SEO
How To Do A Sitemap Audit For Better Indexing & Crawling Via Python
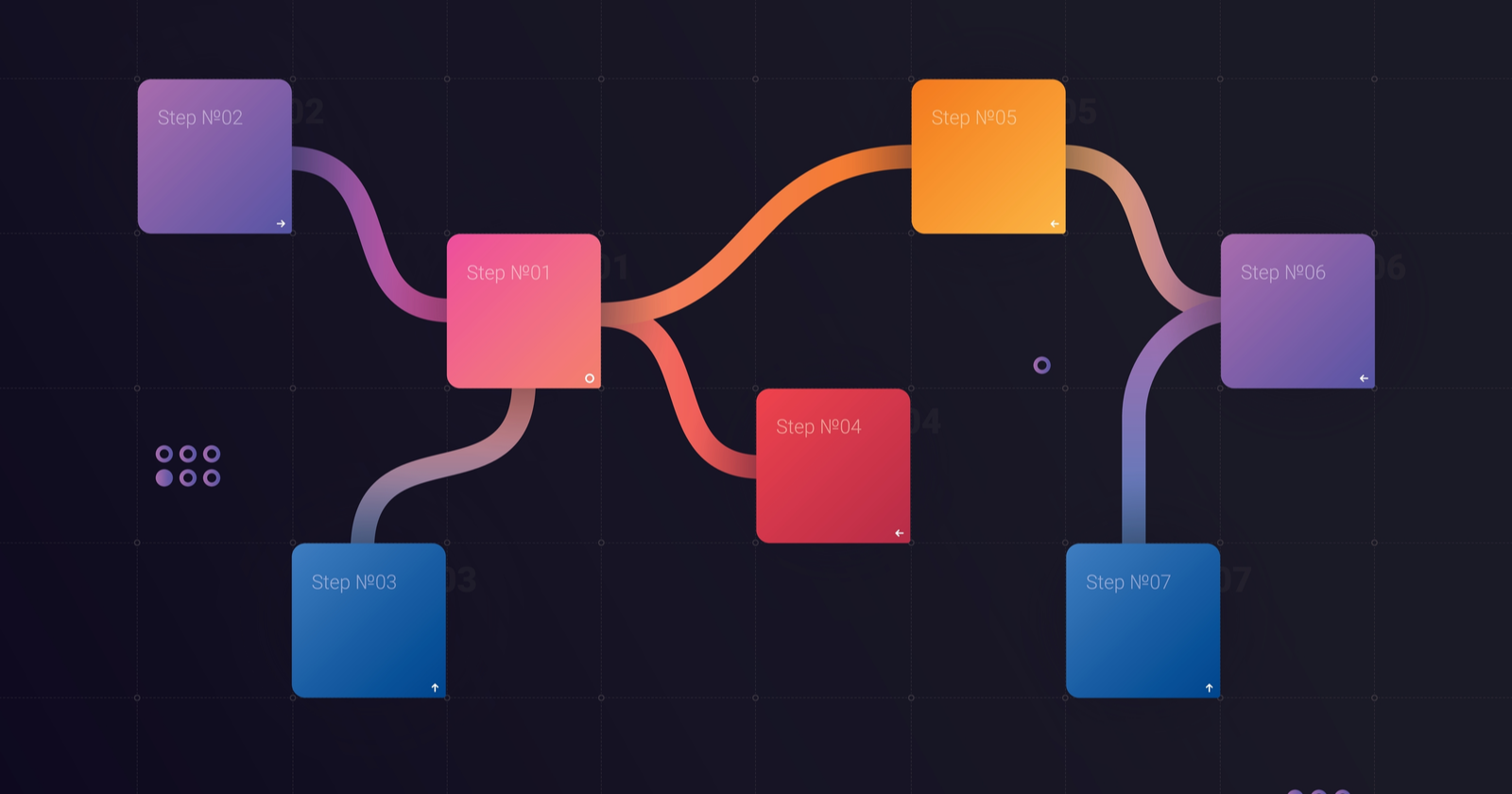
Sitemap auditing involves syntax, crawlability, and indexation checks for the URLs and tags in your sitemap files.
A sitemap file contains the URLs to index with further information regarding the last modification date, priority of the URL, images, videos on the URL, and other language alternates of the URL, along with the change frequency.
Sitemap index files can involve millions of URLs, even if a single sitemap can only involve 50,000 URLs at the top.
Auditing these URLs for better indexation and crawling might take time.
But with the help of Python and SEO automation, it is possible to audit millions of URLs within the sitemaps.
What Do You Need To Perform A Sitemap Audit With Python?
To understand the Python Sitemap Audit process, you’ll need:
- A fundamental understanding of technical SEO and sitemap XML files.
- Working knowledge of Python and sitemap XML syntax.
- The ability to work with Python Libraries, Pandas, Advertools, LXML, Requests, and XPath Selectors.
Which URLs Should Be In The Sitemap?
A healthy sitemap XML sitemap file should include the following criteria:
- All URLs should have a 200 Status Code.
- All URLs should be self-canonical.
- URLs should be open to being indexed and crawled.
- URLs shouldn’t be duplicated.
- URLs shouldn’t be soft 404s.
- The sitemap should have a proper XML syntax.
- The URLs in the sitemap should have an aligning canonical with Open Graph and Twitter Card URLs.
- The sitemap should have less than 50.000 URLs and a 50 MB size.
What Are The Benefits Of A Healthy XML Sitemap File?
Smaller sitemaps are better than larger sitemaps for faster indexation. This is particularly important in News SEO, as smaller sitemaps help for increasing the overall valid indexed URL count.
Differentiate frequently updated and static content URLs from each other to provide a better crawling distribution among the URLs.
Using the “lastmod” date in an honest way that aligns with the actual publication or update date helps a search engine to trust the date of the latest publication.
While performing the Sitemap Audit for better indexing, crawling, and search engine communication with Python, the criteria above are followed.
An Important Note…
When it comes to a sitemap’s nature and audit, Google and Microsoft Bing don’t use “changefreq” for changing frequency of the URLs and “priority” to understand the prominence of a URL. In fact, they call it a “bag of noise.”
However, Yandex and Baidu use all these tags to understand the website’s characteristics.
A 16-Step Sitemap Audit For SEO With Python
A sitemap audit can involve content categorization, site-tree, or topicality and content characteristics.
However, a sitemap audit for better indexing and crawlability mainly involves technical SEO rather than content characteristics.
In this step-by-step sitemap audit process, we’ll use Python to tackle the technical aspects of sitemap auditing millions of URLs.
1. Import The Python Libraries For Your Sitemap Audit
The following code block is to import the necessary Python Libraries for the Sitemap XML File audit.
import advertools as adv import pandas as pd from lxml import etree from IPython.core.display import display, HTML display(HTML("<style>.container { width:100% !important; }</style>"))
Here’s what you need to know about this code block:
- Advertools is necessary for taking the URLs from the sitemap file and making a request for taking their content or the response status codes.
- “Pandas” is necessary for aggregating and manipulating the data.
- Plotly is necessary for the visualization of the sitemap audit output.
- LXML is necessary for the syntax audit of the sitemap XML file.
- IPython is optional to expand the output cells of Jupyter Notebook to 100% width.
2. Take All Of The URLs From The Sitemap
Millions of URLs can be taken into a Pandas data frame with Advertools, as shown below.
sitemap_url = "https://www.complaintsboard.com/sitemap.xml" sitemap = adv.sitemap_to_df(sitemap_url) sitemap.to_csv("sitemap.csv") sitemap_df = pd.read_csv("sitemap.csv", index_col=False) sitemap_df.drop(columns=["Unnamed: 0"], inplace=True) sitemap_df
Above, the Complaintsboard.com sitemap has been taken into a Pandas data frame, and you can see the output below.
-
A General Sitemap URL Extraction with Sitemap Tags with Python is above.
In total, we have 245,691 URLs in the sitemap index file of Complaintsboard.com.
The website uses “changefreq,” “lastmod,” and “priority” with an inconsistency.
3. Check Tag Usage Within The Sitemap XML File
To understand which tags are used or not within the Sitemap XML file, use the function below.
def check_sitemap_tag_usage(sitemap): lastmod = sitemap["lastmod"].isna().value_counts() priority = sitemap["priority"].isna().value_counts() changefreq = sitemap["changefreq"].isna().value_counts() lastmod_perc = sitemap["lastmod"].isna().value_counts(normalize = True) * 100 priority_perc = sitemap["priority"].isna().value_counts(normalize = True) * 100 changefreq_perc = sitemap["changefreq"].isna().value_counts(normalize = True) * 100 sitemap_tag_usage_df = pd.DataFrame(data={"lastmod":lastmod, "priority":priority, "changefreq":changefreq, "lastmod_perc": lastmod_perc, "priority_perc": priority_perc, "changefreq_perc": changefreq_perc}) return sitemap_tag_usage_df.astype(int)
The function check_sitemap_tag_usage is a data frame constructor based on the usage of the sitemap tags.
It takes the “lastmod,” “priority,” and “changefreq” columns by implementing “isna()” and “value_counts()” methods via “pd.DataFrame”.
Below, you can see the output.

