FLAN: Google Research Develops Better Machine Learning
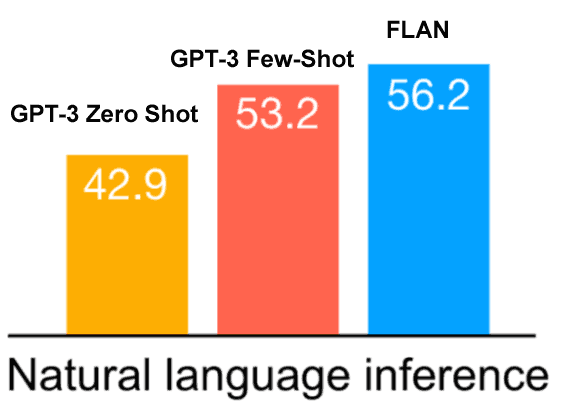
Google recently published research on a technique to train a model to be able to solve natural language processing problems in a way that can be applied to multiple tasks. Rather than train a model to solve one kind of problem, this approach teaches it how to solve a wide range of problems, making it more efficient and advancing the state of the art.
Google Doesn’t Use All Research In Their Algorithms
Google’s official statement on research papers is that just because it publishes an algorithm doesn’t mean that it’s in use at Google Search.
Nothing in the research paper says it should be used in search. But what makes this research of interest is that it advances the state of the art and improves on current technology.
The Value Of Being Aware of Technology
People who don’t know how search engines work can end up understanding it in terms that are pure speculation.
That’s how the search industry ended up with false ideas such as “LSI Keywords” and nonsensical strategies such as trying to beat the competition by creating content that is ten times better (or simply bigger) than the competitor’s content, with zero consideration of what users might need and require.
The value in knowing about these algorithms and techniques is of being aware of the general contours of what goes on in search engines so that one does not make the error of underestimating what search engines are capable of.
The Problem That FLAN Solves
The main problem this technique solves is of enabling a machine to use its vast amount of knowledge to solve real-world tasks.
The approach teaches the machine how to generalize problem solving in order to be able to solve unseen problems.
It does this by feeding instructions to solve specific problems then generalizing those instructions in order to solve other problems.
The researchers state:
“The model is fine-tuned on disparate sets of instructions and generalizes to unseen instructions. As more types of tasks are added to the fine-tuning data model performance improves.
…We show that by training a model on these instructions it not only becomes good at solving the kinds of instructions it has seen during training but becomes good at following instructions in general.”
The research paper cites a current popular technique called “zero-shot or few-shot prompting” that trains a machine to solve a specific language problem and describes the shortcoming in this technique.
Referencing the zero shot/few shot prompting technique:
“This technique formulates a task based on text that a language model might have seen during training, where then the language model generates the answer by completing the text.
For instance, to classify the sentiment of a movie review, a language model might be given the sentence, “The movie review ‘best RomCom since Pretty Woman’ is _” and be asked to complete the sentence with either the word “positive” or “negative”.”
The researchers note that the zero shot approach performs well but that the performance has to be measured against tasks that the model has previously seen before.
The researchers write:
“…it requires careful prompt engineering to design tasks to look like data that the model has seen during training…”
And that kind of shortcoming is what FLAN solves. Because the training instructions are generalized the model is able to solve more problems including solving tasks it has not previously been trained on.
Could This Technique Be Used By Google?
Google rarely discusses specific research papers and whether or not what’s described is in use. Google’s official stance on research papers that it publishes many of them and that they don’t necessarily end up in their search ranking algorithm.
Google is generally opaque about what’s in their algorithms and rightly so.
Even when it announces new technologies Google tends to give them names that do not correspond with published research papers. For example, names like Neural Matching and Rank Brain don’t correspond with specific research papers.
It’s important to review the success of the research because some research falls short of their goals and don’t perform as well as current state of the art in techniques and algorithms.
Those research papers that fall short can more or less be ignored but they’re good to know about.
The research papers that are of most value to the search marketing community are those that are successful and perform significantly better than the current state of the art.
And that is the case with FLAN.
FLAN performs better than other techniques and for that reason FLAN is something to be aware of.
The researchers noted:
“We evaluated FLAN on 25 tasks and found that it improves over zero-shot prompting on all but four of them. We found that our results are better than zero-shot GPT-3 on 20 of 25 tasks, and better than even few-shot GPT-3 on some tasks.”
Natural Language Inference
Natural Language Inference Task is one in which the machine has to determine if a given premise is true, false or undetermined/neutral (neither true or false).
Natural Language Inference Performance of FLAN
Reading Comprehension
This is a task of answering a question based on content in a document.
Reading Comprehension Performance of FLAN
Closed-book QA
This is the ability to answer questions with factual data, which tests the ability to match known facts with the questions. An example is answering questions like what color is the sky or who was the first president of the United States.
Closed Book QA Performance of FLAN
Is Google Using FLAN?
As previously stated, Google does not generally confirm whether they’re using a specific algorithm or technique.
However, the fact that this particular technique moves the state of the art forward could mean that it’s not unreasonable to speculate that some form of it could be integrated into Google’s algorithm, improving its ability to answer search queries.
This research was published on October 28, 2021.
Could some of this have been incorporated into the recent Core Algorithm Update?
Core algorithm updates are generally focused on understanding queries and web pages better and providing better answers.
One can only speculate as Google rarely shares specifics, especially with regard to core algorithm updates.
Citation
Introducing FLAN: More generalizable Language Models with Instruction Fine-Tuning
Image by Shutterstock
You must be logged in to post a comment Login