SEO
Leaked Google Memo Admits Defeat By Open Source AI
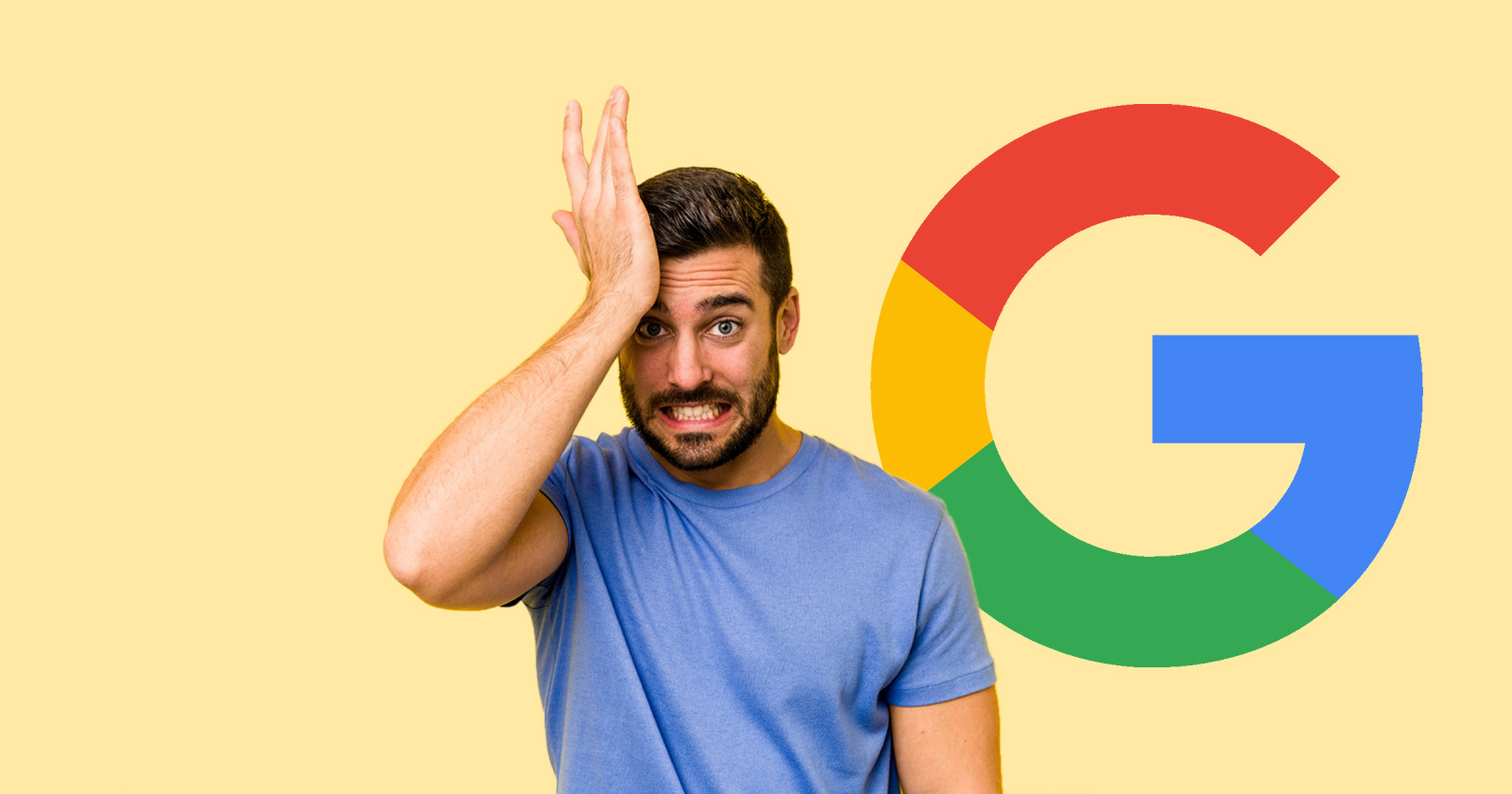
A leaked Google memo offers a point by point summary of why Google is losing to open source AI and suggests a path back to dominance and owning the platform.
The memo opens by acknowledging their competitor was never OpenAI and was always going to be Open Source.
Cannot Compete Against Open Source
Further, they admit that they are not positioned in any way to compete against open source, acknowledging that they have already lost the struggle for AI dominance.
They wrote:
“We’ve done a lot of looking over our shoulders at OpenAI. Who will cross the next milestone? What will the next move be?
But the uncomfortable truth is, we aren’t positioned to win this arms race and neither is OpenAI. While we’ve been squabbling, a third faction has been quietly eating our lunch.
I’m talking, of course, about open source.
Plainly put, they are lapping us. Things we consider “major open problems” are solved and in people’s hands today.”
The bulk of the memo is spent describing how Google is outplayed by open source.
And even though Google has a slight advantage over open source, the author of the memo acknowledges that it is slipping away and will never return.
The self-analysis of the metaphoric cards they’ve dealt themselves is considerably downbeat:
“While our models still hold a slight edge in terms of quality, the gap is closing astonishingly quickly.
Open-source models are faster, more customizable, more private, and pound-for-pound more capable.