The data frame above shows that 96,840 of the URLs do not have the Lastmod tag, which is equal to 39% of the total URL count of the sitemap file.
The same usage percentage is 19% for the “priority” and the “changefreq” within the sitemap XML file.
There are three main content freshness signals from a website.
These are dates from a web page (visible to the user), structured data (invisible to the user), “lastmod” in the sitemap.
If these dates are not consistent with each other, search engines can ignore the dates on the websites to see their freshness signals.
4. Audit The Site-tree And URL Structure Of The Website
Understanding the most important or crowded URL Path is necessary to weigh the website’s SEO efforts or technical SEO Audits.
A single improvement for Technical SEO can benefit thousands of URLs simultaneously, which creates a cost-effective and budget-friendly SEO strategy.
URL Structure Understanding mainly focuses on the website’s more prominent sections and content network analysis understanding.
To create a URL Tree Dataframe from a website’s URLs from the sitemap, use the following code block.
sitemap_url_df = adv.url_to_df(sitemap_df["loc"]) sitemap_url_df
With the help of “urllib” or the “advertools” as above, you can easily parse the URLs within the sitemap into a data frame.
-
Creating a URL Tree with URLLib or Advertools is easy.
- Checking the URL breakdowns helps to understand the overall information tree of a website.
The data frame above contains the “scheme,” “netloc,” “path,” and every “/” breakdown within the URLs as a “dir” which represents the directory.
Auditing the URL structure of the website is prominent for two objectives.
These are checking whether all URLs have “HTTPS” and understanding the content network of the website.
Content analysis with sitemap files is not the topic of the “Indexing and Crawling” directly, thus at the end of the article, we will talk about it slightly.
Check the next section to see the SSL Usage on Sitemap URLs.
5. Check The HTTPS Usage On The URLs Within Sitemap
Use the following code block to check the HTTP Usage ratio for the URLs within the Sitemap.
sitemap_url_df["scheme"].value_counts().to_frame()
The code block above uses a simple data filtration for the “scheme” column which contains the URLs’ HTTPS Protocol information.
using the “value_counts” we see that all URLs are on the HTTPS.

