SEO
Everything You Need To Know
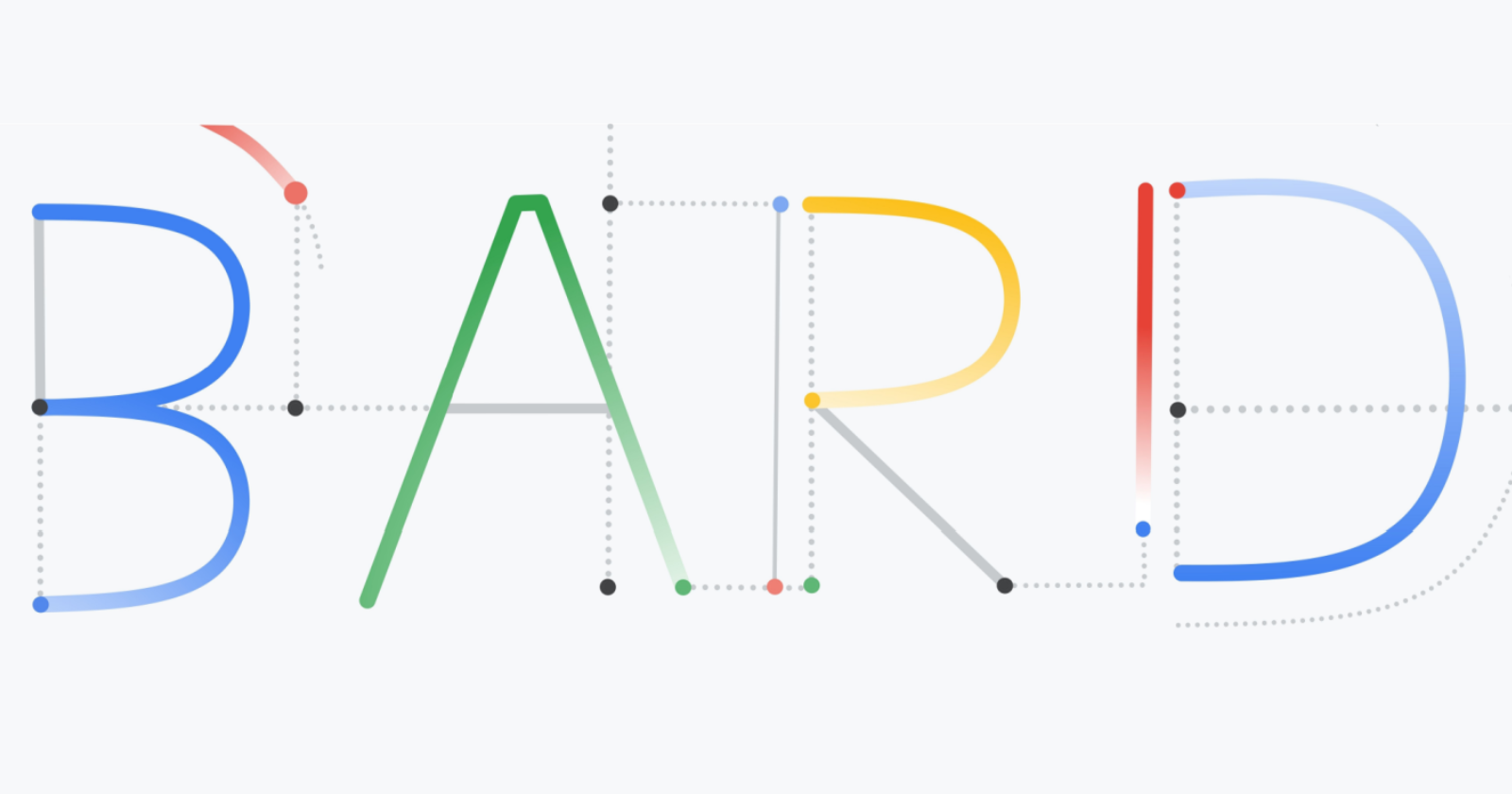
Google has just released Bard, its answer to ChatGPT, and users are getting to know it to see how it compares to OpenAI’s artificial intelligence-powered chatbot.