They are doing things with $100 and 13B params that we struggle with at $10M and 540B.
And they are doing so in weeks, not months.”
Large Language Model Size is Not an Advantage
Perhaps the most chilling realization expressed in the memo is Google’s size is no longer an advantage.
The outlandishly large size of their models are now seen as disadvantages and not in any way the insurmountable advantage they thought them to be.
The leaked memo lists a series of events that signal Google’s (and OpenAI’s) control of AI may rapidly be over.
It recounts that barely a month ago, in March 2023, the open source community obtained a leaked open source model large language model developed by Meta called LLaMA.
Within days and weeks the global open source community developed all the building parts necessary to create Bard and ChatGPT clones.
Sophisticated steps such as instruction tuning and reinforcement learning from human feedback (RLHF) were quickly replicated by the global open source community, on the cheap no less.
- Instruction tuning
A process of fine-tuning a language model to make it do something specific that it wasn’t initially trained to do. - Reinforcement learning from human feedback (RLHF)
A technique where humans rate a language models output so that it learns which outputs are satisfactory to humans.
RLHF is the technique used by OpenAI to create InstructGPT, which is a model underlying ChatGPT and allows the GPT-3.5 and GPT-4 models to take instructions and complete tasks.
RLHF is the fire that open source has taken from
Scale of Open Source Scares Google
What scares Google in particular is the fact that the Open Source movement is able to scale their projects in a way that closed source cannot.
The question and answer dataset used to create the open source ChatGPT clone, Dolly 2.0, was entirely created by thousands of employee volunteers.
Google and OpenAI relied partially on question and answers from scraped from sites like Reddit.
The open source Q&A dataset created by Databricks is claimed to be of a higher quality because the humans who contributed to creating it were professionals and the answers they provided were longer and more substantial than what is found in a typical question and answer dataset scraped from a public forum.
The leaked memo observed:
“At the beginning of March the open source community got their hands on their first really capable foundation model, as Meta’s LLaMA was leaked to the public.
It had no instruction or conversation tuning, and no RLHF.
Nonetheless, the community immediately understood the significance of what they had been given.
A tremendous outpouring of innovation followed, with just days between major developments…
Here we are, barely a month later, and there are variants with instruction tuning, quantization, quality improvements, human evals, multimodality, RLHF, etc. etc. many of which build on each other.
Most importantly, they have solved the scaling problem to the extent that anyone can tinker.
Many of the new ideas are from ordinary people.
The barrier to entry for training and experimentation has dropped from the total output of a major research organization to one person, an evening, and a beefy laptop.”
In other words, what took months and years for Google and OpenAI to train and build only took a matter of days for the open source community.
That has to be a truly frightening scenario to Google.
It’s one of the reasons why I’ve been writing so much about the open source AI movement as it truly looks like where the future of generative AI will be in a relatively short period of time.
Open Source Has Historically Surpassed Closed Source
The memo cites the recent experience with OpenAI’s DALL-E, the deep learning model used to create images versus the open source Stable Diffusion as a harbinger of what is currently befalling Generative AI like Bard and ChatGPT.
Dall-e was released by OpenAI in January 2021. Stable Diffusion, the open source version, was released a year and a half later in August 2022 and in a few short weeks overtook the popularity of Dall-E.
This timeline graph shows how fast Stable Diffusion overtook Dall-E:
The above Google Trends timeline shows how interest in the open source Stable Diffusion model vastly surpassed that of Dall-E within a matter of three weeks of its release.
And though Dall-E had been out for a year and a half, interest in Stable Diffusion kept soaring exponentially while OpenAI’s Dall-E remained stagnant.
The existential threat of similar events overtaking Bard (and OpenAI) is giving Google nightmares.
The Creation Process of Open Source Model is Superior
Another factor that’s alarming engineers at Google is that the process for creating and improving open source models is fast, inexpensive and lends itself perfectly to a global collaborative approach common to open source projects.
The memo observes that new techniques such as LoRA (Low-Rank Adaptation of Large Language Models), allow for the fine-tuning of language models in a matter of days with exceedingly low cost, with the final LLM comparable to the exceedingly more expensive LLMs created by Google and OpenAI.
Another benefit is that open source engineers can build on top of previous work, iterate, instead of having to start from scratch.
Building large language models with billions of parameters in the way that OpenAI and Google have been doing is not necessary today.
Which may be the point that Sam Alton recently was hinting at when he recently said that the era of massive large language models is over.
The author of the Google memo contrasted the cheap and fast LoRA approach to creating LLMs against the current big AI approach.
The memo author reflects on Google’s shortcoming:
“By contrast, training giant models from scratch not only throws away the pretraining, but also any iterative improvements that have been made on top. In the open source world, it doesn’t take long before these improvements dominate, making a full retrain extremely costly.
We should be thoughtful about whether each new application or idea really needs a whole new model.
…Indeed, in terms of engineer-hours, the pace of improvement from these models vastly outstrips what we can do with our largest variants, and the best are already largely indistinguishable from ChatGPT.”
The author concludes with the realization that what they thought was their advantage, their giant models and concomitant prohibitive cost, was actually a disadvantage.
The global-collaborative nature of Open Source is more efficient and orders of magnitude faster at innovation.
How can a closed-source system compete against the overwhelming multitude of engineers around the world?
