SEO
Using Python + Streamlit To Find Striking Distance Keyword Opportunities
Python is an excellent tool to automate repetitive tasks as well as gain additional insights into data.
In this article, you’ll learn how to build a tool to check which keywords are close to ranking in positions one to three and advises whether there is an opportunity to naturally work those keywords into the page.
It’s perfect for Python beginners and pros alike and is a great introduction to using Python for SEO.
If you’d just like to get stuck in there’s a handy Streamlit app available for the code. This is simple to use and requires no coding experience.
There’s also a Google Colaboratory Sheet if you’d like to poke around with the code. If you can crawl a website, you can use this script!
Here’s an example of what we’ll be making today:

These keywords are found in the page title and H1, but not in the copy. Adding these keywords naturally to the existing copy would be an easy way to increase relevancy for these keywords.
By taking the hint from search engines and naturally including any missing keywords a site already ranks for, we increase the confidence of search engines to rank those keywords higher in the SERPs.
This report can be created manually, but it’s pretty time-consuming.
So, we’re going to automate the process using a Python SEO script.
Preview Of The Output
This is a sample of what the final output will look like after running the report:


The final output takes the top five opportunities by search volume for each page and neatly lays each one horizontally along with the estimated search volume.
It also shows the total search volume of all keywords a page has within striking distance, as well as the total number of keywords within reach.
The top five keywords by search volume are then checked to see if they are found in the title, H1, or copy, then flagged TRUE or FALSE.
This is great for finding quick wins! Just add the missing keyword naturally into the page copy, title, or H1.
Getting Started
The setup is fairly straightforward. We just need a crawl of the site (ideally with a custom extraction for the copy you’d like to check), and an exported file of all keywords a site ranks for.
This post will walk you through the setup, the code, and will link to a Google Colaboratory sheet if you just want to get stuck in without coding it yourself.
To get started you will need:
We’ve named this the Striking Distance Report as it flags keywords that are easily within striking distance.
(We have defined striking distance as keywords that rank in positions four to 20, but have made this a configurable option in case you would like to define your own parameters.)
Striking Distance SEO Report: Getting Started
1. Crawl The Target Website
- Set a custom extractor for the page copy (optional, but recommended).
- Filter out pagination pages from the crawl.
2. Export All Keywords The Site Ranks For Using Your Favorite Provider
- Filter keywords that trigger as a site link.
- Remove keywords that trigger as an image.
- Filter branded keywords.
- Use both exports to create an actionable Striking Distance report from the keyword and crawl data with Python.
Crawling The Site
I’ve opted to use Screaming Frog to get the initial crawl. Any crawler will work, so long as the CSV export uses the same column names or they’re renamed to match.
The script expects to find the following columns in the crawl CSV export:
"Address", "Title 1", "H1-1", "Copy 1", "Indexability"
Crawl Settings
The first thing to do is to head over to the main configuration settings within Screaming Frog:
Configuration > Spider > Crawl
The main settings to use are:
Crawl Internal Links, Canonicals, and the Pagination (Rel Next/Prev) setting.
(The script will work with everything else selected, but the crawl will take longer to complete!)


Next, it’s on to the Extraction tab.
Configuration > Spider > Extraction


At a bare minimum, we need to extract the page title, H1, and calculate whether the page is indexable as shown below.
Indexability is useful because it’s an easy way for the script to identify which URLs to drop in one go, leaving only keywords that are eligible to rank in the SERPs.
If the script cannot find the indexability column, it’ll still work as normal but won’t differentiate between pages that can and cannot rank.
Setting A Custom Extractor For Page Copy
In order to check whether a keyword is found within the page copy, we need to set a custom extractor in Screaming Frog.
Configuration > Custom > Extraction
Name the extractor “Copy” as seen below.


Important: The script expects the extractor to be named “Copy” as above, so please double check!
Lastly, make sure Extract Text is selected to export the copy as text, rather than HTML.
There are many guides on using custom extractors online if you need help setting one up, so I won’t go over it again here.
Once the extraction has been set it’s time to crawl the site and export the HTML file in CSV format.
Exporting The CSV File
Exporting the CSV file is as easy as changing the drop-down menu displayed underneath Internal to HTML and pressing the Export button.
Internal > HTML > Export


After clicking Export, It’s important to make sure the type is set to CSV format.
The export screen should look like the below:


Tip 1: Filtering Out Pagination Pages
I recommend filtering out pagination pages from your crawl either by selecting Respect Next/Prev under the Advanced settings (or just deleting them from the CSV file, if you prefer).


Tip 2: Saving The Crawl Settings
Once you have set the crawl up, it’s worth just saving the crawl settings (which will also remember the custom extraction).
This will save a lot of time if you want to use the script again in the future.
File > Configuration > Save As


Exporting Keywords
Once we have the crawl file, the next step is to load your favorite keyword research tool and export all of the keywords a site ranks for.
The goal here is to export all the keywords a site ranks for, filtering out branded keywords and any which triggered as a sitelink or image.
For this example, I’m using the Organic Keyword Report in Ahrefs, but it will work just as well with Semrush if that’s your preferred tool.
In Ahrefs, enter the domain you’d like to check in Site Explorer and choose Organic Keywords.


Site Explorer > Organic Keywords


This will bring up all keywords the site is ranking for.
Filtering Out Sitelinks And Image links
The next step is to filter out any keywords triggered as a sitelink or an image pack.
The reason we need to filter out sitelinks is that they have no influence on the parent URL ranking. This is because only the parent page technically ranks for the keyword, not the sitelink URLs displayed under it.
Filtering out sitelinks will ensure that we are optimizing the correct page.


Here’s how to do it in Ahrefs.


Lastly, I recommend filtering out any branded keywords. You can do this by filtering the CSV output directly, or by pre-filtering in the keyword tool of your choice before the export.
Finally, when exporting make sure to choose Full Export and the UTF-8 format as shown below.


By default, the script works with Ahrefs (v1/v2) and Semrush keyword exports. It can work with any keyword CSV file as long as the column names the script expects are present.
Processing
The following instructions pertain to running a Google Colaboratory sheet to execute the code.
There is now a simpler option for those that prefer it in the form of a Streamlit app. Simply follow the instructions provided to upload your crawl and keyword file.
Now that we have our exported files, all that’s left to be done is to upload them to the Google Colaboratory sheet for processing.
Select Runtime > Run all from the top navigation to run all cells in the sheet.