The name ‘Bard’ is purely marketing-driven, as there are no algorithms named Bard, but we do know that the chatbot is powered by LaMDA.
Here is everything we know about Bard so far and some interesting research that may offer an idea of the kind of algorithms that may power Bard.
What Is Google Bard?
Bard is an experimental Google chatbot that is powered by the LaMDA large language model.
It’s a generative AI that accepts prompts and performs text-based tasks like providing answers and summaries and creating various forms of content.
Bard also assists in exploring topics by summarizing information found on the internet and providing links for exploring websites with more information.
Why Did Google Release Bard?
Google released Bard after the wildly successful launch of OpenAI’s ChatGPT, which created the perception that Google was falling behind technologically.
ChatGPT was perceived as a revolutionary technology with the potential to disrupt the search industry and shift the balance of power away from Google search and the lucrative search advertising business.
On December 21, 2022, three weeks after the launch of ChatGPT, the New York Times reported that Google had declared a “code red” to quickly define its response to the threat posed to its business model.
Forty-seven days after the code red strategy adjustment, Google announced the launch of Bard on February 6, 2023.
What Was The Issue With Google Bard?
The announcement of Bard was a stunning failure because the demo that was meant to showcase Google’s chatbot AI contained a factual error.
The inaccuracy of Google’s AI turned what was meant to be a triumphant return to form into a humbling pie in the face.
Google’s shares subsequently lost a hundred billion dollars in market value in a single day, reflecting a loss of confidence in Google’s ability to navigate the looming era of AI.
How Does Google Bard Work?
Bard is powered by a “lightweight” version of LaMDA.
LaMDA is a large language model that is trained on datasets consisting of public dialogue and web data.
There are two important factors related to the training described in the associated research paper, which you can download as a PDF here: LaMDA: Language Models for Dialog Applications (read the abstract here).
- A. Safety: The model achieves a level of safety by tuning it with data that was annotated by crowd workers.
- B. Groundedness: LaMDA grounds itself factually with external knowledge sources (through information retrieval, which is search).
The LaMDA research paper states:
“…factual grounding, involves enabling the model to consult external knowledge sources, such as an information retrieval system, a language translator, and a calculator.
We quantify factuality using a groundedness metric, and we find that our approach enables the model to generate responses grounded in known sources, rather than responses that merely sound plausible.”
Google used three metrics to evaluate the LaMDA outputs:
- Sensibleness: A measurement of whether an answer makes sense or not.
- Specificity: Measures if the answer is the opposite of generic/vague or contextually specific.
- Interestingness: This metric measures if LaMDA’s answers are insightful or inspire curiosity.
All three metrics were judged by crowdsourced raters, and that data was fed back into the machine to keep improving it.
The LaMDA research paper concludes by stating that crowdsourced reviews and the system’s ability to fact-check with a search engine were useful techniques.
Google’s researchers wrote:
“We find that crowd-annotated data is an effective tool for driving significant additional gains.
We also find that calling external APIs (such as an information retrieval system) offers a path towards significantly improving groundedness, which we define as the extent to which a generated response contains claims that can be referenced and checked against a known source.”
How Is Google Planning To Use Bard In Search?
The future of Bard is currently envisioned as a feature in search.
Google’s announcement in February was insufficiently specific on how Bard would be implemented.
The key details were buried in a single paragraph close to the end of the blog announcement of Bard, where it was described as an AI feature in search.
That lack of clarity fueled the perception that Bard would be integrated into search, which was never the case.
Google’s February 2023 announcement of Bard states that Google will at some point integrate AI features into search:
“Soon, you’ll see AI-powered features in Search that distill complex information and multiple perspectives into easy-to-digest formats, so you can quickly understand the big picture and learn more from the web: whether that’s seeking out additional perspectives, like blogs from people who play both piano and guitar, or going deeper on a related topic, like steps to get started as a beginner.
