SEO
How To Visualize & Customize Backlink Analysis With Python
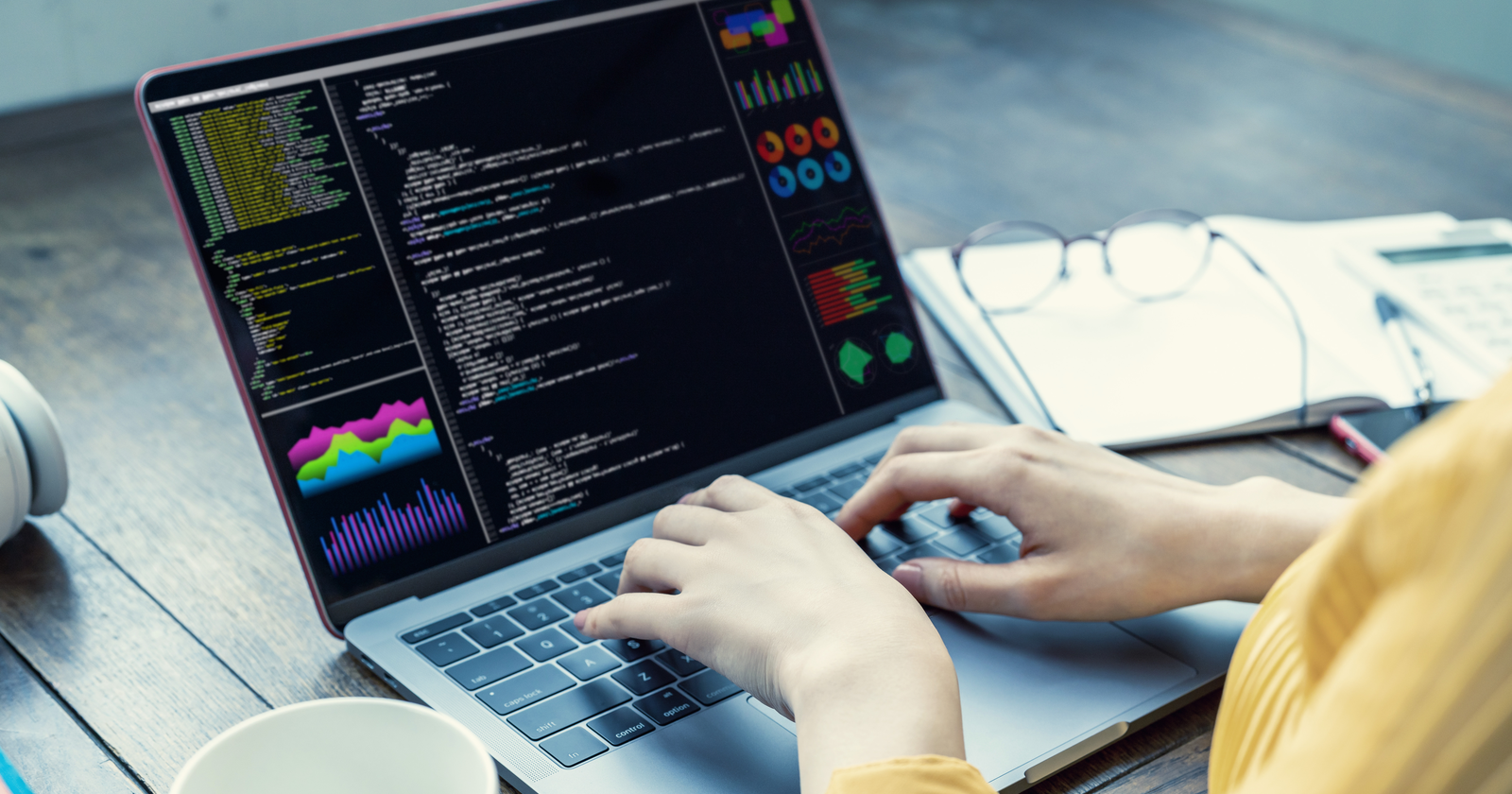
Chances are, you’ve used one of the more popular tools such as Ahrefs or Semrush to analyze your site’s backlinks.
These tools trawl the web to get a list of sites linking to your website with a domain rating and other data describing the quality of your backlinks.
It’s no secret that backlinks play a big part in Google’s algorithm, so it makes sense as a minimum to understand your own site before comparing it with the competition.
While using tools gives you insight into specific metrics, learning to analyze backlinks on your own gives you more flexibility into what it is you’re measuring and how it’s presented.
And although you could do most of the analysis on a spreadsheet, Python has certain advantages.
Other than the sheer number of rows it can handle, it can also more readily look at the statistical side, such as distributions.
In this column, you’ll find step-by-step instructions on how to visualize basic backlink analysis and customize your reports by considering different link attributes using Python.
Not Taking A Seat
We’re going to pick a small website from the U.K. furniture sector as an example and walk through some basic analysis using Python.
So what is the value of a site’s backlinks for SEO?
At its simplest, I’d say quality and quantity.
Quality is subjective to the expert yet definitive to Google by way of metrics such as authority and content relevance.
We’ll start by evaluating the link quality with the available data before evaluating the quantity.
Time to code.
import re import time import random import pandas as pd import numpy as np import datetime from datetime import timedelta from plotnine import * import matplotlib.pyplot as plt from pandas.api.types import is_string_dtype from pandas.api.types import is_numeric_dtype import uritools pd.set_option('display.max_colwidth', None) %matplotlib inline root_domain = 'johnsankey.co.uk' hostdomain = 'www.johnsankey.co.uk' hostname="johnsankey" full_domain = 'https://www.johnsankey.co.uk' target_name="John Sankey"
We start by importing the data and cleaning up the column names to make it easier to handle and quicker to type for the later stages.
target_ahrefs_raw = pd.read_csv( 'data/johnsankey.co.uk-refdomains-subdomains__2022-03-18_15-15-47.csv')
List comprehensions are a powerful and less intensive way to clean up the column names.
target_ahrefs_raw.columns = [col.lower() for col in target_ahrefs_raw.columns]
The list comprehension instructs Python to convert the column name to lower case for each column (‘col’) in the dataframe’s columns.
