MARKETING
How to build a basic genAI strategy for your marketing organization
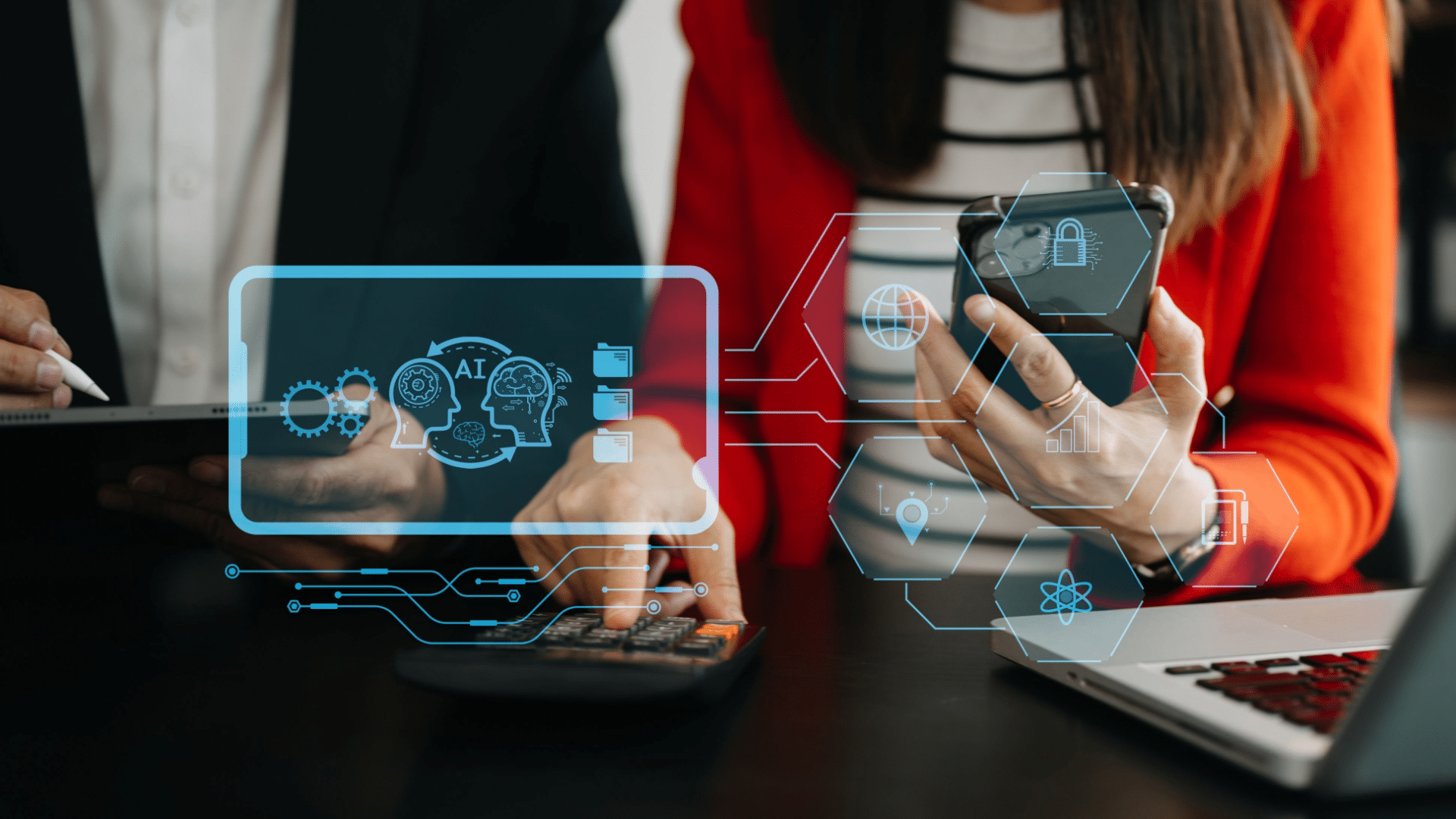
A few days ago, I joined a virtual holiday happy hour event with my best friend from college, Will, and our close mutual friend, Erin. Erin is a seasoned PR veteran and communications professor and I often invite her into my University of Oregon classes as a guest lecturer. As we were toasting the holidays, the conversation moved toward AI and the impact it would have on the new year.