The script will prompt you to upload the keyword CSV from Ahrefs or Semrush first and the crawl file afterward.


That’s it! The script will automatically download an actionable CSV file you can use to optimize your site.


Once you’re familiar with the whole process, using the script is really straightforward.
Code Breakdown And Explanation
If you’re learning Python for SEO and interested in what the code is doing to produce the report, stick around for the code walkthrough!
Install The Libraries
Let’s install pandas to get the ball rolling.
!pip install pandas
Import The Modules
Next, we need to import the required modules.
import pandas as pd from pandas import DataFrame, Series from typing import Union from google.colab import files
Set The Variables
Now it’s time to set the variables.
The script considers any keywords between positions four and 20 as within striking distance.
Changing the variables here will let you define your own range if desired. It’s worth experimenting with the settings to get the best possible output for your needs.
# set all variables here min_volume = 10 # set the minimum search volume min_position = 4 # set the minimum position / default = 4 max_position = 20 # set the maximum position / default = 20 drop_all_true = True # If all checks (h1/title/copy) are true, remove the recommendation (Nothing to do) pagination_filters = "filterby|page|p=" # filter patterns used to detect and drop paginated pages
Upload The Keyword Export CSV File
The next step is to read in the list of keywords from the CSV file.
It is set up to accept an Ahrefs report (V1 and V2) as well as a Semrush export.
This code reads in the CSV file into a Pandas DataFrame.
upload = files.upload() upload = list(upload.keys())[0] df_keywords = pd.read_csv( (upload), error_bad_lines=False, low_memory=False, encoding="utf8", dtype={ "URL": "str", "Keyword": "str", "Volume": "str", "Position": int, "Current URL": "str", "Search Volume": int, }, ) print("Uploaded Keyword CSV File Successfully!")
If everything went to plan, you’ll see a preview of the DataFrame created from the keyword CSV export.


Upload The Crawl Export CSV File
Once the keywords have been imported, it’s time to upload the crawl file.
This fairly simple piece of code reads in the crawl with some error handling option and creates a Pandas DataFrame named df_crawl. upload = files.upload() upload = list(upload.keys())[0] df_crawl = pd.read_csv( (upload), error_bad_lines=False, low_memory=False, encoding="utf8", dtype="str", ) print("Uploaded Crawl Dataframe Successfully!")
Once the CSV file has finished uploading, you’ll see a preview of the DataFrame.


Clean And Standardize The Keyword Data
The next step is to rename the column names to ensure standardization between the most common types of file exports.
Essentially, we’re getting the keyword DataFrame into a good state and filtering using cutoffs defined by the variables.
df_keywords.rename( columns={ "Current position": "Position", "Current URL": "URL", "Search Volume": "Volume", }, inplace=True, ) # keep only the following columns from the keyword dataframe cols = "URL", "Keyword", "Volume", "Position" df_keywords = df_keywords.reindex(columns=cols) try: # clean the data. (v1 of the ahrefs keyword export combines strings and ints in the volume column) df_keywords["Volume"] = df_keywords["Volume"].str.replace("0-10", "0") except AttributeError: pass # clean the keyword data df_keywords = df_keywords[df_keywords["URL"].notna()] # remove any missing values df_keywords = df_keywords[df_keywords["Volume"].notna()] # remove any missing values df_keywords = df_keywords.astype({"Volume": int}) # change data type to int df_keywords = df_keywords.sort_values(by="Volume", ascending=False) # sort by highest vol to keep the top opportunity # make new dataframe to merge search volume back in later df_keyword_vol = df_keywords[["Keyword", "Volume"]] # drop rows if minimum search volume doesn't match specified criteria df_keywords.loc[df_keywords["Volume"] < min_volume, "Volume_Too_Low"] = "drop" df_keywords = df_keywords[~df_keywords["Volume_Too_Low"].isin(["drop"])] # drop rows if minimum search position doesn't match specified criteria df_keywords.loc[df_keywords["Position"] <= min_position, "Position_Too_High"] = "drop" df_keywords = df_keywords[~df_keywords["Position_Too_High"].isin(["drop"])] # drop rows if maximum search position doesn't match specified criteria df_keywords.loc[df_keywords["Position"] >= max_position, "Position_Too_Low"] = "drop" df_keywords = df_keywords[~df_keywords["Position_Too_Low"].isin(["drop"])]
Clean And Standardize The Crawl Data
Next, we need to clean and standardize the crawl data.
Essentially, we use reindex to only keep the “Address,” “Indexability,” “Page Title,” “H1-1,” and “Copy 1” columns, discarding the rest.
We use the handy “Indexability” column to only keep rows that are indexable. This will drop canonicalized URLs, redirects, and so on. I recommend enabling this option in the crawl.
Lastly, we standardize the column names so they’re a little nicer to work with.
# keep only the following columns from the crawl dataframe cols = "Address", "Indexability", "Title 1", "H1-1", "Copy 1" df_crawl = df_crawl.reindex(columns=cols) # drop non-indexable rows df_crawl = df_crawl[~df_crawl["Indexability"].isin(["Non-Indexable"])] # standardise the column names df_crawl.rename(columns={"Address": "URL", "Title 1": "Title", "H1-1": "H1", "Copy 1": "Copy"}, inplace=True) df_crawl.head()
Group The Keywords
As we approach the final output, it’s necessary to group our keywords together to calculate the total opportunity for each page.
Here, we’re calculating how many keywords are within striking distance for each page, along with the combined search volume.
# groups the URLs (remove the dupes and combines stats) # make a copy of the keywords dataframe for grouping - this ensures stats can be merged back in later from the OG df df_keywords_group = df_keywords.copy() df_keywords_group["KWs in Striking Dist."] = 1 # used to count the number of keywords in striking distance df_keywords_group = ( df_keywords_group.groupby("URL") .agg({"Volume": "sum", "KWs in Striking Dist.": "count"}) .reset_index() ) df_keywords_group.head()


Once complete, you’ll see a preview of the DataFrame.
Display Keywords In Adjacent Rows
We use the grouped data as the basis for the final output. We use Pandas.unstack to reshape the DataFrame to display the keywords in the style of a GrepWords export.