target_ahrefs_raw.columns = [col.replace(' ','_') for col in target_ahrefs_raw.columns] target_ahrefs_raw.columns = [col.replace('.','_') for col in target_ahrefs_raw.columns] target_ahrefs_raw.columns = [col.replace('__','_') for col in target_ahrefs_raw.columns] target_ahrefs_raw.columns = [col.replace('(','') for col in target_ahrefs_raw.columns] target_ahrefs_raw.columns = [col.replace(')','') for col in target_ahrefs_raw.columns] target_ahrefs_raw.columns = [col.replace('%','') for col in target_ahrefs_raw.columns]
Though not strictly necessary, I like having a count column as standard for aggregations and a single value column “project” should I need to group the entire table.
target_ahrefs_raw['rd_count'] = 1 target_ahrefs_raw['project'] = target_name target_ahrefs_raw
-
Screenshot from Pandas, March 2022
Now we have a dataframe with clean column names.
The next step is to clean the actual table values and make them more useful for analysis.
Make a copy of the previous dataframe and give it a new name.
target_ahrefs_clean_dtypes = target_ahrefs_raw
Clean the dofollow_ref_domains column, which tells us how many ref domains the site linking has.
In this case, we’ll convert the dashes to zeroes and then cast the whole column as a whole number.
# referring_domains target_ahrefs_clean_dtypes['dofollow_ref_domains'] = np.where(target_ahrefs_clean_dtypes['dofollow_ref_domains'] == '-', 0, target_ahrefs_clean_dtypes['dofollow_ref_domains']) target_ahrefs_clean_dtypes['dofollow_ref_domains'] = target_ahrefs_clean_dtypes['dofollow_ref_domains'].astype(int) # linked_domains target_ahrefs_clean_dtypes['dofollow_linked_domains'] = np.where(target_ahrefs_clean_dtypes['dofollow_linked_domains'] == '-', 0, target_ahrefs_clean_dtypes['dofollow_linked_domains']) target_ahrefs_clean_dtypes['dofollow_linked_domains'] = target_ahrefs_clean_dtypes['dofollow_linked_domains'].astype(int)
First_seen tells us the date the link was first found.
We’ll convert the string to a date format that Python can process and then use this to derive the age of the links later on.
# first_seen target_ahrefs_clean_dtypes['first_seen'] = pd.to_datetime(target_ahrefs_clean_dtypes['first_seen'], format="%d/%m/%Y %H:%M")
Converting first_seen to a date also means we can perform time aggregations by month and year.
This is useful as it’s not always the case that links for a site will get acquired daily, although it would be nice for my own site if it did!
target_ahrefs_clean_dtypes['month_year'] = target_ahrefs_clean_dtypes['first_seen'].dt.to_period('M')
The link age is calculated by taking today’s date and subtracting the first_seen date.
Then it’s converted to a number format and divided by a huge number to get the number of days.
# link age target_ahrefs_clean_dtypes['link_age'] = datetime.datetime.now() - target_ahrefs_clean_dtypes['first_seen'] target_ahrefs_clean_dtypes['link_age'] = target_ahrefs_clean_dtypes['link_age'] target_ahrefs_clean_dtypes['link_age'] = target_ahrefs_clean_dtypes['link_age'].astype(int) target_ahrefs_clean_dtypes['link_age'] = (target_ahrefs_clean_dtypes['link_age']/(3600 * 24 * 1000000000)).round(0) target_ahrefs_clean_dtypes