6. Check The Robots.txt Disallow Commands For Crawlability
The structure of URLs within the sitemap is beneficial to see whether there is a situation for “submitted but disallowed”.
To see whether there is a robots.txt file of the website, use the code block below.
import requests r = requests.get("https://www.complaintsboard.com/robots.txt") R.status_code 200
Simply, we send a “get request” to the robots.txt URL.
If the response status code is 200, it means there is a robots.txt file for the user-agent-based crawling control.
After checking the “robots.txt” existence, we can use the “adv.robotstxt_test” method for bulk robots.txt audit for crawlability of the URLs in the sitemap.
sitemap_df_robotstxt_check = adv.robotstxt_test("https://www.complaintsboard.com/robots.txt", urls=sitemap_df["loc"], user_agents=["*"]) sitemap_df_robotstxt_check["can_fetch"].value_counts()
We have created a new variable called “sitemap_df_robotstxt_check”, and assigned the output of the “robotstxt_test” method.
We have used the URLs within the sitemap with the “sitemap_df[“loc”]”.
We have performed the audit for all of the user-agents via the “user_agents = [“*”]” parameter and value pair.
You can see the result below.
True 245690 False 1 Name: can_fetch, dtype: int64
It shows that there is one URL that is disallowed but submitted.
We can filter the specific URL as below.
pd.set_option("display.max_colwidth",255) sitemap_df_robotstxt_check[sitemap_df_robotstxt_check["can_fetch"] == False]
We have used “set_option” to expand all of the values within the “url_path” section.
-
A URL appears as disallowed but submitted via a sitemap as in Google Search Console Coverage Reports.
- We see that a “profile” page has been disallowed and submitted.
Later, the same control can be done for further examinations such as “disallowed but internally linked”.
But, to do that, we need to crawl at least 3 million URLs from ComplaintsBoard.com, and it can be an entirely new guide.
Some website URLs do not have a proper “directory hierarchy”, which can make the analysis of the URLs, in terms of content network characteristics, harder.
Complaintsboard.com doesn’t use a proper URL structure and taxonomy, so analyzing the website structure is not easy for an SEO or Search Engine.
But the most used words within the URLs or the content update frequency can signal which topic the company actually weighs on.
Since we focus on “technical aspects” in this tutorial, you can read the Sitemap Content Audit here.
7. Check The Status Code Of The Sitemap URLs With Python
Every URL within the sitemap has to have a 200 Status Code.
A crawl has to be performed to check the status codes of the URLs within the sitemap.
But, since it’s costly when you have millions of URLs to audit, we can simply use a new crawling method from Advertools.
Without taking the response body, we can crawl just the response headers of the URLs within the sitemap.
It is useful to decrease the crawl time for auditing possible robots, indexing, and canonical signals from the response headers.
To perform a response header crawl, use the “adv.crawl_headers” method.
adv.crawl_headers(sitemap_df["loc"], output_file="sitemap_df_header.jl") df_headers = pd.read_json("sitemap_df_header.jl", lines=True) df_headers["status"].value_counts()
The explanation of the code block for checking the URLs’ status codes within the Sitemap XML Files for the Technical SEO aspect can be seen below.
200 207866 404 23 Name: status, dtype: int64
It shows that the 23 URL from the sitemap is actually 404.
And, they should be removed from the sitemap.
To audit which URLs from the sitemap are 404, use the filtration method below from Pandas.
df_headers[df_headers["status"] == 404]
The result can be seen below.

8. Check The Canonicalization From Response Headers
From time to time, using canonicalization hints on the response headers is beneficial for crawling and indexing signal consolidation.
In this context, the canonical tag on the HTML and the response header has to be the same.
If there are two different canonicalization signals on a web page, the search engines can ignore both assignments.
For ComplaintsBoard.com, we don’t have a canonical response header.
- The first step is auditing whether the response header for canonical usage exists.
- The second step is comparing the response header canonical value to the HTML canonical value if it exists.
- The third step is checking whether the canonical values are self-referential.
Check the columns of the output of the header crawl to check the Canonicalization from Response Headers.
df_headers.columns
Below, you can see the columns.

If you are not familiar with the response headers, you may not know how to use canonical hints within response headers.
A response header can include the canonical hint with the “Link” value.
It is registered as “resp_headers_link” by the Advertools directly.
Another problem is that the extracted strings appear within the “<URL>;” string pattern.
It means we will use regex to extract it.
df_headers["resp_headers_link"]
You can see the result below.

The regex pattern “[^<>][a-z:/0-9-.]*” is good enough to extract the specific canonical value.
A self-canonicalization check with the response headers is below.
df_headers["response_header_canonical"] = df_headers["resp_headers_link"].str.extract(r"([^<>][a-z:/0-9-.]*)") (df_headers["response_header_canonical"] == df_headers["url"]).value_counts()
We have used two different boolean checks.
One to check whether the response header canonical hint is equal to the URL itself.
Another to see whether the status code is 200.
Since we have 404 URLs within the sitemap, their canonical value will be “NaN”.

- We have 29 outliers for Technical SEO. Every wrong signal given to the search engine for indexation or ranking will cause the dilution of the ranking signals.
To see these URLs, use the code block below.

The Canonical Values from the Response Headers can be seen above.
df_headers[(df_headers["response_header_canonical"] != df_headers["url"]) & (df_headers["status"] == 200)]
Even a single “/” in the URL can cause canonicalization conflict as appears here for the homepage.