These new AI features will begin rolling out on Google Search soon.”
It’s clear that Bard is not search. Rather, it is intended to be a feature in search and not a replacement for search.
What Is A Search Feature?
A feature is something like Google’s Knowledge Panel, which provides knowledge information about notable people, places, and things.
Google’s “How Search Works” webpage about features explains:
“Google’s search features ensure that you get the right information at the right time in the format that’s most useful to your query.
Sometimes it’s a webpage, and sometimes it’s real-world information like a map or inventory at a local store.”
In an internal meeting at Google (reported by CNBC), employees questioned the use of Bard in search.
One employee pointed out that large language models like ChatGPT and Bard are not fact-based sources of information.
The Google employee asked:
“Why do we think the big first application should be search, which at its heart is about finding true information?”
Jack Krawczyk, the product lead for Google Bard, answered:
“I just want to be very clear: Bard is not search.”
At the same internal event, Google’s Vice President of Engineering for Search, Elizabeth Reid, reiterated that Bard is not search.
She said:
“Bard is really separate from search…”
What we can confidently conclude is that Bard is not a new iteration of Google search. It is a feature.
Bard Is An Interactive Method For Exploring Topics
Google’s announcement of Bard was fairly explicit that Bard is not search. This means that, while search surfaces links to answers, Bard helps users investigate knowledge.
The announcement explains:
“When people think of Google, they often think of turning to us for quick factual answers, like ‘how many keys does a piano have?’
But increasingly, people are turning to Google for deeper insights and understanding – like, ‘is the piano or guitar easier to learn, and how much practice does each need?’
Learning about a topic like this can take a lot of effort to figure out what you really need to know, and people often want to explore a diverse range of opinions or perspectives.”
It may be helpful to think of Bard as an interactive method for accessing knowledge about topics.
Bard Samples Web Information
The problem with large language models is that they mimic answers, which can lead to factual errors.
The researchers who created LaMDA state that approaches like increasing the size of the model can help it gain more factual information.
But they noted that this approach fails in areas where facts are constantly changing during the course of time, which researchers refer to as the “temporal generalization problem.”
Freshness in the sense of timely information cannot be trained with a static language model.
The solution that LaMDA pursued was to query information retrieval systems. An information retrieval system is a search engine, so LaMDA checks search results.
This feature from LaMDA appears to be a feature of Bard.
The Google Bard announcement explains:
“Bard seeks to combine the breadth of the world’s knowledge with the power, intelligence, and creativity of our large language models.
It draws on information from the web to provide fresh, high-quality responses.”
LaMDA and (possibly by extension) Bard achieve this with what is called the toolset (TS).
The toolset is explained in the LaMDA researcher paper:
“We create a toolset (TS) that includes an information retrieval system, a calculator, and a translator.
TS takes a single string as input and outputs a list of one or more strings. Each tool in TS expects a string and returns a list of strings.
For example, the calculator takes “135+7721”, and outputs a list containing [“7856”]. Similarly, the translator can take “hello in French” and output [‘Bonjour’].
Finally, the information retrieval system can take ‘How old is Rafael Nadal?’, and output [‘Rafael Nadal / Age / 35’].
The information retrieval system is also capable of returning snippets of content from the open web, with their corresponding URLs.
The TS tries an input string on all of its tools, and produces a final output list of strings by concatenating the output lists from every tool in the following order: calculator, translator, and information retrieval system.
A tool will return an empty list of results if it can’t parse the input (e.g., the calculator cannot parse ‘How old is Rafael Nadal?’), and therefore does not contribute to the final output list.”