With the data types cleaned, and some new data features created, the fun can begin!
Link Quality
The first part of our analysis evaluates link quality, which summarizes the whole dataframe using the describe function to get descriptive statistics of all the columns.
target_ahrefs_analysis = target_ahrefs_clean_dtypes target_ahrefs_analysis.describe()

So from the above table, we can see the average (mean), the number of referring domains (107), and the variation (the 25th percentile and so on).
The average Domain Rating (equivalent to Moz’s Domain Authority) of referring domains is 27.
Is that a good thing?
In the absence of competitor data to compare in this market sector, it’s hard to know. This is where your experience as an SEO practitioner comes in.
However, I’m certain we could all agree that it could be higher.
How much higher to make a shift is another question.

The table above can be a bit dry and hard to visualize, so we’ll plot a histogram to get an intuitive understanding of the referring domain’s authority.
dr_dist_plt = ( ggplot(target_ahrefs_analysis, aes(x = 'dr')) + geom_histogram(alpha = 0.6, fill="blue", bins = 100) + scale_y_continuous() + theme(legend_position = 'right')) dr_dist_plt

The distribution is heavily skewed, showing that most of the referring domains have an authority rating of zero.
Beyond zero, the distribution looks fairly uniform, with an equal amount of domains across different levels of authority.
Link age is another important factor for SEO.
Let’s check out the distribution below.
linkage_dist_plt = ( ggplot(target_ahrefs_analysis, aes(x = 'link_age')) + geom_histogram(alpha = 0.6, fill="blue", bins = 100) + scale_y_continuous() + theme(legend_position = 'right')) linkage_dist_plt

The distribution looks more normal even if it is still skewed with the majority of the links being new.
The most common link age appears to be around 200 days, which is less than a year, suggesting most of the links were acquired recently.
Out of interest, let’s see how this correlates with domain authority.
dr_linkage_plt = ( ggplot(target_ahrefs_analysis, aes(x = 'dr', y = 'link_age')) + geom_point(alpha = 0.4, colour="blue", size = 2) + geom_smooth(method = 'lm', se = False, colour="red", size = 3, alpha = 0.4) ) print(target_ahrefs_analysis['dr'].corr(target_ahrefs_analysis['link_age'])) dr_linkage_plt 0.1941101232345909

The plot (along with the 0.19 figure printed above) shows no correlation between the two.
And why should there be?
A correlation would only imply that the higher authority links were acquired in the early phase of the site’s history.
The reason for the non-correlation will become more apparent later on.
We’ll now look at the link quality throughout time.
If we were to literally plot the number of links by date, the time series would look rather messy and less useful as shown below (no code supplied to render the chart).
To achieve this, we will calculate a running average of the Domain Rating by month of the year.
Note the expanding( ) function, which instructs Pandas to include all previous rows with each new row.
target_rd_cummean_df = target_ahrefs_analysis target_rd_mean_df = target_rd_cummean_df.groupby(['month_year'])['dr'].sum().reset_index() target_rd_mean_df['dr_runavg'] = target_rd_mean_df['dr'].expanding().mean() target_rd_mean_df

We now have a table that we can use to feed the graph and visualize it.
dr_cummean_smooth_plt = ( ggplot(target_rd_mean_df, aes(x = 'month_year', y = 'dr_runavg', group = 1)) + geom_line(alpha = 0.6, colour="blue", size = 2) + scale_y_continuous() + scale_x_date() + theme(legend_position = 'right', axis_text_x=element_text(rotation=90, hjust=1) ))
dr_cummean_smooth_plt

This is quite interesting as it seems the site started off attracting high authority links at the beginning of its time (probably a PR campaign launching the business).
It then faded for four years before reprising with a new link acquisition of high authority links again.
Volume Of Links
It sounds good just writing that heading!
Who wouldn’t want a large volume of (good) links to their site?
Quality is one thing; volume is another, which is what we’ll analyze next.
Much like the previous operation, we’ll use the expanding function to calculate a cumulative sum of the links acquired to date.
target_count_cumsum_df = target_ahrefs_analysis target_count_cumsum_df = target_count_cumsum_df.groupby(['month_year'])['rd_count'].sum().reset_index() target_count_cumsum_df['count_runsum'] = target_count_cumsum_df['rd_count'].expanding().sum() target_count_cumsum_df