- You can check the canonical conflict here.
If you check log files, you will see that the search engine crawls the URLs from the “Link” response headers.
Thus in technical SEO, this should be weighted.
9. Check The Indexing And Crawling Commands From Response Headers
There are 14 different X-Robots-Tag specifications for the Google search engine crawler.
The latest one is “indexifembedded” to determine the indexation amount on a web page.
The Indexing and Crawling directives can be in the form of a response header or the HTML meta tag.
This section focuses on the response header version of indexing and crawling directives.
- The first step is checking whether the X-Robots-Tag property and values exist within the HTTP Header or not.
- The second step is auditing whether it aligns itself with the HTML Meta Tag properties and values if they exist.
Use the command below yo check the X-Robots-Tag” from the response headers.
def robots_tag_checker(dataframe:pd.DataFrame): for i in df_headers: if i.__contains__("robots"): return i else: return "There is no robots tag" robots_tag_checker(df_headers) OUTPUT>>> 'There is no robots tag'
We have created a custom function to check the “X-Robots-tag” response headers from the web pages’ source code.
It appears that our test subject website doesn’t use the X-Robots-Tag.
If there would be an X-Robots-tag, the code block below should be used.
df_headers["response_header_x_robots_tag"].value_counts() df_headers[df_headers["response_header_x_robots_tag"] == "noindex"]
Check whether there is a “noindex” directive from the response headers, and filter the URLs with this indexation conflict.
In the Google Search Console Coverage Report, those appear as “Submitted marked as noindex”.
Contradicting indexing and canonicalization hints and signals might make a search engine ignore all of the signals while making the search algorithms trust less to the user-declared signals.
10. Check The Self Canonicalization Of Sitemap URLs
Every URL in the sitemap XML files should give a self-canonicalization hint.
Sitemaps should only include the canonical versions of the URLs.
The Python code block in this section is to understand whether the sitemap URLs have self-canonicalization values or not.
To check the canonicalization from the HTML Documents’ “<head>” section, crawl the websites by taking their response body.
Use the code block below.
user_agent = "Mozilla/5.0 (Linux; Android 6.0.1; Nexus 5X Build/MMB29P) AppleWebKit/537.36 (KHTML, like Gecko) Chrome/W.X.Y.Z Mobile Safari/537.36 (compatible; Googlebot/2.1; +http://www.google.com/bot.html)"
The difference between “crawl_headers” and the “crawl” is that “crawl” takes the entire response body while the “crawl_headers” is only for response headers.
adv.crawl(sitemap_df["loc"], output_file="sitemap_crawl_complaintsboard.jl", follow_links=False, custom_settings={"LOG_FILE":"sitemap_crawl_complaintsboard.log", “USER_AGENT”:user_agent})
You can check the file size differences from crawl logs to response header crawl and entire response body crawl.

From 6GB output to the 387 MB output is quite economical.
If a search engine just wants to see certain response headers and the status code, creating information on the headers would make their crawl hits more economical.
How To Deal With Large DataFrames For Reading And Aggregating Data?
This section requires dealing with the large data frames.
A computer can’t read a Pandas DataFrame from a CSV or JL file if the file size is larger than the computer’s RAM.
Thus, the “chunking” method is used.
When a website sitemap XML File contains millions of URLs, the total crawl output will be larger than tens of gigabytes.
An iteration across sitemap crawl output data frame rows is necessary.
For chunking, use the code block below.
df_iterator = pd.read_json( 'sitemap_crawl_complaintsboard.jl', chunksize=10000, lines=True) for i, df_chunk in enumerate(df_iterator): output_df = pd.DataFrame(data={"url":df_chunk["url"],"canonical":df_chunk["canonical"], "self_canonicalised":df_chunk["url"] == df_chunk["canonical"]}) mode="w" if i == 0 else 'a' header = i == 0 output_df.to_csv( "canonical_check.csv", index=False, header=header, mode=mode ) df[((df["url"] != df["canonical"]) == True) & (df["self_canonicalised"] == False) & (df["canonical"].isna() != True)]
You can see the result below.

We see that the paginated URLs from the “book” subfolder give canonical hints to the first page, which is a non-correct practice according to the Google guidelines.
11. Check The Sitemap Sizes Within Sitemap Index Files
Every Sitemap File should be less than 50 MB. Use the Python code block below in the Technical SEO with Python context to check the sitemap file size.
pd.pivot_table(sitemap_df[sitemap_df["loc"].duplicated()==True], index="sitemap")
You can see the result below.

We see that all sitemap XML files are under 50MB.
For better and faster indexation, keeping the sitemap URLs valuable and unique while decreasing the size of the sitemap files is beneficial.
12. Check The URL Count Per Sitemap With Python
Every URL within the sitemaps should have fewer than 50.000 URLs.
Use the Python code block below to check the URL Counts within the sitemap XML files.
(pd.pivot_table(sitemap_df, values=["loc"], index="sitemap", aggfunc="count") .sort_values(by="loc", ascending=False))
You can see the result below.

- All sitemaps have less than 50.000 URLs. Some sitemaps have only one URL, which wastes the search engine’s attention.
Keeping sitemap URLs that are frequently updated different from the static and stale content URLs is beneficial.
URL Count and URL Content character differences help a search engine to adjust crawl demand effectively for different website sections.
13. Check The Indexing And Crawling Meta Tags From URLs’ Content With Python
Even if a web page is not disallowed from robots.txt, it can still be disallowed from the HTML Meta Tags.
Thus, checking the HTML Meta Tags for better indexation and crawling is necessary.
Using the “custom selectors” is necessary to perform the HTML Meta Tag audit for the sitemap URLs.
sitemap = adv.sitemap_to_df("https://www.holisticseo.digital/sitemap.xml") adv.crawl(url_list=sitemap["loc"][:1000], output_file="meta_command_audit.jl", follow_links=False, xpath_selectors= {"meta_command": "//meta[@name="robots"]/@content"}, custom_settings={"CLOSESPIDER_PAGECOUNT":1000}) df_meta_check = pd.read_json("meta_command_audit.jl", lines=True) df_meta_check["meta_command"].str.contains("nofollow|noindex", regex=True).value_counts()
The “//meta[@name=”robots”]/@content” XPATH selector is to extract all the robots commands from the URLs from the sitemap.
We have used only the first 1000 URLs in the sitemap.
And, I stop crawling after the initial 1000 responses.
I have used another website to check the Crawling Meta Tags since ComplaintsBoard.com doesn’t have it on the source code.
You can see the result below.