# create a new df, combine the merged data with the original data. display in adjacent rows ala grepwords df_merged_all_kws = df_keywords_group.merge( df_keywords.groupby("URL")["Keyword"] .apply(lambda x: x.reset_index(drop=True)) .unstack() .reset_index() ) # sort by biggest opportunity df_merged_all_kws = df_merged_all_kws.sort_values( by="KWs in Striking Dist.", ascending=False ) # reindex the columns to keep just the top five keywords cols = "URL", "Volume", "KWs in Striking Dist.", 0, 1, 2, 3, 4 df_merged_all_kws = df_merged_all_kws.reindex(columns=cols) # create union and rename the columns df_striking: Union[Series, DataFrame, None] = df_merged_all_kws.rename( columns={ "Volume": "Striking Dist. Vol", 0: "KW1", 1: "KW2", 2: "KW3", 3: "KW4", 4: "KW5", } ) # merges striking distance df with crawl df to merge in the title, h1 and category description df_striking = pd.merge(df_striking, df_crawl, on="URL", how="inner")
Set The Final Column Order And Insert Placeholder Columns
Lastly, we set the final column order and merge in the original keyword data.
There are a lot of columns to sort and create!
# set the final column order and merge the keyword data in cols = [ "URL", "Title", "H1", "Copy", "Striking Dist. Vol", "KWs in Striking Dist.", "KW1", "KW1 Vol", "KW1 in Title", "KW1 in H1", "KW1 in Copy", "KW2", "KW2 Vol", "KW2 in Title", "KW2 in H1", "KW2 in Copy", "KW3", "KW3 Vol", "KW3 in Title", "KW3 in H1", "KW3 in Copy", "KW4", "KW4 Vol", "KW4 in Title", "KW4 in H1", "KW4 in Copy", "KW5", "KW5 Vol", "KW5 in Title", "KW5 in H1", "KW5 in Copy", ] # re-index the columns to place them in a logical order + inserts new blank columns for kw checks. df_striking = df_striking.reindex(columns=cols)
Merge In The Keyword Data For Each Column
This code merges the keyword volume data back into the DataFrame. It’s more or less the equivalent of an Excel VLOOKUP function.
# merge in keyword data for each keyword column (KW1 - KW5) df_striking = pd.merge(df_striking, df_keyword_vol, left_on="KW1", right_on="Keyword", how="left") df_striking['KW1 Vol'] = df_striking['Volume'] df_striking.drop(['Keyword', 'Volume'], axis=1, inplace=True) df_striking = pd.merge(df_striking, df_keyword_vol, left_on="KW2", right_on="Keyword", how="left") df_striking['KW2 Vol'] = df_striking['Volume'] df_striking.drop(['Keyword', 'Volume'], axis=1, inplace=True) df_striking = pd.merge(df_striking, df_keyword_vol, left_on="KW3", right_on="Keyword", how="left") df_striking['KW3 Vol'] = df_striking['Volume'] df_striking.drop(['Keyword', 'Volume'], axis=1, inplace=True) df_striking = pd.merge(df_striking, df_keyword_vol, left_on="KW4", right_on="Keyword", how="left") df_striking['KW4 Vol'] = df_striking['Volume'] df_striking.drop(['Keyword', 'Volume'], axis=1, inplace=True) df_striking = pd.merge(df_striking, df_keyword_vol, left_on="KW5", right_on="Keyword", how="left") df_striking['KW5 Vol'] = df_striking['Volume'] df_striking.drop(['Keyword', 'Volume'], axis=1, inplace=True)
Clean The Data Some More
The data requires additional cleaning to populate empty values, (NaNs), as empty strings. This improves the readability of the final output by creating blank cells, instead of cells populated with NaN string values.
Next, we convert the columns to lowercase so that they match when checking whether a target keyword is featured in a specific column.
# replace nan values with empty strings df_striking = df_striking.fillna("") # drop the title, h1 and category description to lower case so kws can be matched to them df_striking["Title"] = df_striking["Title"].str.lower() df_striking["H1"] = df_striking["H1"].str.lower() df_striking["Copy"] = df_striking["Copy"].str.lower()
Check Whether The Keyword Appears In The Title/H1/Copy and Return True Or False
This code checks if the target keyword is found in the page title/H1 or copy.
It’ll flag true or false depending on whether a keyword was found within the on-page elements.
df_striking["KW1 in Title"] = df_striking.apply(lambda row: row["KW1"] in row["Title"], axis=1) df_striking["KW1 in H1"] = df_striking.apply(lambda row: row["KW1"] in row["H1"], axis=1) df_striking["KW1 in Copy"] = df_striking.apply(lambda row: row["KW1"] in row["Copy"], axis=1) df_striking["KW2 in Title"] = df_striking.apply(lambda row: row["KW2"] in row["Title"], axis=1) df_striking["KW2 in H1"] = df_striking.apply(lambda row: row["KW2"] in row["H1"], axis=1) df_striking["KW2 in Copy"] = df_striking.apply(lambda row: row["KW2"] in row["Copy"], axis=1) df_striking["KW3 in Title"] = df_striking.apply(lambda row: row["KW3"] in row["Title"], axis=1) df_striking["KW3 in H1"] = df_striking.apply(lambda row: row["KW3"] in row["H1"], axis=1) df_striking["KW3 in Copy"] = df_striking.apply(lambda row: row["KW3"] in row["Copy"], axis=1) df_striking["KW4 in Title"] = df_striking.apply(lambda row: row["KW4"] in row["Title"], axis=1) df_striking["KW4 in H1"] = df_striking.apply(lambda row: row["KW4"] in row["H1"], axis=1) df_striking["KW4 in Copy"] = df_striking.apply(lambda row: row["KW4"] in row["Copy"], axis=1) df_striking["KW5 in Title"] = df_striking.apply(lambda row: row["KW5"] in row["Title"], axis=1) df_striking["KW5 in H1"] = df_striking.apply(lambda row: row["KW5"] in row["H1"], axis=1) df_striking["KW5 in Copy"] = df_striking.apply(lambda row: row["KW5"] in row["Copy"], axis=1)