That’s the data, now the graph.
target_count_cumsum_plt = ( ggplot(target_count_cumsum_df, aes(x = 'month_year', y = 'count_runsum', group = 1)) + geom_line(alpha = 0.6, colour="blue", size = 2) + scale_y_continuous() + scale_x_date() + theme(legend_position = 'right', axis_text_x=element_text(rotation=90, hjust=1) )) target_count_cumsum_plt

We see that links acquired at the beginning of 2017 slowed down but steadily added over the next four years before accelerating again around March 2021.
Again, it would be good to correlate that with performance.
Taking It Further
Of course, the above is just the tip of the iceberg, as it’s a simple exploration of one site. It’s difficult to infer anything useful for improving rankings in competitive search spaces.
Below are some areas for further data exploration and analysis.
- Adding social media share data to both the destination URLs.
- Correlating overall site visibility with the running average DR over time.
- Plotting the distribution of DR over time.
- Adding search volume data on the host names to see how many brand searches the referring domains receive as a measure of true authority.
- Joining with crawl data to the destination URLs to test for content relevance.
- Link velocity – the rate at which new links from new sites are acquired.
- Integrating all of the above ideas into your analysis to compare to your competitors.
I’m certain there are plenty of ideas not listed above, feel free to share below.
More resources:
Featured Image: metamorworks/Shutterstock
SEO
Google Declares It The “Gemini Era” As Revenue Grows 15%
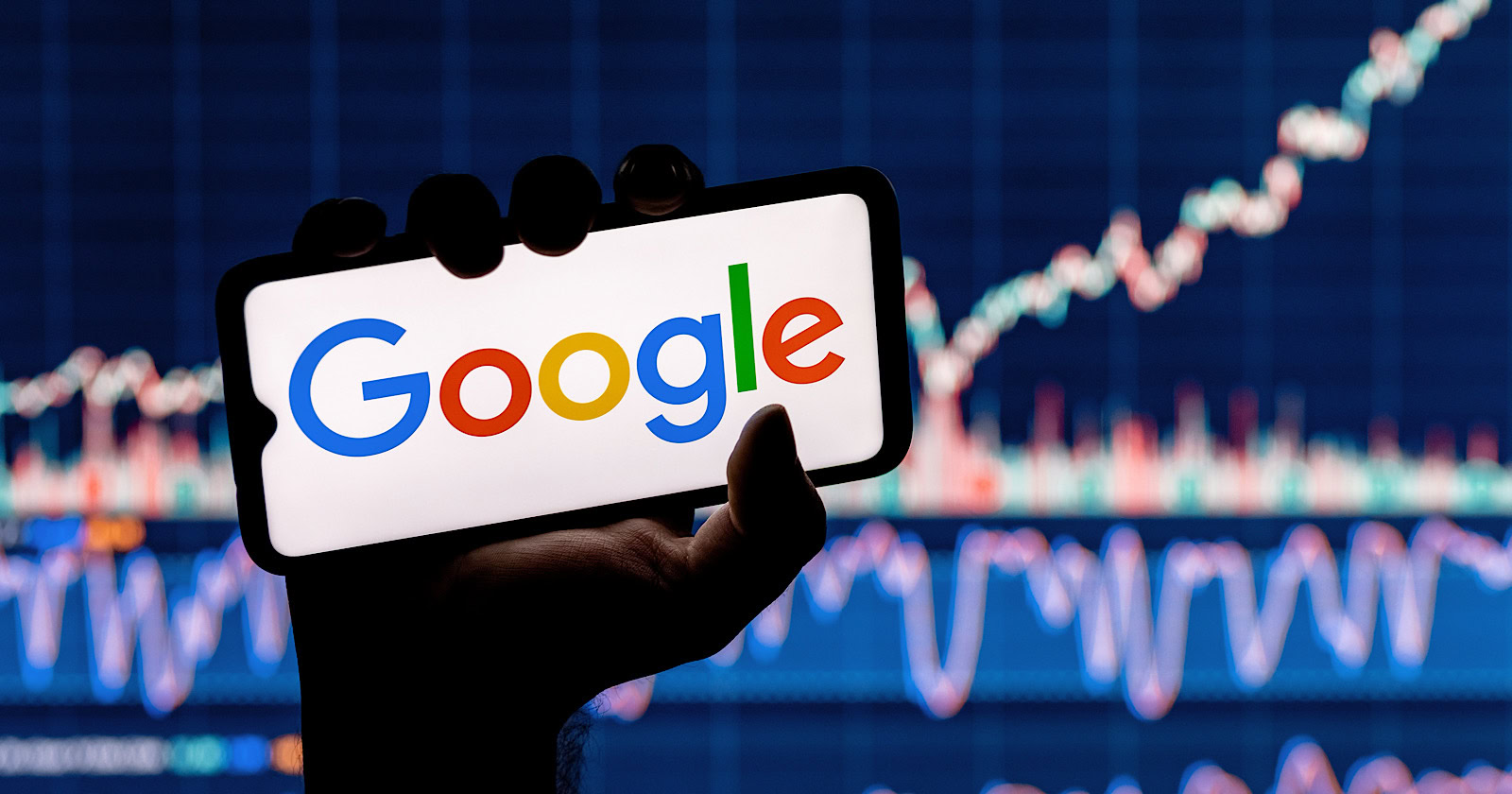
Alphabet Inc., Google’s parent company, announced its first quarter 2024 financial results today.
While Google reported double-digit growth in key revenue areas, the focus was on its AI developments, dubbed the “Gemini era” by CEO Sundar Pichai.
The Numbers: 15% Revenue Growth, Operating Margins Expand
Alphabet reported Q1 revenues of $80.5 billion, a 15% increase year-over-year, exceeding Wall Street’s projections.
Net income was $23.7 billion, with diluted earnings per share of $1.89. Operating margins expanded to 32%, up from 25% in the prior year.
Ruth Porat, Alphabet’s President and CFO, stated:
“Our strong financial results reflect revenue strength across the company and ongoing efforts to durably reengineer our cost base.”
Google’s core advertising units, such as Search and YouTube, drove growth. Google advertising revenues hit $61.7 billion for the quarter.
The Cloud division also maintained momentum, with revenues of $9.6 billion, up 28% year-over-year.
Pichai highlighted that YouTube and Cloud are expected to exit 2024 at a combined $100 billion annual revenue run rate.
Generative AI Integration in Search