- None of the URLs from the sitemap have “nofollow” or “noindex” within the “Robots” commands.
To check their values, use the code below.
df_meta_check[df_meta_check["meta_command"].str.contains("nofollow|noindex", regex=True) == False][["url", "meta_command"]]
You can see the result below.

14. Validate The Sitemap XML File Syntax With Python
Sitemap XML File Syntax validation is necessary to validate the integration of the sitemap file with the search engine’s perception.
Even if there are certain syntax errors, a search engine can recognize the sitemap file during the XML Normalization.
But, every syntax error can decrease the efficiency for certain levels.
Use the code block below to validate the Sitemap XML File Syntax.
def validate_sitemap_syntax(xml_path: str, xsd_path: str) xmlschema_doc = etree.parse(xsd_path) xmlschema = etree.XMLSchema(xmlschema_doc) xml_doc = etree.parse(xml_path) result = xmlschema.validate(xml_doc) return result validate_sitemap_syntax("sej_sitemap.xml", "sitemap.xsd")
For this example, I have used “https://www.searchenginejournal.com/sitemap_index.xml”. The XSD file involves the XML file’s context and tree structure.
It is stated in the first line of the Sitemap file as below.
For further information, you can also check DTD documentation.
15. Check The Open Graph URL And Canonical URL Matching
It is not a secret that search engines also use the Open Graph and RSS Feed URLs from the source code for further canonicalization and exploration.
The Open Graph URLs should be the same as the canonical URL submission.
From time to time, even in Google Discover, Google chooses to use the image from the Open Graph.
To check the Open Graph URL and Canonical URL consistency, use the code block below.
for i, df_chunk in enumerate(df_iterator): if "og:url" in df_chunk.columns: output_df = pd.DataFrame(data={ "canonical":df_chunk["canonical"], "og:url":df_chunk["og:url"], "open_graph_canonical_consistency":df_chunk["canonical"] == df_chunk["og:url"]}) mode="w" if i == 0 else 'a' header = i == 0 output_df.to_csv( "open_graph_canonical_consistency.csv", index=False, header=header, mode=mode ) else: print("There is no Open Graph URL Property")
There is no Open Graph URL Property
If there is an Open Graph URL Property on the website, it will give a CSV file to check whether the canonical URL and the Open Graph URL are the same or not.
But for this website, we don’t have an Open Graph URL.
Thus, I have used another website for the audit.
if "og:url" in df_meta_check.columns: output_df = pd.DataFrame(data={ "canonical":df_meta_check["canonical"], "og:url":df_meta_check["og:url"], "open_graph_canonical_consistency":df_meta_check["canonical"] == df_meta_check["og:url"]}) mode="w" if i == 0 else 'a' #header = i == 0 output_df.to_csv( "df_og_url_canonical_audit.csv", index=False, #header=header, mode=mode ) else: print("There is no Open Graph URL Property") df = pd.read_csv("df_og_url_canonical_audit.csv") df
You can see the result below.

We see that all canonical URLs and the Open Graph URLs are the same.

16. Check The Duplicate URLs Within Sitemap Submissions
A sitemap index file shouldn’t have duplicated URLs across different sitemap files or within the same sitemap XML file.
The duplication of the URLs within the sitemap files can make a search engine download the sitemap files less since a certain percentage of the sitemap file is bloated with unnecessary submissions.
For certain situations, it can appear as a spamming attempt to control the crawling schemes of the search engine crawlers.
use the code block below to check the duplicate URLs within the sitemap submissions.
sitemap_df["loc"].duplicated().value_counts()
You can see that the 49574 URLs from the sitemap are duplicated.

To see which sitemaps have more duplicated URLs, use the code block below.
pd.pivot_table(sitemap_df[sitemap_df["loc"].duplicated()==True], index="sitemap", values="loc", aggfunc="count").sort_values(by="loc", ascending=False)
You can see the result.

Chunking the sitemaps can help with site-tree and technical SEO analysis.
To see the duplicated URLs within the Sitemap, use the code block below.
sitemap_df[sitemap_df["loc"].duplicated() == True]
You can see the result below.