My friends shared that I was one of the only professionals in their network that had a positive view of AI and had embraced using it at work. I was pleasantly surprised to hear that I was offering up a unique perspective and happily shared the many ways I had been using generative AI for marketing. Erin then asked me a great question, which was: “How do you get started bringing such a disruptive tool into an organization?”
So — Erin and Will, my loves — this article is for you and for anyone looking to be a champion for AI at work. This article will help you:
- Better understand how AI can help you and your team.
- Build a basic AI strategy.
- Bring generative AI into your organization.
However, this article is not:
- A risk assessment analysis.
- Legal advice on how to navigate risk.
- Governance strategies or guardrails.
- A list of the best AI tools (they will change over time).
There are some great resources available for those important topics, including the National Institute of Standards and Technology AI risk management framework, a great starting point if your role is responsible for AI governance.
Building a basic AI strategy
As with any project I take on, whether personal or professional, I always start with strategy. The most basic building blocks of an effective strategy are an overall objective; a target audience and audience profiles; budget; and KPI’s to help you measure the outcome of your strategic planning. So, let’s start with these three basic questions:
- Identify the opportunity and objective: Which marketing tasks could benefit from the help of AI tools?
- Identify your target user base: Which roles within my organization complete these tasks?
- Define KPI’s: How will we measure the success of our AI tools to positively impact the completion of these tasks?
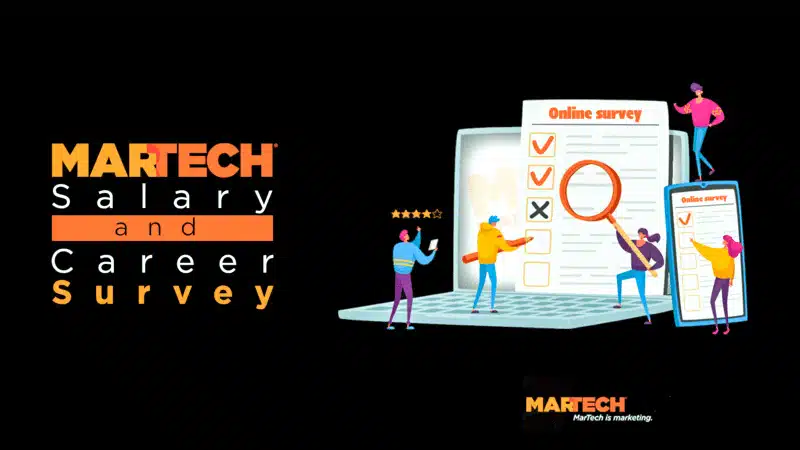
Take Martech’s 2024 Salary and Career Survey
From AI to layoffs, it’s been quite a year. We’d like to know how it’s been for you. Please take this short survey so we can have your input on the state of martech salaries and careers.
Identify the opportunity
This first step is to identify the areas and specific marketing tasks that would benefit from generative AI. This includes assessing content creation, data analysis, customer engagement and campaign optimization tasks. I recommend a good old-fashioned brainstorming session, where you list all tasks that are currently part of your team’s workflow. You may want to review the previous posts in my Decoding AI for Marketing series, as they are chock full of creative marketing applications for generative AI tools.
For additional inspiration, I have crafted a blog post and infographic that lists out seven marketing categories or pillars and the specific marketing tasks associated with each one that can benefit from AI.
The pillars include:
- Content creation and management.
- Data analysis and insights.
- Customer engagement and experience.
- Campaign optimization and management.
- Product marketing and strategy.
- Digital marketing and ecommerce.
- Event marketing and PR.