Delete True/False Values If There Is No Keyword
This will delete true/false values when there is no keyword adjacent.
# delete true / false values if there is no keyword df_striking.loc[df_striking["KW1"] == "", ["KW1 in Title", "KW1 in H1", "KW1 in Copy"]] = "" df_striking.loc[df_striking["KW2"] == "", ["KW2 in Title", "KW2 in H1", "KW2 in Copy"]] = "" df_striking.loc[df_striking["KW3"] == "", ["KW3 in Title", "KW3 in H1", "KW3 in Copy"]] = "" df_striking.loc[df_striking["KW4"] == "", ["KW4 in Title", "KW4 in H1", "KW4 in Copy"]] = "" df_striking.loc[df_striking["KW5"] == "", ["KW5 in Title", "KW5 in H1", "KW5 in Copy"]] = "" df_striking.head()
Drop Rows If All Values == True
This configurable option is really useful for reducing the amount of QA time required for the final output by dropping the keyword opportunity from the final output if it is found in all three columns.
def true_dropper(col1, col2, col3): drop = df_striking.drop( df_striking[ (df_striking[col1] == True) & (df_striking[col2] == True) & (df_striking[col3] == True) ].index ) return drop if drop_all_true == True: df_striking = true_dropper("KW1 in Title", "KW1 in H1", "KW1 in Copy") df_striking = true_dropper("KW2 in Title", "KW2 in H1", "KW2 in Copy") df_striking = true_dropper("KW3 in Title", "KW3 in H1", "KW3 in Copy") df_striking = true_dropper("KW4 in Title", "KW4 in H1", "KW4 in Copy") df_striking = true_dropper("KW5 in Title", "KW5 in H1", "KW5 in Copy")
Download The CSV File
The last step is to download the CSV file and start the optimization process.
df_striking.to_csv('Keywords in Striking Distance.csv', index=False) files.download("Keywords in Striking Distance.csv")
Conclusion
If you are looking for quick wins for any website, the striking distance report is a really easy way to find them.
Don’t let the number of steps fool you. It’s not as complex as it seems. It’s as simple as uploading a crawl and keyword export to the supplied Google Colab sheet or using the Streamlit app.
The results are definitely worth it!
More Resources:
Featured Image: aurielaki/Shutterstock
!function(f,b,e,v,n,t,s)
{if(f.fbq)return;n=f.fbq=function(){n.callMethod?
n.callMethod.apply(n,arguments):n.queue.push(arguments)};
if(!f._fbq)f._fbq=n;n.push=n;n.loaded=!0;n.version=’2.0′;
n.queue=[];t=b.createElement(e);t.async=!0;
t.src=v;s=b.getElementsByTagName(e)[0];
s.parentNode.insertBefore(t,s)}(window,document,’script’,
‘https://connect.facebook.net/en_US/fbevents.js’);
if( typeof sopp !== “undefined” && sopp === ‘yes’ ){
fbq(‘dataProcessingOptions’, [‘LDU’], 1, 1000);
}else{
fbq(‘dataProcessingOptions’, []);
}
fbq(‘init’, ‘1321385257908563’);
fbq(‘track’, ‘PageView’);
fbq(‘trackSingle’, ‘1321385257908563’, ‘ViewContent’, {
content_name: ‘python-seo-striking-distance’,
content_category: ‘seo-strategy technical-seo’
});
SEO
How to Revive an Old Blog Article for SEO
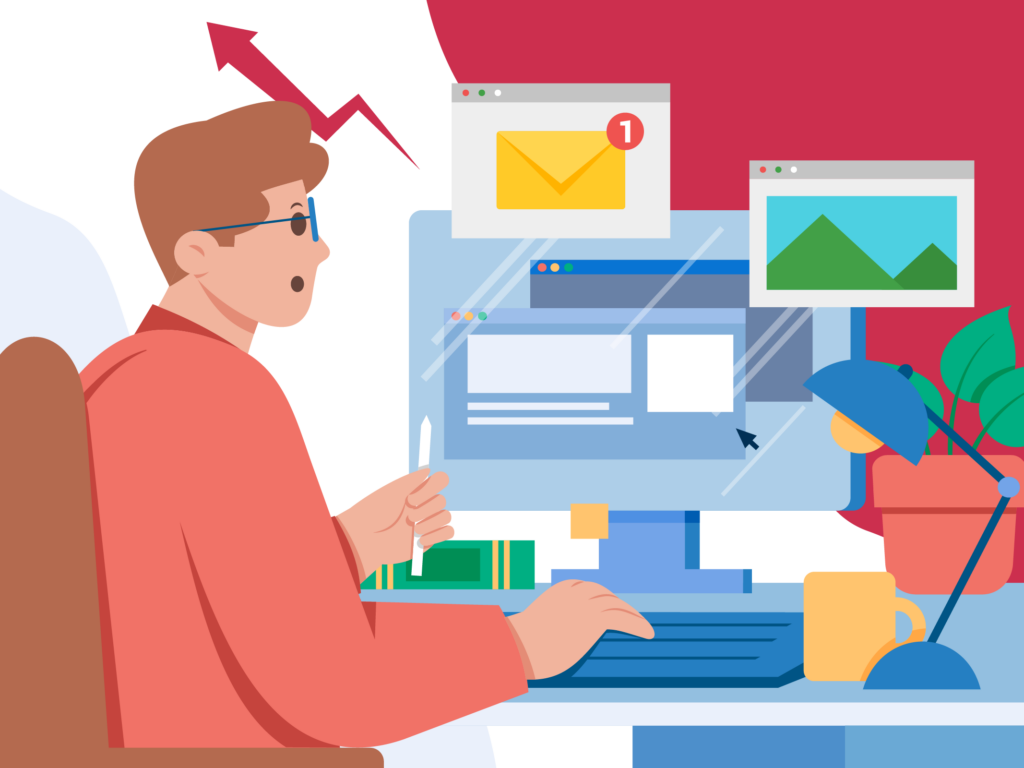
Quick question: What do you typically do with your old blog posts? Most likely, the answer is: Not much.
If that’s the case, you’re not alone. Many of us in SEO and content marketing tend to focus on continuously creating new content, rather than leveraging our existing blog posts.
However, here’s the reality—Google is becoming increasingly sophisticated in evaluating content quality, and we need to adapt accordingly. Just as it’s easier to encourage existing customers to make repeat purchases, updating old content on your website is a more efficient and sustainable strategy in the long run.
Ways to Optimize Older Content
Some of your old content might not be optimized for SEO very well, rank for irrelevant keywords, or drive no traffic at all. If the quality is still decent, however, you should be able to optimize it properly with little effort.
Refresh Content
If your blog post contains a specific year or mentions current events, it may become outdated over time. If the rest of the content is still relevant (like if it’s targeting an evergreen topic), simply updating the date might be all you need to do.
Rewrite Old Blog Posts
When the content quality is low (you might have greatly improved your writing skills since you’ve written the post) but the potential is still there, there’s not much you can do apart from rewriting an old blog post completely.
This is not a waste—you’re saving time on brainstorming since the basic structure is already in place. Now, focus on improving the quality.
Delete Old Blog Posts
You might find a blog post that just seems unusable. Should you delete your old content? It depends. If it’s completely outdated, of low quality, and irrelevant to any valuable keywords for your website, it’s better to remove it.
Once you decide to delete the post, don’t forget to set up a 301 redirect to a related post or page, or to your homepage.
Promote Old Blog Posts