Conclusion
I wanted to show how to validate a sitemap file for better and healthier indexation and crawling for Technical SEO.
Python is vastly used for data science, machine learning, and natural language processing.
But, you can also use it for Technical SEO Audits to support the other SEO Verticals with a Holistic SEO Approach.
In a future article, we can expand these Technical SEO Audits further with different details and methods.
But, in general, this is one of the most comprehensive Technical SEO guides for Sitemaps and Sitemap Audit Tutorial with Python.
More resources:
Featured Image: elenasavchina2/Shutterstock
SEO
Google Declares It The “Gemini Era” As Revenue Grows 15%
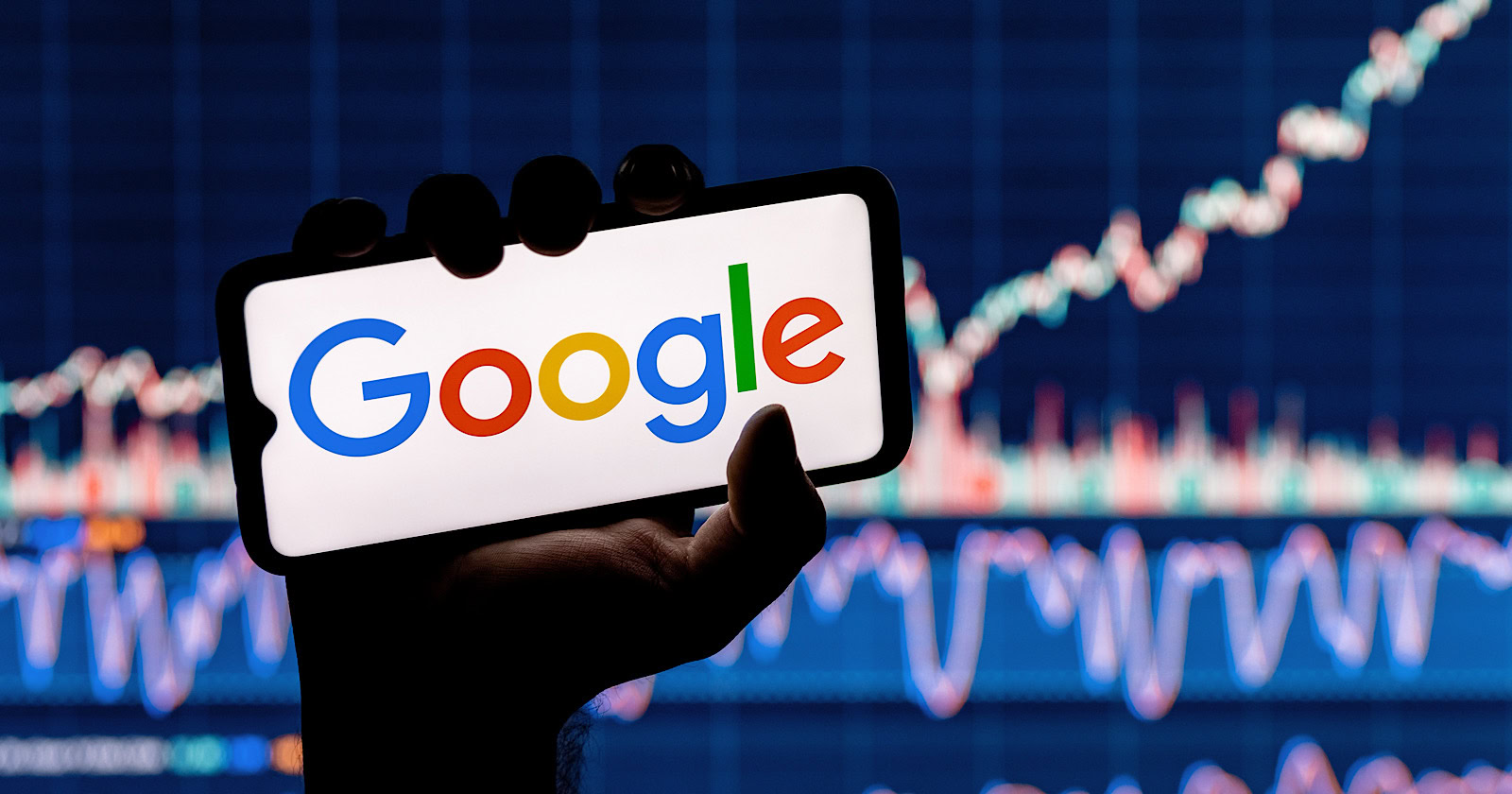
Alphabet Inc., Google’s parent company, announced its first quarter 2024 financial results today.
While Google reported double-digit growth in key revenue areas, the focus was on its AI developments, dubbed the “Gemini era” by CEO Sundar Pichai.
The Numbers: 15% Revenue Growth, Operating Margins Expand
Alphabet reported Q1 revenues of $80.5 billion, a 15% increase year-over-year, exceeding Wall Street’s projections.
Net income was $23.7 billion, with diluted earnings per share of $1.89. Operating margins expanded to 32%, up from 25% in the prior year.
Ruth Porat, Alphabet’s President and CFO, stated:
“Our strong financial results reflect revenue strength across the company and ongoing efforts to durably reengineer our cost base.”
Google’s core advertising units, such as Search and YouTube, drove growth. Google advertising revenues hit $61.7 billion for the quarter.
The Cloud division also maintained momentum, with revenues of $9.6 billion, up 28% year-over-year.
Pichai highlighted that YouTube and Cloud are expected to exit 2024 at a combined $100 billion annual revenue run rate.
Generative AI Integration in Search
Google experimented with AI-powered features in Search Labs before recently introducing AI overviews into the main search results page.
Regarding the gradual rollout, Pichai states:
“We are being measured in how we do this, focusing on areas where gen AI can improve the Search experience, while also prioritizing traffic to websites and merchants.”
Pichai reports that Google’s generative AI features have answered over a billion queries already:
“We’ve already served billions of queries with our generative AI features. It’s enabling people to access new information, to ask questions in new ways, and to ask more complex questions.”
Google reports increased Search usage and user satisfaction among those interacting with the new AI overview results.
The company also highlighted its “Circle to Search” feature on Android, which allows users to circle objects on their screen or in videos to get instant AI-powered answers via Google Lens.
Reorganizing For The “Gemini Era”
As part of the AI roadmap, Alphabet is consolidating all teams building AI models under the Google DeepMind umbrella.