The author concludes that they cannot compete and that direct competition is, in their words, a “losing proposition.”
That’s the crisis, the storm, that’s developing outside of Google.
If You Can’t Beat Open Source Join Them
The only consolation the memo author finds in open source is that because the open source innovations are free, Google can also take advantage of it.
Lastly, the author concludes that the only approach open to Google is to own the platform in the same way they dominate the open source Chrome and Android platforms.
They point to how Meta is benefiting from releasing their LLaMA large language model for research and how they now have thousands of people doing their work for free.
Perhaps the big takeaway from the memo then is that Google may in the near future try to replicate their open source dominance by releasing their projects on an open source basis and thereby own the platform.
The memo concludes that going open source is the most viable option:
“Google should establish itself a leader in the open source community, taking the lead by cooperating with, rather than ignoring, the broader conversation.
This probably means taking some uncomfortable steps, like publishing the model weights for small ULM variants. This necessarily means relinquishing some control over our models.
But this compromise is inevitable.
We cannot hope to both drive innovation and control it.”
Open Source Walks Away With the AI Fire
Last week I made an allusion to the Greek myth of the human hero Prometheus stealing fire from the gods on Mount Olympus, pitting the open source to Prometheus against the “Olympian gods” of Google and OpenAI:
I tweeted:
“While Google, Microsoft and Open AI squabble amongst each other and have their backs turned, is Open Source walking off with their fire?”
The leak of Google’s memo confirms that observation but it also points at a possible strategy change at Google to join the open source movement and thereby co-opt it and dominate it in the same way they did with Chrome and Android.
Read the leaked Google memo here:
Understanding the Impact of Google’s November 2024 Core Update on Global Search Rankings
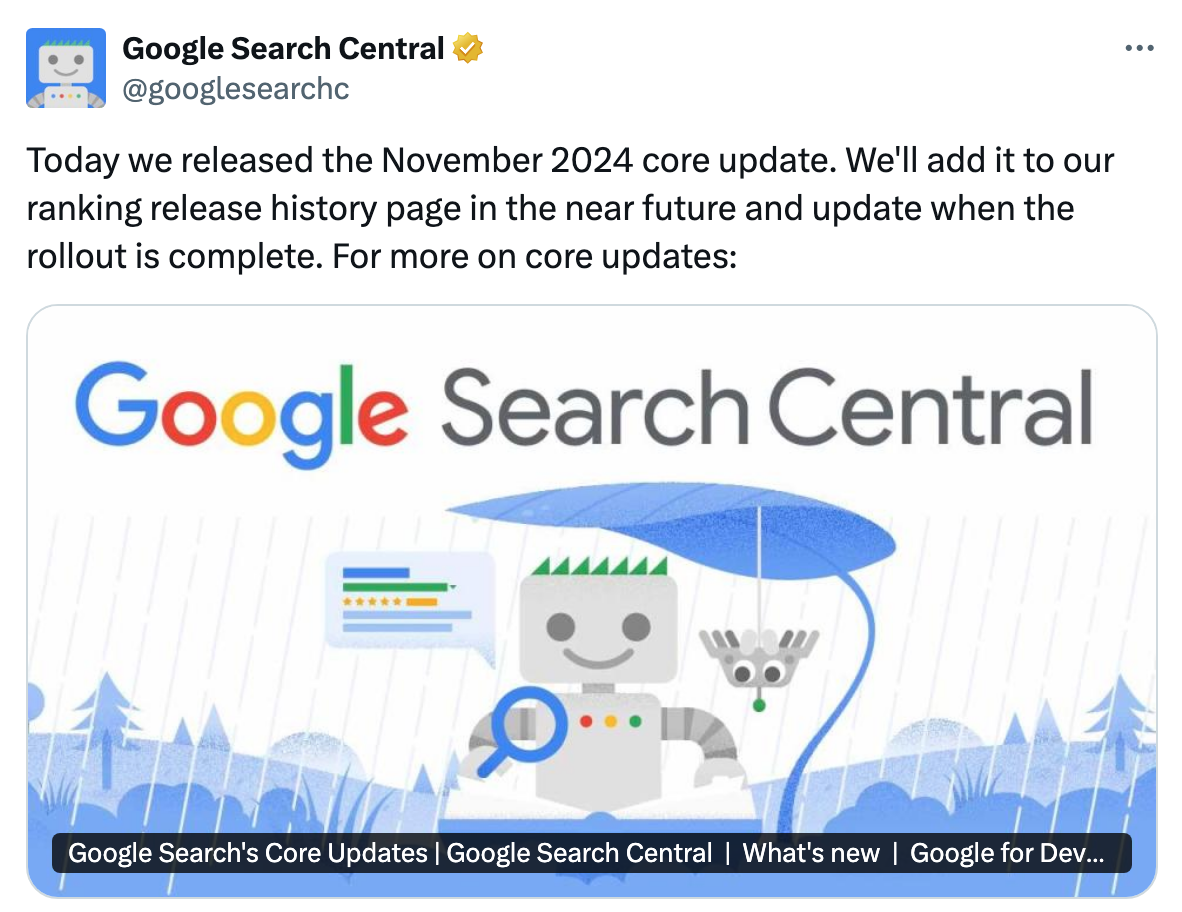
Introduction
In November 2024, Google launched its latest core algorithm update, a broad refinement designed to enhance the quality of its search engine results. Rolling out over approximately two weeks, the update continues Google’s ongoing commitment to delivering more relevant, useful, and high-quality search experiences for users worldwide. This article explores the nature of the November 2024 Core Update, its potential impact on websites, and strategies for site owners to adapt and thrive in its aftermath.