Sometimes all your content needs is a bit of promotion to start ranking and getting traffic again. Share it on your social media, link to it from a new post – do something to get it discoverable again to your audience. This can give it the boost it needs to attract organic links too.
Which Blog Posts Should You Update?
Deciding when to update or rewrite blog posts is a decision that relies on one important thing: a content audit.
Use your Google Analytics to find out which blog posts used to drive tons of traffic, but no longer have the same reach. You can also use Google Search Console to find out which of your blog posts have lost visibility in comparison to previous months. I have a guide on website analysis using Google Analytics and Google Search Console you can follow.
If you use keyword tracking tools like SE Ranking, you can also use the data it provides to come up with a list of blog posts that have dropped in the rankings.
Make data-driven decisions to identify which blog posts would benefit from these updates – i.e., which ones still have the chance to recover their keyword rankings and organic traffic.
With Google’s helpful content update, which emphasizes better user experiences, it’s crucial to ensure your content remains relevant, valuable, and up-to-date.
How To Update Old Blog Posts for SEO
Updating articles can be an involved process. Here are some tips and tactics to help you get it right.
Author’s Note: I have a Comprehensive On-Page SEO Checklist you might also be interested in following while you’re doing your content audit.
Conduct New Keyword Research
Updating your post without any guide won’t get you far. Always do your keyword research to understand how users are searching for your given topic.
Proper research can also show you relevant questions and sections that can be added to the blog post you’re updating or rewriting. Make sure to take a look at the People Also Ask (PAA) section that shows up when you search for your target keyword. Check out other websites like Answer The Public, Reddit, and Quora to see what users are looking for too.
Look for New Ranking Opportunities
When trying to revive an old blog post for SEO, keep an eye out for new SEO opportunities (e.g., AI Overview, featured snippets, and related search terms) that didn’t exist when you first wrote your blog post. Some of these features can be targeted by the new content you will add to your post, if you write with the aim to be eligible for it.
Rewrite Headlines and Meta Tags
If you want to attract new readers, consider updating your headlines and meta tags.
Your headlines and meta tags should fulfill these three things:
- Reflect the rewritten and new content you’ve added to the blog post.
- Be optimized for the new keywords it’s targeting (if any).
- Appeal to your target audience – who may have changed tastes from when the blog post was originally made.
Remember that your meta tags in particular act like a brief advertisement for your blog post, since this is what the user first sees when your blog post is shown in the search results page.
Take a look at your blog post’s click-through rate on Google Search Console – if it falls below 2%, it’s definitely time for new meta tags.
Replace Outdated Information and Statistics
Updating blog content with current studies and statistics enhances the relevance and credibility of your post. By providing up-to-date information, you help your audience make better, well-informed decisions, while also showing that your content is trustworthy.
Tighten or Expand Ideas
Your old content might be too short to provide real value to users – or you might have rambled on and on in your post. It’s important to evaluate whether you need to make your content more concise, or if you need to elaborate more.
Keep the following tips in mind as you refine your blog post’s ideas:
- Evaluate Helpfulness: Measure how well your content addresses your readers’ pain points. Aim to follow the E-E-A-T model (Experience, Expertise, Authoritativeness, Trustworthiness).
- Identify Missing Context: Consider whether your content needs more detail or clarification. View it from your audience’s perspective and ask if the information is complete, or if more information is needed.
- Interview Experts: Speak with industry experts or thought leaders to get fresh insights. This will help support your writing, and provide unique points that enhance the value of your content.
- Use Better Examples: Examples help simplify complex concepts. Add new examples or improve existing ones to strengthen your points.
- Add New Sections if Needed: If your content lacks depth or misses a key point, add new sections to cover these areas more thoroughly.
- Remove Fluff: Every sentence should contribute to the overall narrative. Eliminate unnecessary content to make your post more concise.
- Revise Listicles: Update listicle items based on SEO recommendations and content quality. Add or remove headings to stay competitive with higher-ranking posts.
Improve Visuals and Other Media
No doubt that there are tons of old graphics and photos in your blog posts that can be improved with the tools we have today. Make sure all of the visuals used in your content are appealing and high quality.