Pichai revealed that, through hardware and software improvements, the company has reduced machine costs associated with its generative AI search results by 80% over the past year.
He states:
“Our data centers are some of the most high-performing, secure, reliable and efficient in the world. We’ve developed new AI models and algorithms that are more than one hundred times more efficient than they were 18 months ago.
How Will Google Make Money With AI?
Alphabet sees opportunities to monetize AI through its advertising products, Cloud offerings, and subscription services.
Google is integrating Gemini into ad products like Performance Max. The company’s Cloud division is bringing “the best of Google AI” to enterprise customers worldwide.
Google One, the company’s subscription service, surpassed 100 million paid subscribers in Q1 and introduced a new premium plan featuring advanced generative AI capabilities powered by Gemini models.
Future Outlook
Pichai outlined six key advantages positioning Alphabet to lead the “next wave of AI innovation”:
- Research leadership in AI breakthroughs like the multimodal Gemini model
- Robust AI infrastructure and custom TPU chips
- Integrating generative AI into Search to enhance the user experience
- A global product footprint reaching billions
- Streamlined teams and improved execution velocity
- Multiple revenue streams to monetize AI through advertising and cloud
With upcoming events like Google I/O and Google Marketing Live, the company is expected to share further updates on its AI initiatives and product roadmap.
Featured Image: Sergei Elagin/Shutterstock
SEO
brightonSEO Live Blog
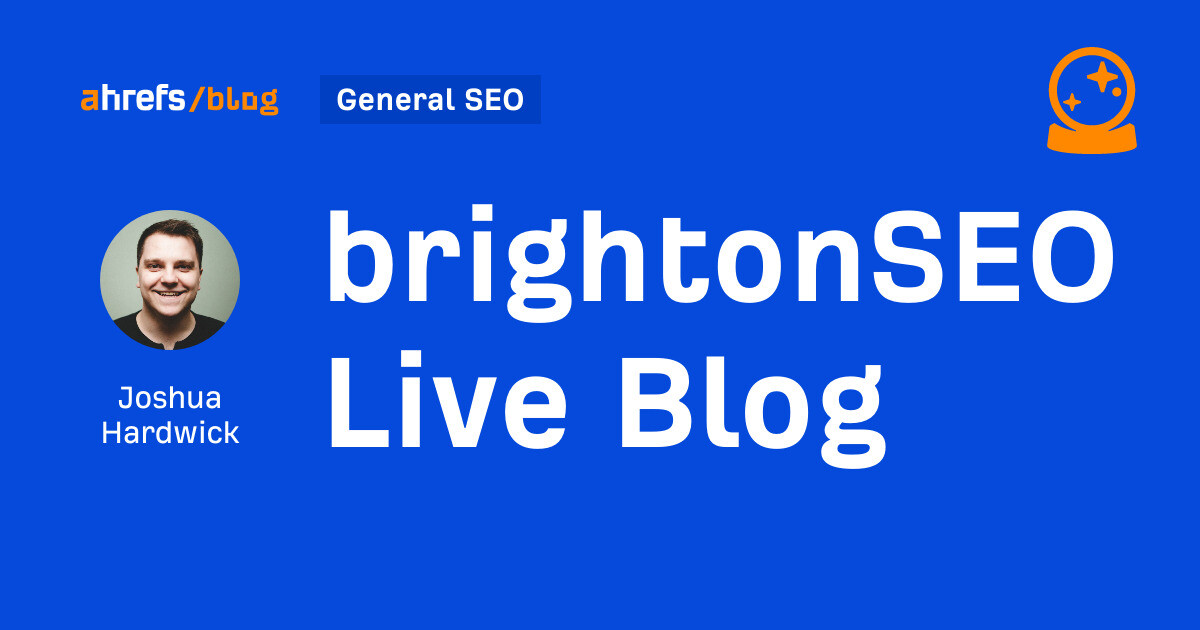
Hello everyone. It’s April again, so I’m back in Brighton for another two days of Being the introvert I am, my idea of fun isn’t hanging around our booth all day explaining we’ve run out of t-shirts (seriously, you need to be fast if you want swag!). So I decided to do something useful and live-blog the event instead.
Follow below for talk takeaways and (very) mildly humorous commentary. sun, sea, and SEO!
SEO
Google Further Postpones Third-Party Cookie Deprecation In Chrome
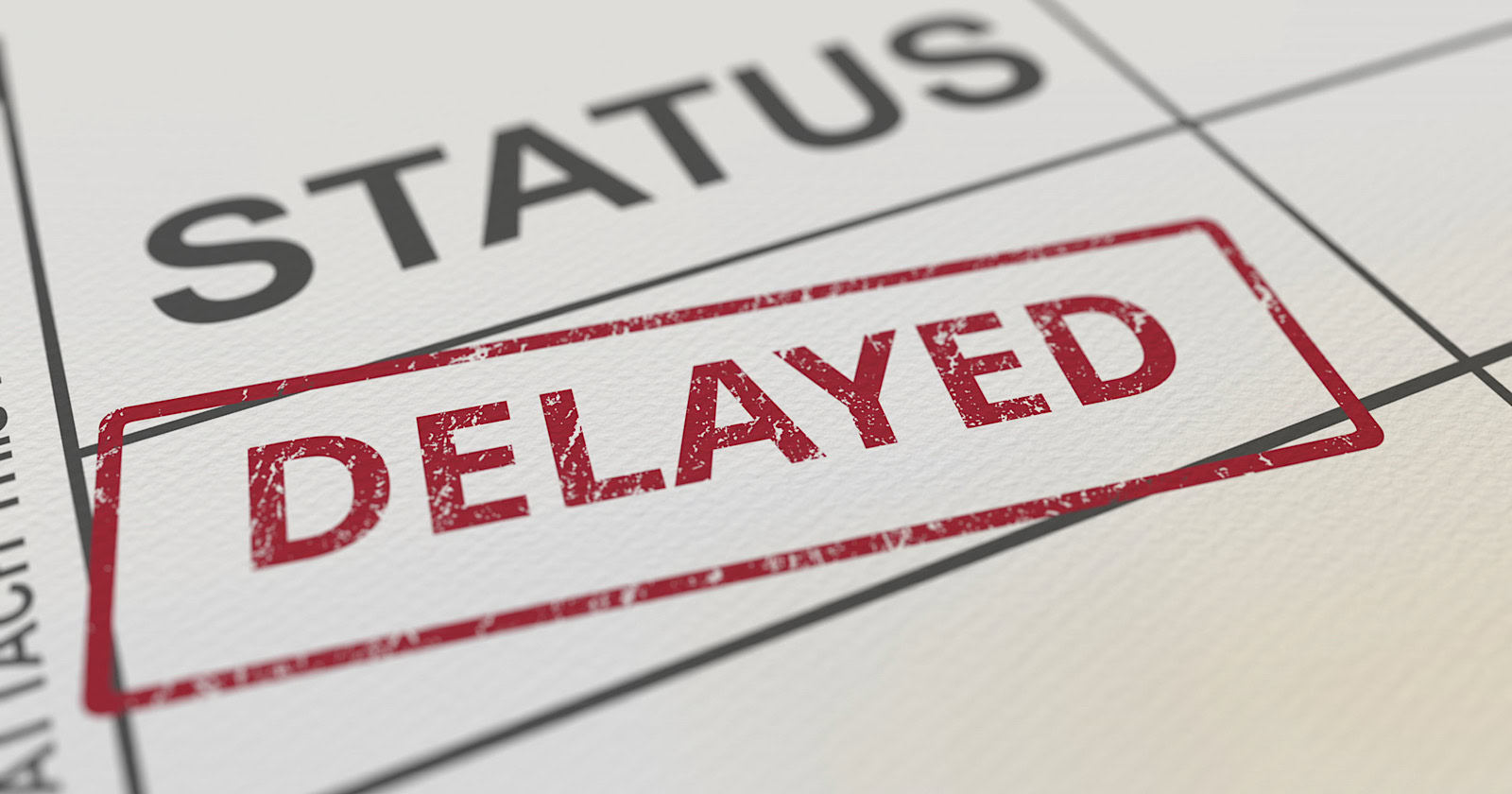
Google has again delayed its plan to phase out third-party cookies in the Chrome web browser. The latest postponement comes after ongoing challenges in reconciling feedback from industry stakeholders and regulators.
The announcement was made in Google and the UK’s Competition and Markets Authority (CMA) joint quarterly report on the Privacy Sandbox initiative, scheduled for release on April 26.
Chrome’s Third-Party Cookie Phaseout Pushed To 2025
Google states it “will not complete third-party cookie deprecation during the second half of Q4” this year as planned.
Instead, the tech giant aims to begin deprecating third-party cookies in Chrome “starting early next year,” assuming an agreement can be reached with the CMA and the UK’s Information Commissioner’s Office (ICO).
The statement reads:
“We recognize that there are ongoing challenges related to reconciling divergent feedback from the industry, regulators and developers, and will continue to engage closely with the entire ecosystem. It’s also critical that the CMA has sufficient time to review all evidence, including results from industry tests, which the CMA has asked market participants to provide by the end of June.”
Continued Engagement With Regulators
Google reiterated its commitment to “engaging closely with the CMA and ICO” throughout the process and hopes to conclude discussions this year.
This marks the third delay to Google’s plan to deprecate third-party cookies, initially aiming for a Q3 2023 phaseout before pushing it back to late 2024.
The postponements reflect the challenges in transitioning away from cross-site user tracking while balancing privacy and advertiser interests.
Transition Period & Impact
In January, Chrome began restricting third-party cookie access for 1% of users globally. This percentage was expected to gradually increase until 100% of users were covered by Q3 2024.
However, the latest delay gives websites and services more time to migrate away from third-party cookie dependencies through Google’s limited “deprecation trials” program.
The trials offer temporary cookie access extensions until December 27, 2024, for non-advertising use cases that can demonstrate direct user impact and functional breakage.
While easing the transition, the trials have strict eligibility rules. Advertising-related services are ineligible, and origins matching known ad-related domains are rejected.
Google states the program aims to address functional issues rather than relieve general data collection inconveniences.
Publisher & Advertiser Implications
The repeated delays highlight the potential disruption for digital publishers and advertisers relying on third-party cookie tracking.
Industry groups have raised concerns that restricting cross-site tracking could push websites toward more opaque privacy-invasive practices.
However, privacy advocates view the phaseout as crucial in preventing covert user profiling across the web.
With the latest postponement, all parties have more time to prepare for the eventual loss of third-party cookies and adopt Google’s proposed Privacy Sandbox APIs as replacements.
Featured Image: Novikov Aleksey/Shutterstock
-
PPC7 days ago
19 Best SEO Tools in 2024 (For Every Use Case)
-
SEARCHENGINES6 days ago
Daily Search Forum Recap: April 19, 2024
-
WORDPRESS7 days ago
How to Make $5000 of Passive Income Every Month in WordPress
-
WORDPRESS5 days ago
13 Best HubSpot Alternatives for 2024 (Free + Paid)
-
MARKETING6 days ago
Battling for Attention in the 2024 Election Year Media Frenzy
-
SEO7 days ago
25 WordPress Alternatives Best For SEO
-
WORDPRESS6 days ago
7 Best WooCommerce Points and Rewards Plugins (Free & Paid)
-
AFFILIATE MARKETING7 days ago
AI Will Transform the Workplace. Here’s How HR Can Prepare for It.
You must be logged in to post a comment Login