1. What Is a Google Core Update?
Core updates are large-scale changes to Google’s search algorithms. Unlike targeted updates aimed at specific sectors or issues, core updates broadly impact all regions and languages. They reflect Google’s effort to re-evaluate how content is assessed and ranked based on relevance, usefulness, and reliability. Previous updates include significant releases like the March and August 2024 updates, illustrating the frequency and scope of these changes.
2. Goals of the November 2024 Core Update
The November update focuses on refining the quality of search results. According to Google’s official statements, it seeks to amplify genuinely useful content while reducing the visibility of content primarily designed to manipulate rankings without meeting user needs. This effort emphasizes Google’s consistent push for “people-first” content—engaging and useful information that serves users, not search engines.
3. Key Features and Characteristics of the Update
- Global Impact: The update affects search rankings on a global scale and is not confined to any particular industry or niche.
- Rollout Duration: Spanning about two weeks, the rollout’s timing allows Google to fully implement algorithmic changes and assess their effects.
- Broad Adjustments: The update doesn’t target specific sites but involves systemic reassessment across Google’s ranking systems.
- Dynamic Search Environment: This core update follows in the footsteps of the August and March 2024 updates, representing a year of significant search result refinement.
4. What This Means for Site Owners
- Traffic Fluctuations: Websites may observe shifts in rankings and traffic during the update’s rollout and subsequent completion. These changes highlight the dynamic nature of Google search and require continuous monitoring and adaptation.
- Recommended Actions:
- Wait and Analyze: Site owners experiencing changes should wait until the rollout’s completion before making significant adjustments.
- Utilize Google Search Console: Compare traffic and ranking data from before and after the update to identify potential areas of improvement.
- Focus on High-Impact Pages: Pages with notable drops in ranking should undergo thorough content evaluation using Google’s guidelines
5. Recovery and Adaptation Strategies
Recovering from a negative impact due to a core update may take weeks or months as Google’s systems adjust and validate content changes. Site owners should prioritize delivering high-quality, reliable, and user-focused content. Specific steps include:
- Content Evaluation: Assess content against Google’s guidelines, focusing on readability, user satisfaction, and factual accuracy.
- No Quick Fixes: Avoid superficial changes aimed solely at improving rankings. Sustainable improvements are more valuable and impactful(November 2024 core upda…).
- People-First Content: Ensure content serves real user needs, as opposed to purely SEO-driven objectives. This aligns with Google’s long-term priorities for search quality
6. Comparative Analysis with Previous Updates
The November 2024 Core Update continues trends observed in previous updates like March and August 2024. While each update has its nuances, their collective goal remains consistent: bettering search quality and delivering relevant results. Comparing data from these updates can reveal patterns and offer insights into Google’s evolving criteria
7. Broader Implications for the SEO Industry
Google’s ongoing core updates underscore the critical importance of a user-centric approach to SEO. For digital marketers and SEO specialists, adapting strategies to these updates involves staying informed, using reliable analytics tools, and keeping content fresh and engaging. The need for adaptability is paramount, as Google continually shifts the parameters of what defines quality content
Conclusion
The November 2024 Core Update serves as a reminder that Google’s algorithmic changes are not designed to punish but to reward helpful, authentic, and user-focused content. Site owners and marketers who embrace this philosophy are better positioned to weather core updates and even benefit from improved rankings and traffic over time. By maintaining a focus on user experience, transparency, and relevance, creators can align with Google’s evolving standards and thrive in the ever-changing digital landscape
SEO
How to Revive an Old Blog Article for SEO
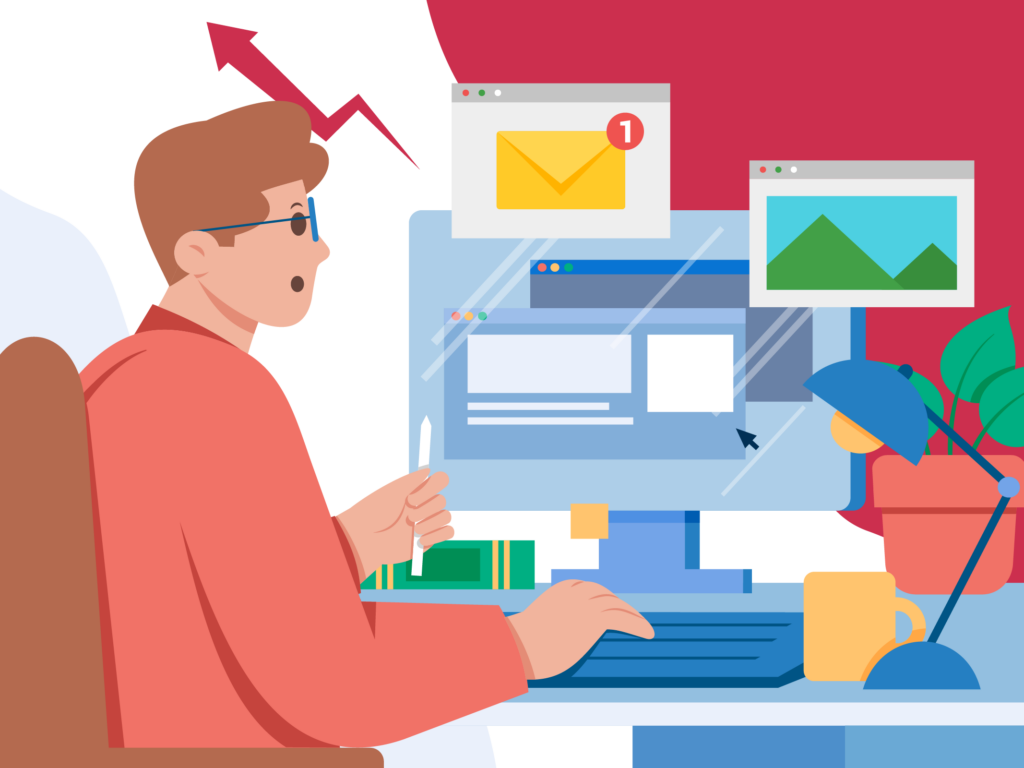
Quick question: What do you typically do with your old blog posts? Most likely, the answer is: Not much.
