of the headings was changed from this:
You recently moved your site
To this new heading:
Site moves and migrations
Google’s Updated Ranking Drops Documentation
Google’s updated documentation is a well thought out but I think that the recommendations for large algorithmic drops are helpful for some cases and not helpful for other cases. I have 25 years of SEO experience and have experienced every single Google algorithm update. There are certain updates where the problem is not solved by trying to fix things and Google’s guidance used to be that sometimes there’s nothing to fix. The documentation is better but in my opinion it can be improved even further.
Read the new documentation here:
Debugging drops in Google Search traffic
Review the previous documentation:
Internet Archive Wayback Machine: Debugging drops in Google Search traffic
Featured Image by Shutterstock/Tomacco
SEO
Google March 2024 Core Update Officially Completed A Week Ago
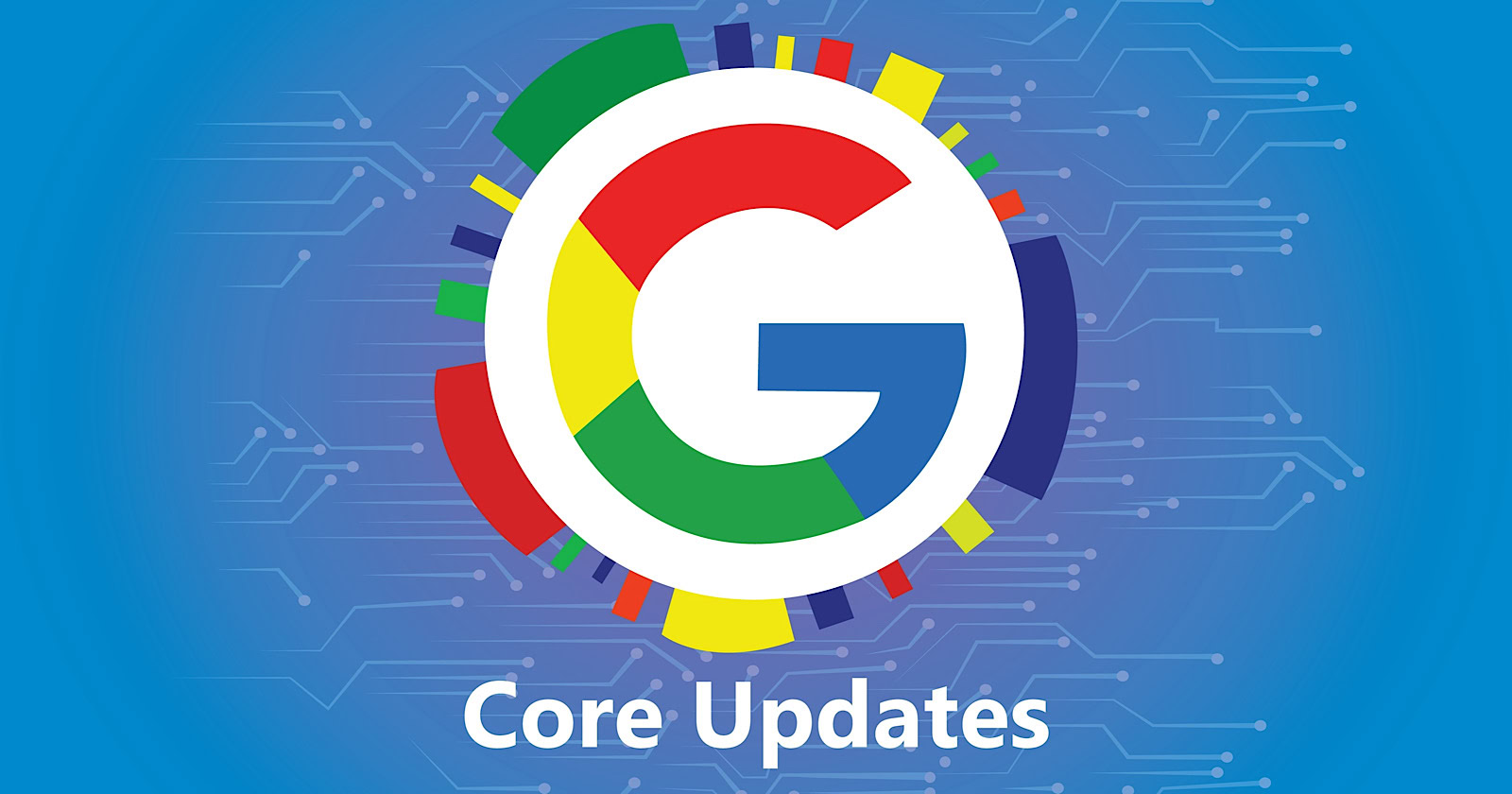
Google has officially completed its March 2024 Core Update, ending over a month of ranking volatility across the web.