Update Internal and External Links
Are your internal and external links up to date? They need to be for your SEO and user experience. Outdated links can lead to broken pages or irrelevant content, frustrating readers and hurting your site’s performance.
You need to check for any broken links on your old blog posts, and update them ASAP. Updating your old blog posts can also lead to new opportunities to link internally to other blog posts and pages, which may not have been available when the post was originally published.
Optimize for Conversions
When updating content, the ultimate goal is often to increase conversions. However, your conversion goals may have changed over the years.
So here’s what you need to check in your updated blog post. First, does the call-to-action (CTA) still link to the products or services you want to promote? If not, update it to direct readers to the current solution or offer.
Second, consider where you can use different conversion strategies. Don’t just add a CTA at the end of the post.
Last, make sure that the blog post leverages product-led content. It’s going to help you mention your products and services in a way that feels natural, without being too pushy. Being subtle can be a high ROI tactic for updated posts.
Key Takeaway
Reviving old blog articles for SEO is a powerful strategy that can breathe new life into your content and boost your website’s visibility. Instead of solely focusing on creating new posts, taking the time to refresh existing content can yield impressive results, both in terms of traffic and conversions.
By implementing these strategies, you can transform old blog posts into valuable resources that attract new readers and retain existing ones. So, roll up your sleeves, dive into your archives, and start updating your content today—your audience and search rankings will thank you!
SEO
How Compression Can Be Used To Detect Low Quality Pages
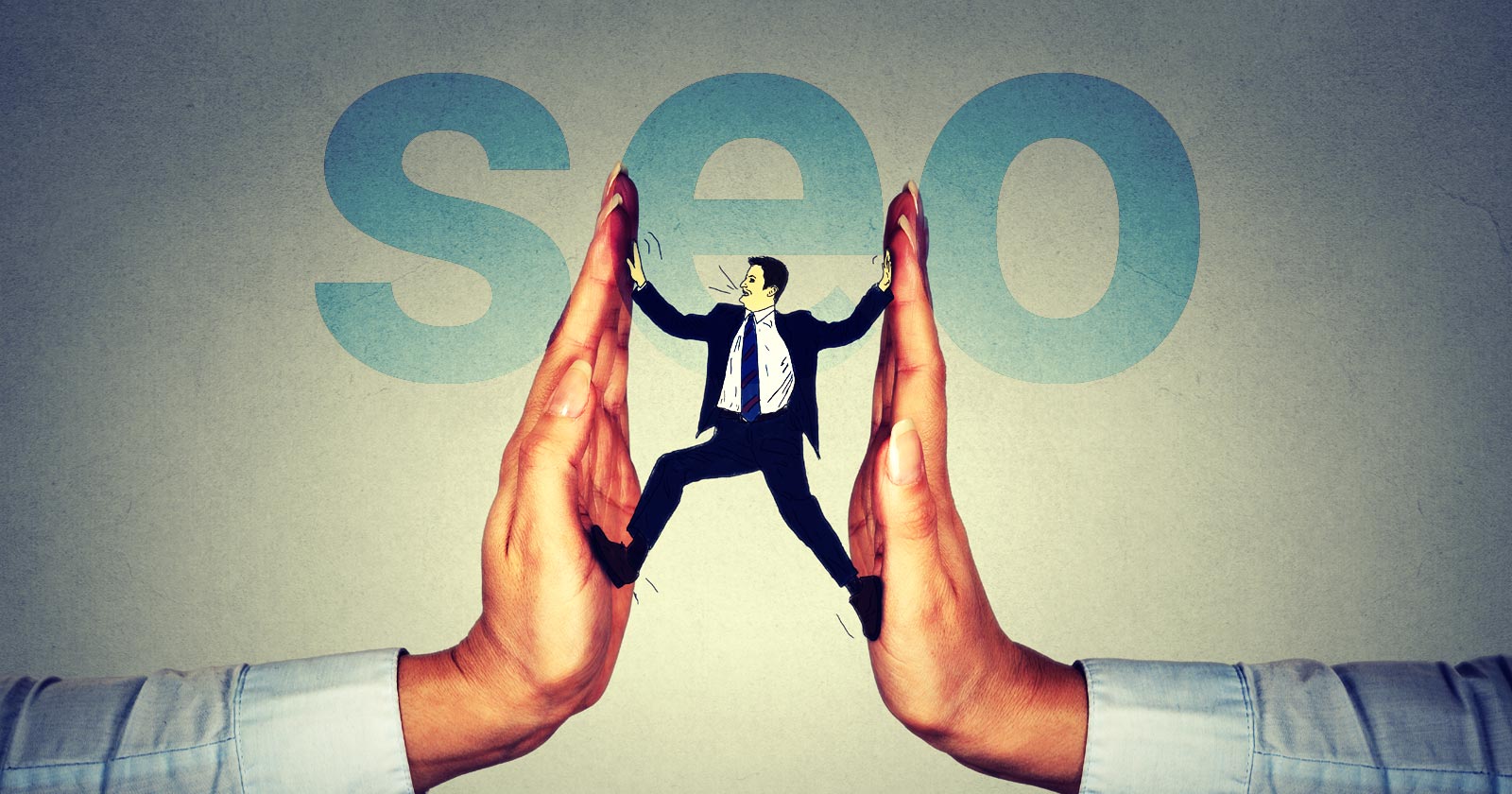
The concept of Compressibility as a quality signal is not widely known, but SEOs should be aware of it. Search engines can use web page compressibility to identify duplicate pages, doorway pages with similar content, and pages with repetitive keywords, making it useful knowledge for SEO.
Although the following research paper demonstrates a successful use of on-page features for detecting spam, the deliberate lack of transparency by search engines makes it difficult to say with certainty if search engines are applying this or similar techniques.
What Is Compressibility?
In computing, compressibility refers to how much a file (data) can be reduced in size while retaining essential information, typically to maximize storage space or to allow more data to be transmitted over the Internet.
TL/DR Of Compression
Compression replaces repeated words and phrases with shorter references, reducing the file size by significant margins. Search engines typically compress indexed web pages to maximize storage space, reduce bandwidth, and improve retrieval speed, among other reasons.
This is a simplified explanation of how compression works:
- Identify Patterns:
A compression algorithm scans the text to find repeated words, patterns and phrases - Shorter Codes Take Up Less Space:
The codes and symbols use less storage space then the original words and phrases, which results in a smaller file size. - Shorter References Use Less Bits:
The “code” that essentially symbolizes the replaced words and phrases uses less data than the originals.
A bonus effect of using compression is that it can also be used to identify duplicate pages, doorway pages with similar content, and pages with repetitive keywords.
Research Paper About Detecting Spam
This research paper is significant because it was authored by distinguished computer scientists known for breakthroughs in AI, distributed computing, information retrieval, and other fields.
Marc Najork
One of the co-authors of the research paper is Marc Najork, a prominent research scientist who currently holds the title of Distinguished Research Scientist at Google DeepMind. He’s a co-author of the papers for TW-BERT, has contributed research for increasing the accuracy of using implicit user feedback like clicks, and worked on creating improved AI-based information retrieval (DSI++: Updating Transformer Memory with New Documents), among many other major breakthroughs in information retrieval.
Dennis Fetterly
Another of the co-authors is Dennis Fetterly, currently a software engineer at Google. He is listed as a co-inventor in a patent for a ranking algorithm that uses links, and is known for his research in distributed computing and information retrieval.
Those are just two of the distinguished researchers listed as co-authors of the 2006 Microsoft research paper about identifying spam through on-page content features. Among the several on-page content features the research paper analyzes is compressibility, which they discovered can be used as a classifier for indicating that a web page is spammy.