If that’s the case, you’re not alone. Many of us in SEO and content marketing tend to focus on continuously creating new content, rather than leveraging our existing blog posts.
However, here’s the reality—Google is becoming increasingly sophisticated in evaluating content quality, and we need to adapt accordingly. Just as it’s easier to encourage existing customers to make repeat purchases, updating old content on your website is a more efficient and sustainable strategy in the long run.
Ways to Optimize Older Content
Some of your old content might not be optimized for SEO very well, rank for irrelevant keywords, or drive no traffic at all. If the quality is still decent, however, you should be able to optimize it properly with little effort.
Refresh Content
If your blog post contains a specific year or mentions current events, it may become outdated over time. If the rest of the content is still relevant (like if it’s targeting an evergreen topic), simply updating the date might be all you need to do.
Rewrite Old Blog Posts
When the content quality is low (you might have greatly improved your writing skills since you’ve written the post) but the potential is still there, there’s not much you can do apart from rewriting an old blog post completely.
This is not a waste—you’re saving time on brainstorming since the basic structure is already in place. Now, focus on improving the quality.
Delete Old Blog Posts
You might find a blog post that just seems unusable. Should you delete your old content? It depends. If it’s completely outdated, of low quality, and irrelevant to any valuable keywords for your website, it’s better to remove it.
Once you decide to delete the post, don’t forget to set up a 301 redirect to a related post or page, or to your homepage.
Promote Old Blog Posts
Sometimes all your content needs is a bit of promotion to start ranking and getting traffic again. Share it on your social media, link to it from a new post – do something to get it discoverable again to your audience. This can give it the boost it needs to attract organic links too.
Which Blog Posts Should You Update?
Deciding when to update or rewrite blog posts is a decision that relies on one important thing: a content audit.
Use your Google Analytics to find out which blog posts used to drive tons of traffic, but no longer have the same reach. You can also use Google Search Console to find out which of your blog posts have lost visibility in comparison to previous months. I have a guide on website analysis using Google Analytics and Google Search Console you can follow.
If you use keyword tracking tools like SE Ranking, you can also use the data it provides to come up with a list of blog posts that have dropped in the rankings.
Make data-driven decisions to identify which blog posts would benefit from these updates – i.e., which ones still have the chance to recover their keyword rankings and organic traffic.
With Google’s helpful content update, which emphasizes better user experiences, it’s crucial to ensure your content remains relevant, valuable, and up-to-date.
How To Update Old Blog Posts for SEO
Updating articles can be an involved process. Here are some tips and tactics to help you get it right.
Author’s Note: I have a Comprehensive On-Page SEO Checklist you might also be interested in following while you’re doing your content audit.
Conduct New Keyword Research
Updating your post without any guide won’t get you far. Always do your keyword research to understand how users are searching for your given topic.
Proper research can also show you relevant questions and sections that can be added to the blog post you’re updating or rewriting. Make sure to take a look at the People Also Ask (PAA) section that shows up when you search for your target keyword. Check out other websites like Answer The Public, Reddit, and Quora to see what users are looking for too.
Look for New Ranking Opportunities
When trying to revive an old blog post for SEO, keep an eye out for new SEO opportunities (e.g., AI Overview, featured snippets, and related search terms) that didn’t exist when you first wrote your blog post. Some of these features can be targeted by the new content you will add to your post, if you write with the aim to be eligible for it.
Rewrite Headlines and Meta Tags
If you want to attract new readers, consider updating your headlines and meta tags.
Your headlines and meta tags should fulfill these three things:
- Reflect the rewritten and new content you’ve added to the blog post.
- Be optimized for the new keywords it’s targeting (if any).
- Appeal to your target audience – who may have changed tastes from when the blog post was originally made.
Remember that your meta tags in particular act like a brief advertisement for your blog post, since this is what the user first sees when your blog post is shown in the search results page.