However, Google didn’t confirm the rollout’s conclusion on its data anomaly page until April 26—a whole week after the update was completed on April 19.
Many in the SEO community had been speculating for days about whether the turbulent update had wrapped up.
The delayed transparency exemplifies Google’s communication issues with publishers and the need for clarity during core updates
Google March 2024 Core Update Timeline & Status
First announced on March 5, the core algorithm update is complete as of April 19. It took 45 days to complete.
Unlike more routine core refreshes, Google warned this one was more complex.
Google’s documentation reads:
“As this is a complex update, the rollout may take up to a month. It’s likely there will be more fluctuations in rankings than with a regular core update, as different systems get fully updated and reinforce each other.”
The aftershocks were tangible, with some websites reporting losses of over 60% of their organic search traffic, according to data from industry observers.
The ripple effects also led to the deindexing of hundreds of sites that were allegedly violating Google’s guidelines.
Addressing Manipulation Attempts
In its official guidance, Google highlighted the criteria it looks for when targeting link spam and manipulation attempts:
- Creating “low-value content” purely to garner manipulative links and inflate rankings.
- Links intended to boost sites’ rankings artificially, including manipulative outgoing links.
- The “repurposing” of expired domains with radically different content to game search visibility.
The updated guidelines warn:
“Any links that are intended to manipulate rankings in Google Search results may be considered link spam. This includes any behavior that manipulates links to your site or outgoing links from your site.”
John Mueller, a Search Advocate at Google, responded to the turbulence by advising publishers not to make rash changes while the core update was ongoing.
However, he suggested sites could proactively fix issues like unnatural paid links.
“If you have noticed things that are worth improving on your site, I’d go ahead and get things done. The idea is not to make changes just for search engines, right? Your users will be happy if you can make things better even if search engines haven’t updated their view of your site yet.”
Emphasizing Quality Over Links
The core update made notable changes to how Google ranks websites.
Most significantly, Google reduced the importance of links in determining a website’s ranking.
In contrast to the description of links as “an important factor in determining relevancy,” Google’s updated spam policies stripped away the “important” designation, simply calling links “a factor.”
This change aligns with Google’s Gary Illyes’ statements that links aren’t among the top three most influential ranking signals.
Instead, Google is giving more weight to quality, credibility, and substantive content.
Consequently, long-running campaigns favoring low-quality link acquisition and keyword optimizations have been demoted.
With the update complete, SEOs and publishers are left to audit their strategies and websites to ensure alignment with Google’s new perspective on ranking.
Core Update Feedback
Google has opened a ranking feedback form related to this core update.
You can use this form until May 31 to provide feedback to Google’s Search team about any issues noticed after the core update.
While the feedback provided won’t be used to make changes for specific queries or websites, Google says it may help inform general improvements to its search ranking systems for future updates.
Google also updated its help documentation on “Debugging drops in Google Search traffic” to help people understand ranking changes after a core update.
Featured Image: Rohit-Tripathi/Shutterstock
FAQ
After the update, what steps should websites take to align with Google’s new ranking criteria?
After Google’s March 2024 Core Update, websites should:
- Improve the quality, trustworthiness, and depth of their website content.
- Stop heavily focusing on getting as many links as possible and prioritize relevant, high-quality links instead.
- Fix any shady or spam-like SEO tactics on their sites.