Here’s a Bard response with a snippet from the open web:
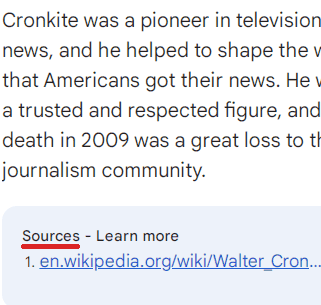
Conversational Question-Answering Systems
There are no research papers that mention the name “Bard.”
However, there is quite a bit of recent research related to AI, including by scientists associated with LaMDA, that may have an impact on Bard.
The following doesn’t claim that Google is using these algorithms. We can’t say for certain that any of these technologies are used in Bard.
The value in knowing about these research papers is in knowing what is possible.
The following are algorithms relevant to AI-based question-answering systems.
One of the authors of LaMDA worked on a project that’s about creating training data for a conversational information retrieval system.
You can download the 2022 research paper as a PDF here: Dialog Inpainting: Turning Documents into Dialogs (and read the abstract here).
The problem with training a system like Bard is that question-and-answer datasets (like datasets comprised of questions and answers found on Reddit) are limited to how people on Reddit behave.
It doesn’t encompass how people outside of that environment behave and the kinds of questions they would ask, and what the correct answers to those questions would be.
The researchers explored creating a system read webpages, then used a “dialog inpainter” to predict what questions would be answered by any given passage within what the machine was reading.
A passage in a trustworthy Wikipedia webpage that says, “The sky is blue,” could be turned into the question, “What color is the sky?”
The researchers created their own dataset of questions and answers using Wikipedia and other webpages. They called the datasets WikiDialog and WebDialog.
- WikiDialog is a set of questions and answers derived from Wikipedia data.
- WebDialog is a dataset derived from webpage dialog on the internet.
These new datasets are 1,000 times larger than existing datasets. The importance of that is it gives conversational language models an opportunity to learn more.
The researchers reported that this new dataset helped to improve conversational question-answering systems by over 40%.
The research paper describes the success of this approach:
“Importantly, we find that our inpainted datasets are powerful sources of training data for ConvQA systems…
When used to pre-train standard retriever and reranker architectures, they advance state-of-the-art across three different ConvQA retrieval benchmarks (QRECC, OR-QUAC, TREC-CAST), delivering up to 40% relative gains on standard evaluation metrics…
Remarkably, we find that just pre-training on WikiDialog enables strong zero-shot retrieval performance—up to 95% of a finetuned retriever’s performance—without using any in-domain ConvQA data. “
Is it possible that Google Bard was trained using the WikiDialog and WebDialog datasets?
It’s difficult to imagine a scenario where Google would pass on training a conversational AI on a dataset that is over 1,000 times larger.
But we don’t know for certain because Google doesn’t often comment on its underlying technologies in detail, except on rare occasions like for Bard or LaMDA.
Large Language Models That Link To Sources
Google recently published an interesting research paper about a way to make large language models cite the sources for their information. The initial version of the paper was published in December 2022, and the second version was updated in February 2023.
This technology is referred to as experimental as of December 2022.
You can download the PDF of the paper here: Attributed Question Answering: Evaluation and Modeling for Attributed Large Language Models (read the Google abstract here).
The research paper states the intent of the technology:
“Large language models (LLMs) have shown impressive results while requiring little or no direct supervision.
Further, there is mounting evidence that LLMs may have potential in information-seeking scenarios.
We believe the ability of an LLM to attribute the text that it generates is likely to be crucial in this setting.
We formulate and study Attributed QA as a key first step in the development of attributed LLMs.
We propose a reproducible evaluation framework for the task and benchmark a broad set of architectures.
We take human annotations as a gold standard and show that a correlated automatic metric is suitable for development.
Our experimental work gives concrete answers to two key questions (How to measure attribution?, and How well do current state-of-the-art methods perform on attribution?), and give some hints as to how to address a third (How to build LLMs with attribution?).”
This kind of large language model can train a system that can answer with supporting documentation that, theoretically, assures that the response is based on something.