Take a look at your blog post’s click-through rate on Google Search Console – if it falls below 2%, it’s definitely time for new meta tags.
Replace Outdated Information and Statistics
Updating blog content with current studies and statistics enhances the relevance and credibility of your post. By providing up-to-date information, you help your audience make better, well-informed decisions, while also showing that your content is trustworthy.
Tighten or Expand Ideas
Your old content might be too short to provide real value to users – or you might have rambled on and on in your post. It’s important to evaluate whether you need to make your content more concise, or if you need to elaborate more.
Keep the following tips in mind as you refine your blog post’s ideas:
- Evaluate Helpfulness: Measure how well your content addresses your readers’ pain points. Aim to follow the E-E-A-T model (Experience, Expertise, Authoritativeness, Trustworthiness).
- Identify Missing Context: Consider whether your content needs more detail or clarification. View it from your audience’s perspective and ask if the information is complete, or if more information is needed.
- Interview Experts: Speak with industry experts or thought leaders to get fresh insights. This will help support your writing, and provide unique points that enhance the value of your content.
- Use Better Examples: Examples help simplify complex concepts. Add new examples or improve existing ones to strengthen your points.
- Add New Sections if Needed: If your content lacks depth or misses a key point, add new sections to cover these areas more thoroughly.
- Remove Fluff: Every sentence should contribute to the overall narrative. Eliminate unnecessary content to make your post more concise.
- Revise Listicles: Update listicle items based on SEO recommendations and content quality. Add or remove headings to stay competitive with higher-ranking posts.
Improve Visuals and Other Media
No doubt that there are tons of old graphics and photos in your blog posts that can be improved with the tools we have today. Make sure all of the visuals used in your content are appealing and high quality.
Update Internal and External Links
Are your internal and external links up to date? They need to be for your SEO and user experience. Outdated links can lead to broken pages or irrelevant content, frustrating readers and hurting your site’s performance.
You need to check for any broken links on your old blog posts, and update them ASAP. Updating your old blog posts can also lead to new opportunities to link internally to other blog posts and pages, which may not have been available when the post was originally published.
Optimize for Conversions
When updating content, the ultimate goal is often to increase conversions. However, your conversion goals may have changed over the years.
So here’s what you need to check in your updated blog post. First, does the call-to-action (CTA) still link to the products or services you want to promote? If not, update it to direct readers to the current solution or offer.
Second, consider where you can use different conversion strategies. Don’t just add a CTA at the end of the post.
Last, make sure that the blog post leverages product-led content. It’s going to help you mention your products and services in a way that feels natural, without being too pushy. Being subtle can be a high ROI tactic for updated posts.
Key Takeaway
Reviving old blog articles for SEO is a powerful strategy that can breathe new life into your content and boost your website’s visibility. Instead of solely focusing on creating new posts, taking the time to refresh existing content can yield impressive results, both in terms of traffic and conversions.
By implementing these strategies, you can transform old blog posts into valuable resources that attract new readers and retain existing ones. So, roll up your sleeves, dive into your archives, and start updating your content today—your audience and search rankings will thank you!
SEO
How Compression Can Be Used To Detect Low Quality Pages
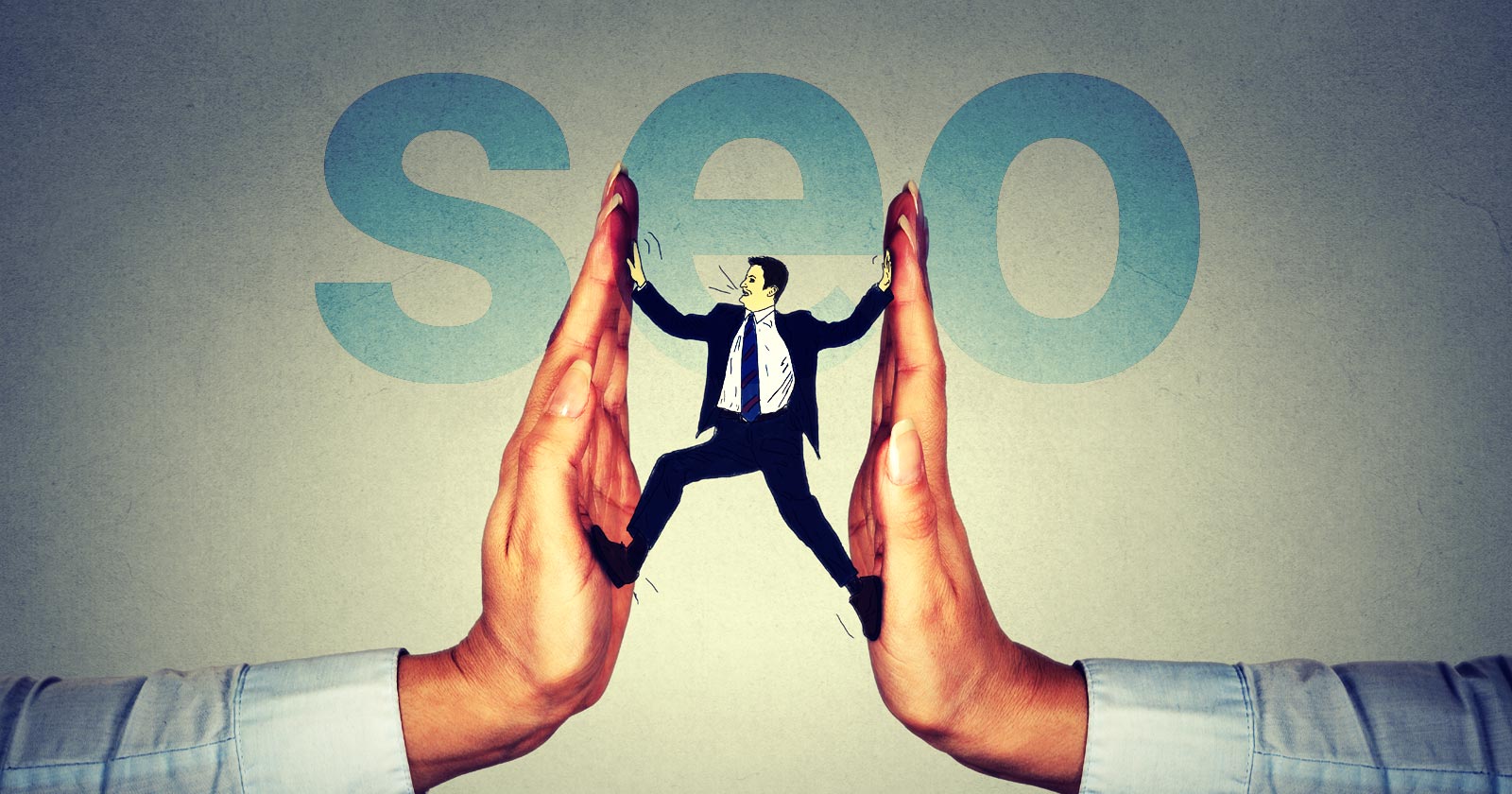
The concept of Compressibility as a quality signal is not widely known, but SEOs should be aware of it. Search engines can use web page compressibility to identify duplicate pages, doorway pages with similar content, and pages with repetitive keywords, making it useful knowledge for SEO.