Detecting Spam Web Pages Through Content Analysis
Although the research paper was authored in 2006, its findings remain relevant to today.
Then, as now, people attempted to rank hundreds or thousands of location-based web pages that were essentially duplicate content aside from city, region, or state names. Then, as now, SEOs often created web pages for search engines by excessively repeating keywords within titles, meta descriptions, headings, internal anchor text, and within the content to improve rankings.
Section 4.6 of the research paper explains:
“Some search engines give higher weight to pages containing the query keywords several times. For example, for a given query term, a page that contains it ten times may be higher ranked than a page that contains it only once. To take advantage of such engines, some spam pages replicate their content several times in an attempt to rank higher.”
The research paper explains that search engines compress web pages and use the compressed version to reference the original web page. They note that excessive amounts of redundant words results in a higher level of compressibility. So they set about testing if there’s a correlation between a high level of compressibility and spam.
They write:
“Our approach in this section to locating redundant content within a page is to compress the page; to save space and disk time, search engines often compress web pages after indexing them, but before adding them to a page cache.
…We measure the redundancy of web pages by the compression ratio, the size of the uncompressed page divided by the size of the compressed page. We used GZIP …to compress pages, a fast and effective compression algorithm.”
High Compressibility Correlates To Spam
The results of the research showed that web pages with at least a compression ratio of 4.0 tended to be low quality web pages, spam. However, the highest rates of compressibility became less consistent because there were fewer data points, making it harder to interpret.
Figure 9: Prevalence of spam relative to compressibility of page.
The researchers concluded:
“70% of all sampled pages with a compression ratio of at least 4.0 were judged to be spam.”
But they also discovered that using the compression ratio by itself still resulted in false positives, where non-spam pages were incorrectly identified as spam:
“The compression ratio heuristic described in Section 4.6 fared best, correctly identifying 660 (27.9%) of the spam pages in our collection, while misidentifying 2, 068 (12.0%) of all judged pages.
Using all of the aforementioned features, the classification accuracy after the ten-fold cross validation process is encouraging:
95.4% of our judged pages were classified correctly, while 4.6% were classified incorrectly.
More specifically, for the spam class 1, 940 out of the 2, 364 pages, were classified correctly. For the non-spam class, 14, 440 out of the 14,804 pages were classified correctly. Consequently, 788 pages were classified incorrectly.”
The next section describes an interesting discovery about how to increase the accuracy of using on-page signals for identifying spam.
Insight Into Quality Rankings
The research paper examined multiple on-page signals, including compressibility. They discovered that each individual signal (classifier) was able to find some spam but that relying on any one signal on its own resulted in flagging non-spam pages for spam, which are commonly referred to as false positive.
The researchers made an important discovery that everyone interested in SEO should know, which is that using multiple classifiers increased the accuracy of detecting spam and decreased the likelihood of false positives. Just as important, the compressibility signal only identifies one kind of spam but not the full range of spam.
The takeaway is that compressibility is a good way to identify one kind of spam but there are other kinds of spam that aren’t caught with this one signal. Other kinds of spam were not caught with the compressibility signal.
This is the part that every SEO and publisher should be aware of:
“In the previous section, we presented a number of heuristics for assaying spam web pages. That is, we measured several characteristics of web pages, and found ranges of those characteristics which correlated with a page being spam. Nevertheless, when used individually, no technique uncovers most of the spam in our data set without flagging many non-spam pages as spam.
For example, considering the compression ratio heuristic described in Section 4.6, one of our most promising methods, the average probability of spam for ratios of 4.2 and higher is 72%. But only about 1.5% of all pages fall in this range. This number is far below the 13.8% of spam pages that we identified in our data set.”
So, even though compressibility was one of the better signals for identifying spam, it still was unable to uncover the full range of spam within the dataset the researchers used to test the signals.
Combining Multiple Signals