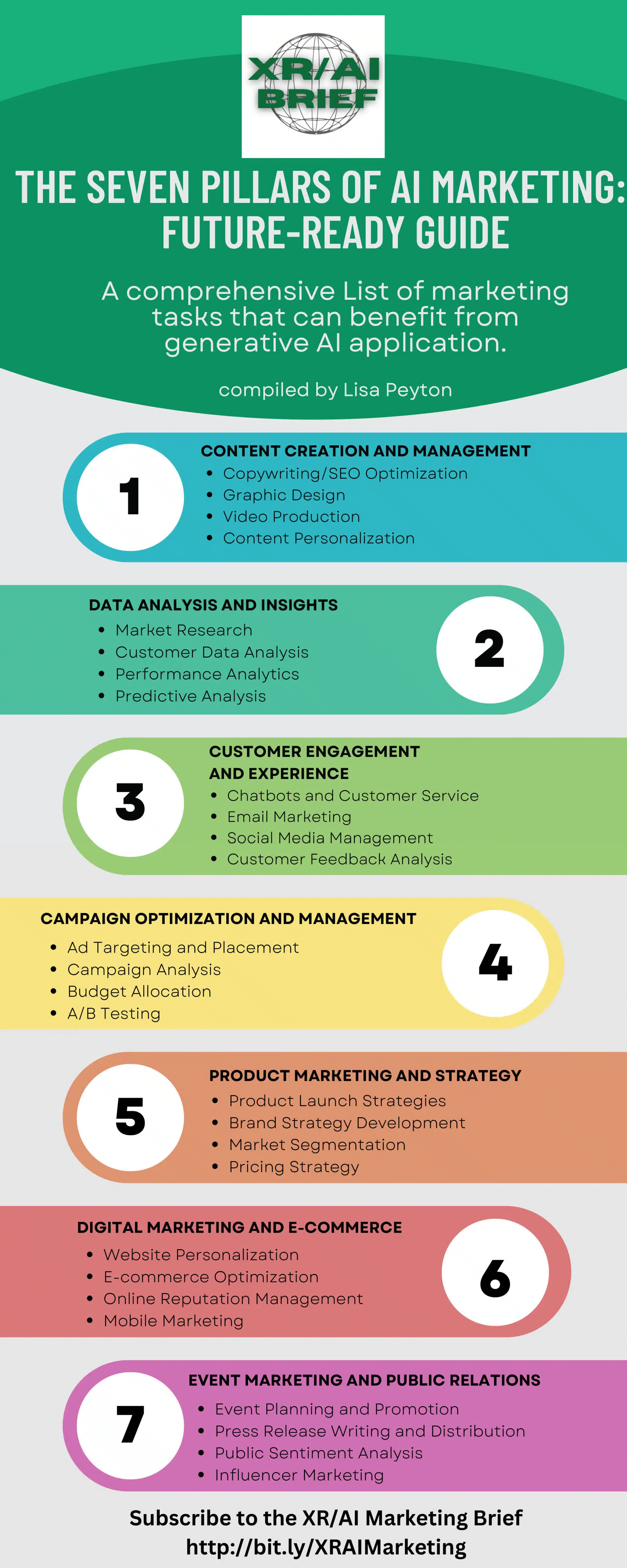
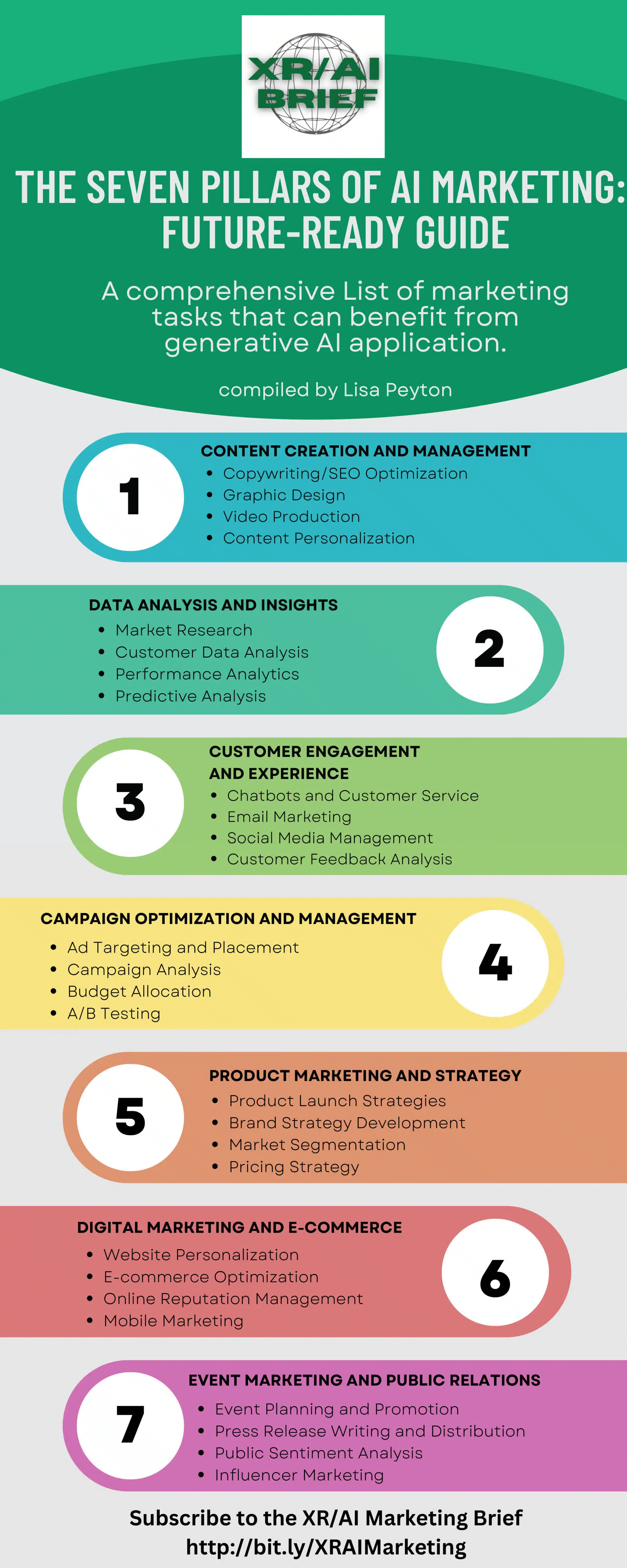
Target users
Once you have identified the specific tasks and objectives of your AI strategy, you can then work to identify the roles within your organization that will need access to AI tools. Depending on the size of your company, you may have hundreds or even thousands of individuals within the marketing department that manage very specific tactics. As one of my Intel colleagues loved to say, you do not want to “boil the ocean.” So a small pilot group that is focused on one specific area would be a good place to start.
If you work for a startup or SMB, your marketing team is likely to be lean and nimble, with only a handful of roles dedicated across many marketing channels. Identifying key contributors responsible for the tasks you identified in step 1, who are eager and willing to evaluate these new tools, will help ensure your plan is successful.
You might also want to conduct a skill gap analysis, assessing the current skill levels of the team regarding AI technology and identifying areas where training or hiring may be necessary.
KPIs for your AI toolset
The next step in this basic strategic planning process is to determine how you will measure the outcome and performance of your AI toolset. These metrics will be specific to the tasks you identified and could help measure increased productivity, creativity, quality of work, customer engagement and many other potential outcomes.
The most important ingredient of this step is for all stakeholders to align on which measurements will determine the effectiveness of AI-powered outcomes.
Budget and human resources
Determining the budget, time, and human resources required for your AI implementation is an essential part of the planning process. This includes software costs, training expenses and potential hiring. I recommend starting with a small pilot, proving the ROI of a small initial investment, and then scaling more broadly across the organization.
To help you organize the output of this basic strategy, I have crafted an AI Strategy Worksheet or template, that you can use as a collaboration tool when brainstorming with your team. I recommend loading this up in Miro, an interactive whiteboard tool, so that remote teams can ideate together and easily save everyone’s input.
Next steps
Constructing this basic AI strategy will set you and your team up for a successful AI implementation. Stay tuned for additional articles within the Decoding AI series that discuss more details on these next steps:
- Building an AI toolbox — tool selection
- AI communications and education — training and adoption
- KPI’s and measuring outcomes
As always, I am available for any questions or help you may need bringing this into your organization. I offer up some great free resources including my XR/AI Marketing Brief — a monthly newsletter — and the AI Marketing Revolution Challenge Video Series.
I would love to connect on Linkedin and perhaps meet at my next live XR Pub Crawl for Marketing on January 19 at noon PT. Happy new year.
Get MarTech! Daily. Free. In your inbox.
Opinions expressed in this article are those of the guest author and not necessarily MarTech. Staff authors are listed here.