The research paper explains:
“To explore these questions, we propose Attributed Question Answering (QA). In our formulation, the input to the model/system is a question, and the output is an (answer, attribution) pair where answer is an answer string, and attribution is a pointer into a fixed corpus, e.g., of paragraphs.
The returned attribution should give supporting evidence for the answer.”
This technology is specifically for question-answering tasks.
The goal is to create better answers – something that Google would understandably want for Bard.
- Attribution allows users and developers to assess the “trustworthiness and nuance” of the answers.
- Attribution allows developers to quickly review the quality of the answers since the sources are provided.
One interesting note is a new technology called AutoAIS that strongly correlates with human raters.
In other words, this technology can automate the work of human raters and scale the process of rating the answers given by a large language model (like Bard).
The researchers share:
“We consider human rating to be the gold standard for system evaluation, but find that AutoAIS correlates well with human judgment at the system level, offering promise as a development metric where human rating is infeasible, or even as a noisy training signal. “
This technology is experimental; it’s probably not in use. But it does show one of the directions that Google is exploring for producing trustworthy answers.
Research Paper On Editing Responses For Factuality
Lastly, there’s a remarkable technology developed at Cornell University (also dating from the end of 2022) that explores a different way to source attribution for what a large language model outputs and can even edit an answer to correct itself.
Cornell University (like Stanford University) licenses technology related to search and other areas, earning millions of dollars per year.
It’s good to keep up with university research because it shows what is possible and what is cutting-edge.
You can download a PDF of the paper here: RARR: Researching and Revising What Language Models Say, Using Language Models (and read the abstract here).
The abstract explains the technology:
“Language models (LMs) now excel at many tasks such as few-shot learning, question answering, reasoning, and dialog.
However, they sometimes generate unsupported or misleading content.
A user cannot easily determine whether their outputs are trustworthy or not, because most LMs do not have any built-in mechanism for attribution to external evidence.
To enable attribution while still preserving all the powerful advantages of recent generation models, we propose RARR (Retrofit Attribution using Research and Revision), a system that 1) automatically finds attribution for the output of any text generation model and 2) post-edits the output to fix unsupported content while preserving the original output as much as possible.
…we find that RARR significantly improves attribution while otherwise preserving the original input to a much greater degree than previously explored edit models.
Furthermore, the implementation of RARR requires only a handful of training examples, a large language model, and standard web search.”
How Do I Get Access To Google Bard?
Google is currently accepting new users to test Bard, which is currently labeled as experimental. Google is rolling out access for Bard here.
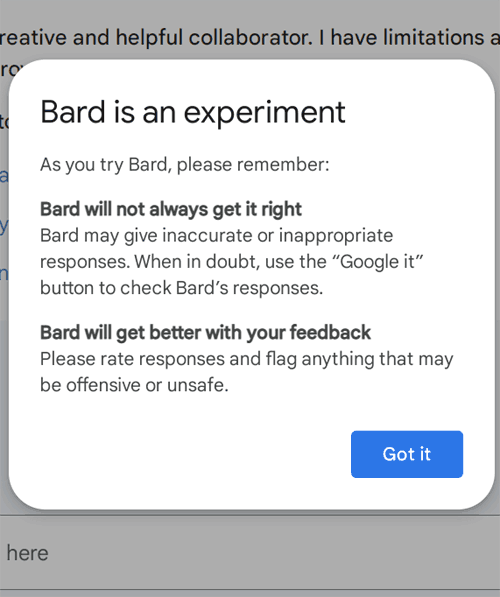
Google is on the record saying that Bard is not search, which should reassure those who feel anxiety about the dawn of AI.
We are at a turning point that is unlike any we’ve seen in, perhaps, a decade.
Understanding Bard is helpful to anyone who publishes on the web or practices SEO because it’s helpful to know the limits of what is possible and the future of what can be achieved.
More Resources:
Featured Image: Whyredphotographor/Shutterstock