The above results indicated that individual signals of low quality are less accurate. So they tested using multiple signals. What they discovered was that combining multiple on-page signals for detecting spam resulted in a better accuracy rate with less pages misclassified as spam.
The researchers explained that they tested the use of multiple signals:
“One way of combining our heuristic methods is to view the spam detection problem as a classification problem. In this case, we want to create a classification model (or classifier) which, given a web page, will use the page’s features jointly in order to (correctly, we hope) classify it in one of two classes: spam and non-spam.”
These are their conclusions about using multiple signals:
“We have studied various aspects of content-based spam on the web using a real-world data set from the MSNSearch crawler. We have presented a number of heuristic methods for detecting content based spam. Some of our spam detection methods are more effective than others, however when used in isolation our methods may not identify all of the spam pages. For this reason, we combined our spam-detection methods to create a highly accurate C4.5 classifier. Our classifier can correctly identify 86.2% of all spam pages, while flagging very few legitimate pages as spam.”
Key Insight:
Misidentifying “very few legitimate pages as spam” was a significant breakthrough. The important insight that everyone involved with SEO should take away from this is that one signal by itself can result in false positives. Using multiple signals increases the accuracy.
What this means is that SEO tests of isolated ranking or quality signals will not yield reliable results that can be trusted for making strategy or business decisions.
Takeaways
We don’t know for certain if compressibility is used at the search engines but it’s an easy to use signal that combined with others could be used to catch simple kinds of spam like thousands of city name doorway pages with similar content. Yet even if the search engines don’t use this signal, it does show how easy it is to catch that kind of search engine manipulation and that it’s something search engines are well able to handle today.
Here are the key points of this article to keep in mind:
- Doorway pages with duplicate content is easy to catch because they compress at a higher ratio than normal web pages.
- Groups of web pages with a compression ratio above 4.0 were predominantly spam.
- Negative quality signals used by themselves to catch spam can lead to false positives.
- In this particular test, they discovered that on-page negative quality signals only catch specific types of spam.
- When used alone, the compressibility signal only catches redundancy-type spam, fails to detect other forms of spam, and leads to false positives.
- Combing quality signals improves spam detection accuracy and reduces false positives.
- Search engines today have a higher accuracy of spam detection with the use of AI like Spam Brain.
Read the research paper, which is linked from the Google Scholar page of Marc Najork:
Detecting spam web pages through content analysis
Featured Image by Shutterstock/pathdoc
SEO
New Google Trends SEO Documentation
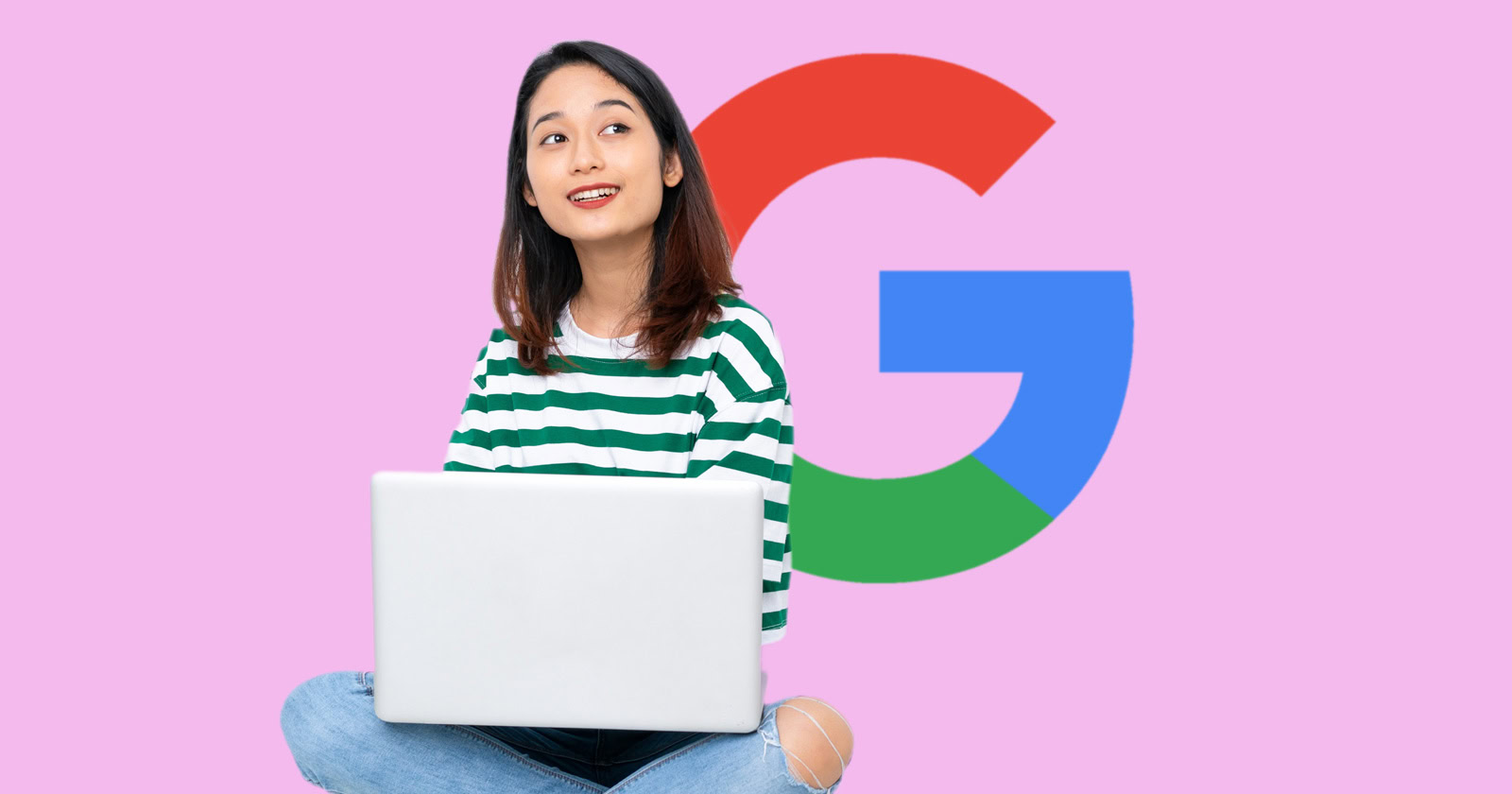
Google Search Central published new documentation on Google Trends, explaining how to use it for search marketing. This guide serves as an easy to understand introduction for newcomers and a helpful refresher for experienced search marketers and publishers.
The new guide has six sections:
- About Google Trends
- Tutorial on monitoring trends
- How to do keyword research with the tool
- How to prioritize content with Trends data
- How to use Google Trends for competitor research
- How to use Google Trends for analyzing brand awareness and sentiment
The section about monitoring trends advises there are two kinds of rising trends, general and specific trends, which can be useful for developing content to publish on a site.
Using the Explore tool, you can leave the search box empty and view the current rising trends worldwide or use a drop down menu to focus on trends in a specific country. Users can further filter rising trends by time periods, categories and the type of search. The results show rising trends by topic and by keywords.
To search for specific trends users just need to enter the specific queries and then filter them by country, time, categories and type of search.
The section called Content Calendar describes how to use Google Trends to understand which content topics to prioritize.
Google explains:
“Google Trends can be helpful not only to get ideas on what to write, but also to prioritize when to publish it. To help you better prioritize which topics to focus on, try to find seasonal trends in the data. With that information, you can plan ahead to have high quality content available on your site a little before people are searching for it, so that when they do, your content is ready for them.”
Read the new Google Trends documentation:
Get started with Google Trends
Featured Image by Shutterstock/Luis Molinero
You must be logged in to post a comment Login