Although the following research paper demonstrates a successful use of on-page features for detecting spam, the deliberate lack of transparency by search engines makes it difficult to say with certainty if search engines are applying this or similar techniques.
What Is Compressibility?
In computing, compressibility refers to how much a file (data) can be reduced in size while retaining essential information, typically to maximize storage space or to allow more data to be transmitted over the Internet.
TL/DR Of Compression
Compression replaces repeated words and phrases with shorter references, reducing the file size by significant margins. Search engines typically compress indexed web pages to maximize storage space, reduce bandwidth, and improve retrieval speed, among other reasons.
This is a simplified explanation of how compression works:
- Identify Patterns:
A compression algorithm scans the text to find repeated words, patterns and phrases - Shorter Codes Take Up Less Space:
The codes and symbols use less storage space then the original words and phrases, which results in a smaller file size. - Shorter References Use Less Bits:
The “code” that essentially symbolizes the replaced words and phrases uses less data than the originals.
A bonus effect of using compression is that it can also be used to identify duplicate pages, doorway pages with similar content, and pages with repetitive keywords.
Research Paper About Detecting Spam
This research paper is significant because it was authored by distinguished computer scientists known for breakthroughs in AI, distributed computing, information retrieval, and other fields.
Marc Najork
One of the co-authors of the research paper is Marc Najork, a prominent research scientist who currently holds the title of Distinguished Research Scientist at Google DeepMind. He’s a co-author of the papers for TW-BERT, has contributed research for increasing the accuracy of using implicit user feedback like clicks, and worked on creating improved AI-based information retrieval (DSI++: Updating Transformer Memory with New Documents), among many other major breakthroughs in information retrieval.
Dennis Fetterly
Another of the co-authors is Dennis Fetterly, currently a software engineer at Google. He is listed as a co-inventor in a patent for a ranking algorithm that uses links, and is known for his research in distributed computing and information retrieval.
Those are just two of the distinguished researchers listed as co-authors of the 2006 Microsoft research paper about identifying spam through on-page content features. Among the several on-page content features the research paper analyzes is compressibility, which they discovered can be used as a classifier for indicating that a web page is spammy.
Detecting Spam Web Pages Through Content Analysis
Although the research paper was authored in 2006, its findings remain relevant to today.
Then, as now, people attempted to rank hundreds or thousands of location-based web pages that were essentially duplicate content aside from city, region, or state names. Then, as now, SEOs often created web pages for search engines by excessively repeating keywords within titles, meta descriptions, headings, internal anchor text, and within the content to improve rankings.
Section 4.6 of the research paper explains:
“Some search engines give higher weight to pages containing the query keywords several times. For example, for a given query term, a page that contains it ten times may be higher ranked than a page that contains it only once. To take advantage of such engines, some spam pages replicate their content several times in an attempt to rank higher.”
The research paper explains that search engines compress web pages and use the compressed version to reference the original web page. They note that excessive amounts of redundant words results in a higher level of compressibility. So they set about testing if there’s a correlation between a high level of compressibility and spam.
They write:
“Our approach in this section to locating redundant content within a page is to compress the page; to save space and disk time, search engines often compress web pages after indexing them, but before adding them to a page cache.