- Carefully review their SEO strategies to ensure they follow Google’s new guidelines.
SEO
Google Declares It The “Gemini Era” As Revenue Grows 15%
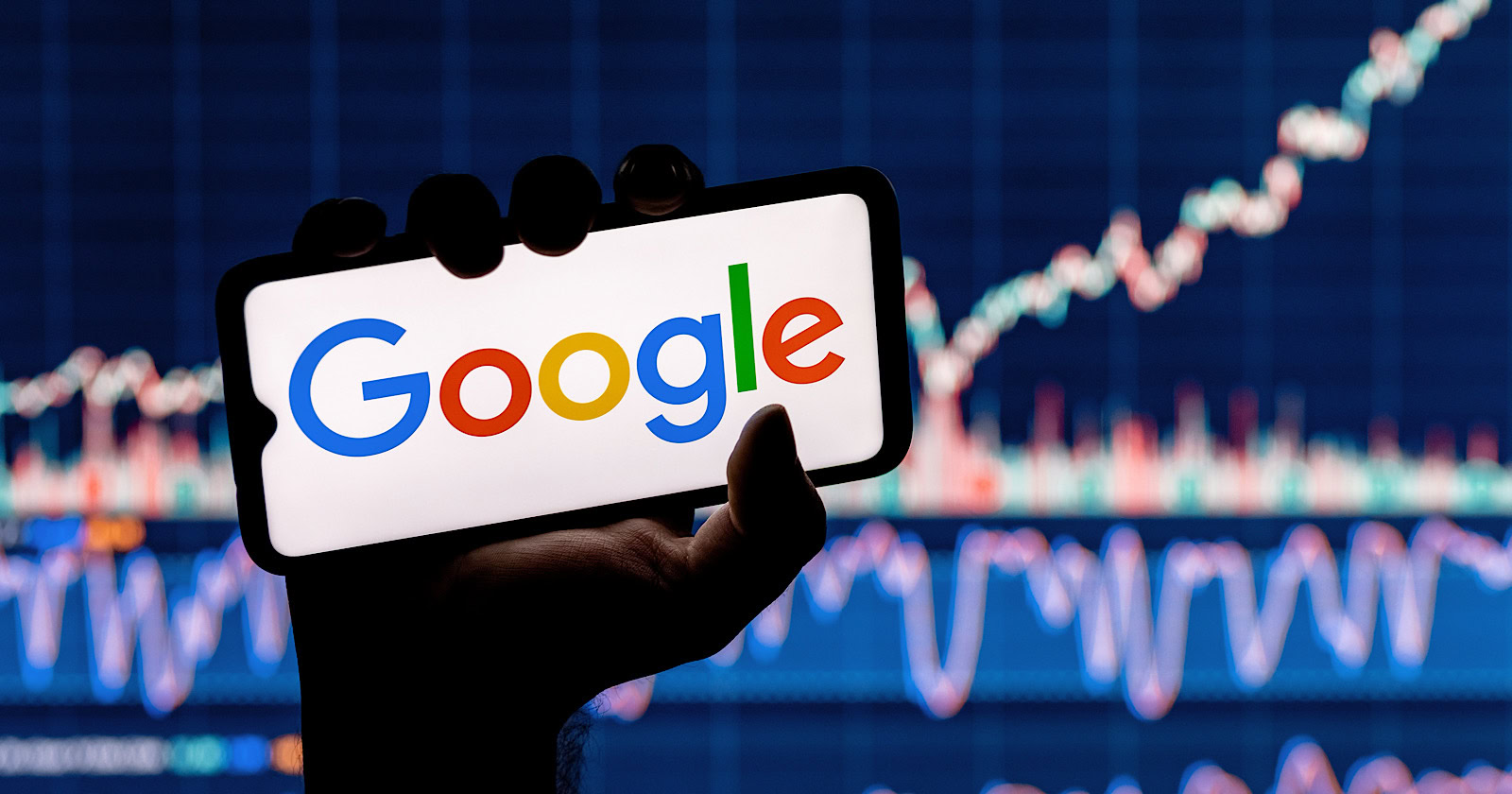
Alphabet Inc., Google’s parent company, announced its first quarter 2024 financial results today.