…We measure the redundancy of web pages by the compression ratio, the size of the uncompressed page divided by the size of the compressed page. We used GZIP …to compress pages, a fast and effective compression algorithm.”
High Compressibility Correlates To Spam
The results of the research showed that web pages with at least a compression ratio of 4.0 tended to be low quality web pages, spam. However, the highest rates of compressibility became less consistent because there were fewer data points, making it harder to interpret.
Figure 9: Prevalence of spam relative to compressibility of page.
The researchers concluded:
“70% of all sampled pages with a compression ratio of at least 4.0 were judged to be spam.”
But they also discovered that using the compression ratio by itself still resulted in false positives, where non-spam pages were incorrectly identified as spam:
“The compression ratio heuristic described in Section 4.6 fared best, correctly identifying 660 (27.9%) of the spam pages in our collection, while misidentifying 2, 068 (12.0%) of all judged pages.
Using all of the aforementioned features, the classification accuracy after the ten-fold cross validation process is encouraging:
95.4% of our judged pages were classified correctly, while 4.6% were classified incorrectly.
More specifically, for the spam class 1, 940 out of the 2, 364 pages, were classified correctly. For the non-spam class, 14, 440 out of the 14,804 pages were classified correctly. Consequently, 788 pages were classified incorrectly.”
The next section describes an interesting discovery about how to increase the accuracy of using on-page signals for identifying spam.
Insight Into Quality Rankings
The research paper examined multiple on-page signals, including compressibility. They discovered that each individual signal (classifier) was able to find some spam but that relying on any one signal on its own resulted in flagging non-spam pages for spam, which are commonly referred to as false positive.
The researchers made an important discovery that everyone interested in SEO should know, which is that using multiple classifiers increased the accuracy of detecting spam and decreased the likelihood of false positives. Just as important, the compressibility signal only identifies one kind of spam but not the full range of spam.
The takeaway is that compressibility is a good way to identify one kind of spam but there are other kinds of spam that aren’t caught with this one signal. Other kinds of spam were not caught with the compressibility signal.
This is the part that every SEO and publisher should be aware of:
“In the previous section, we presented a number of heuristics for assaying spam web pages. That is, we measured several characteristics of web pages, and found ranges of those characteristics which correlated with a page being spam. Nevertheless, when used individually, no technique uncovers most of the spam in our data set without flagging many non-spam pages as spam.
For example, considering the compression ratio heuristic described in Section 4.6, one of our most promising methods, the average probability of spam for ratios of 4.2 and higher is 72%. But only about 1.5% of all pages fall in this range. This number is far below the 13.8% of spam pages that we identified in our data set.”
So, even though compressibility was one of the better signals for identifying spam, it still was unable to uncover the full range of spam within the dataset the researchers used to test the signals.
Combining Multiple Signals
The above results indicated that individual signals of low quality are less accurate. So they tested using multiple signals. What they discovered was that combining multiple on-page signals for detecting spam resulted in a better accuracy rate with less pages misclassified as spam.
The researchers explained that they tested the use of multiple signals:
“One way of combining our heuristic methods is to view the spam detection problem as a classification problem. In this case, we want to create a classification model (or classifier) which, given a web page, will use the page’s features jointly in order to (correctly, we hope) classify it in one of two classes: spam and non-spam.”
These are their conclusions about using multiple signals:
“We have studied various aspects of content-based spam on the web using a real-world data set from the MSNSearch crawler. We have presented a number of heuristic methods for detecting content based spam. Some of our spam detection methods are more effective than others, however when used in isolation our methods may not identify all of the spam pages. For this reason, we combined our spam-detection methods to create a highly accurate C4.5 classifier. Our classifier can correctly identify 86.2% of all spam pages, while flagging very few legitimate pages as spam.”
Key Insight:
Misidentifying “very few legitimate pages as spam” was a significant breakthrough. The important insight that everyone involved with SEO should take away from this is that one signal by itself can result in false positives. Using multiple signals increases the accuracy.
What this means is that SEO tests of isolated ranking or quality signals will not yield reliable results that can be trusted for making strategy or business decisions.
Takeaways
We don’t know for certain if compressibility is used at the search engines but it’s an easy to use signal that combined with others could be used to catch simple kinds of spam like thousands of city name doorway pages with similar content. Yet even if the search engines don’t use this signal, it does show how easy it is to catch that kind of search engine manipulation and that it’s something search engines are well able to handle today.
Here are the key points of this article to keep in mind:
- Doorway pages with duplicate content is easy to catch because they compress at a higher ratio than normal web pages.
- Groups of web pages with a compression ratio above 4.0 were predominantly spam.
- Negative quality signals used by themselves to catch spam can lead to false positives.
- In this particular test, they discovered that on-page negative quality signals only catch specific types of spam.
- When used alone, the compressibility signal only catches redundancy-type spam, fails to detect other forms of spam, and leads to false positives.
- Combing quality signals improves spam detection accuracy and reduces false positives.
- Search engines today have a higher accuracy of spam detection with the use of AI like Spam Brain.
Read the research paper, which is linked from the Google Scholar page of Marc Najork:
Detecting spam web pages through content analysis
Featured Image by Shutterstock/pathdoc