While Google reported double-digit growth in key revenue areas, the focus was on its AI developments, dubbed the “Gemini era” by CEO Sundar Pichai.
The Numbers: 15% Revenue Growth, Operating Margins Expand
Alphabet reported Q1 revenues of $80.5 billion, a 15% increase year-over-year, exceeding Wall Street’s projections.
Net income was $23.7 billion, with diluted earnings per share of $1.89. Operating margins expanded to 32%, up from 25% in the prior year.
Ruth Porat, Alphabet’s President and CFO, stated:
“Our strong financial results reflect revenue strength across the company and ongoing efforts to durably reengineer our cost base.”
Google’s core advertising units, such as Search and YouTube, drove growth. Google advertising revenues hit $61.7 billion for the quarter.
The Cloud division also maintained momentum, with revenues of $9.6 billion, up 28% year-over-year.
Pichai highlighted that YouTube and Cloud are expected to exit 2024 at a combined $100 billion annual revenue run rate.
Generative AI Integration in Search
Google experimented with AI-powered features in Search Labs before recently introducing AI overviews into the main search results page.
Regarding the gradual rollout, Pichai states:
“We are being measured in how we do this, focusing on areas where gen AI can improve the Search experience, while also prioritizing traffic to websites and merchants.”
Pichai reports that Google’s generative AI features have answered over a billion queries already:
“We’ve already served billions of queries with our generative AI features. It’s enabling people to access new information, to ask questions in new ways, and to ask more complex questions.”
Google reports increased Search usage and user satisfaction among those interacting with the new AI overview results.
The company also highlighted its “Circle to Search” feature on Android, which allows users to circle objects on their screen or in videos to get instant AI-powered answers via Google Lens.
Reorganizing For The “Gemini Era”
As part of the AI roadmap, Alphabet is consolidating all teams building AI models under the Google DeepMind umbrella.
Pichai revealed that, through hardware and software improvements, the company has reduced machine costs associated with its generative AI search results by 80% over the past year.
He states:
“Our data centers are some of the most high-performing, secure, reliable and efficient in the world. We’ve developed new AI models and algorithms that are more than one hundred times more efficient than they were 18 months ago.
How Will Google Make Money With AI?
Alphabet sees opportunities to monetize AI through its advertising products, Cloud offerings, and subscription services.
Google is integrating Gemini into ad products like Performance Max. The company’s Cloud division is bringing “the best of Google AI” to enterprise customers worldwide.
Google One, the company’s subscription service, surpassed 100 million paid subscribers in Q1 and introduced a new premium plan featuring advanced generative AI capabilities powered by Gemini models.
Future Outlook
Pichai outlined six key advantages positioning Alphabet to lead the “next wave of AI innovation”:
- Research leadership in AI breakthroughs like the multimodal Gemini model
- Robust AI infrastructure and custom TPU chips
- Integrating generative AI into Search to enhance the user experience
- A global product footprint reaching billions
- Streamlined teams and improved execution velocity
- Multiple revenue streams to monetize AI through advertising and cloud
With upcoming events like Google I/O and Google Marketing Live, the company is expected to share further updates on its AI initiatives and product roadmap.
Featured Image: Sergei Elagin/Shutterstock
-
WORDPRESS7 days ago
13 Best HubSpot Alternatives for 2024 (Free + Paid)
-
MARKETING6 days ago
Advertising in local markets: A playbook for success
-
SEARCHENGINES6 days ago
Google Core Update Flux, AdSense Ad Intent, California Link Tax & More
-
SEARCHENGINES5 days ago
Google Needs Very Few Links To Rank Pages; Links Are Less Important
-
MARKETING5 days ago
How to Use AI For a More Effective Social Media Strategy, According to Ross Simmonds
-
SEO5 days ago
How to Become an SEO Lead (10 Tips That Advanced My Career)
-
PPC4 days ago
10 Most Effective Franchise Marketing Strategies
-
SEARCHENGINES3 days ago
Google Won’t Change The 301 Signals For Ranking & SEO