Google experimented with AI-powered features in Search Labs before recently introducing AI overviews into the main search results page.
Regarding the gradual rollout, Pichai states:
“We are being measured in how we do this, focusing on areas where gen AI can improve the Search experience, while also prioritizing traffic to websites and merchants.”
Pichai reports that Google’s generative AI features have answered over a billion queries already:
“We’ve already served billions of queries with our generative AI features. It’s enabling people to access new information, to ask questions in new ways, and to ask more complex questions.”
Google reports increased Search usage and user satisfaction among those interacting with the new AI overview results.
The company also highlighted its “Circle to Search” feature on Android, which allows users to circle objects on their screen or in videos to get instant AI-powered answers via Google Lens.
Reorganizing For The “Gemini Era”
As part of the AI roadmap, Alphabet is consolidating all teams building AI models under the Google DeepMind umbrella.
Pichai revealed that, through hardware and software improvements, the company has reduced machine costs associated with its generative AI search results by 80% over the past year.
He states:
“Our data centers are some of the most high-performing, secure, reliable and efficient in the world. We’ve developed new AI models and algorithms that are more than one hundred times more efficient than they were 18 months ago.
How Will Google Make Money With AI?
Alphabet sees opportunities to monetize AI through its advertising products, Cloud offerings, and subscription services.
Google is integrating Gemini into ad products like Performance Max. The company’s Cloud division is bringing “the best of Google AI” to enterprise customers worldwide.
Google One, the company’s subscription service, surpassed 100 million paid subscribers in Q1 and introduced a new premium plan featuring advanced generative AI capabilities powered by Gemini models.
Future Outlook
Pichai outlined six key advantages positioning Alphabet to lead the “next wave of AI innovation”:
- Research leadership in AI breakthroughs like the multimodal Gemini model
- Robust AI infrastructure and custom TPU chips
- Integrating generative AI into Search to enhance the user experience
- A global product footprint reaching billions
- Streamlined teams and improved execution velocity
- Multiple revenue streams to monetize AI through advertising and cloud
With upcoming events like Google I/O and Google Marketing Live, the company is expected to share further updates on its AI initiatives and product roadmap.
Featured Image: Sergei Elagin/Shutterstock
SEO
brightonSEO Live Blog
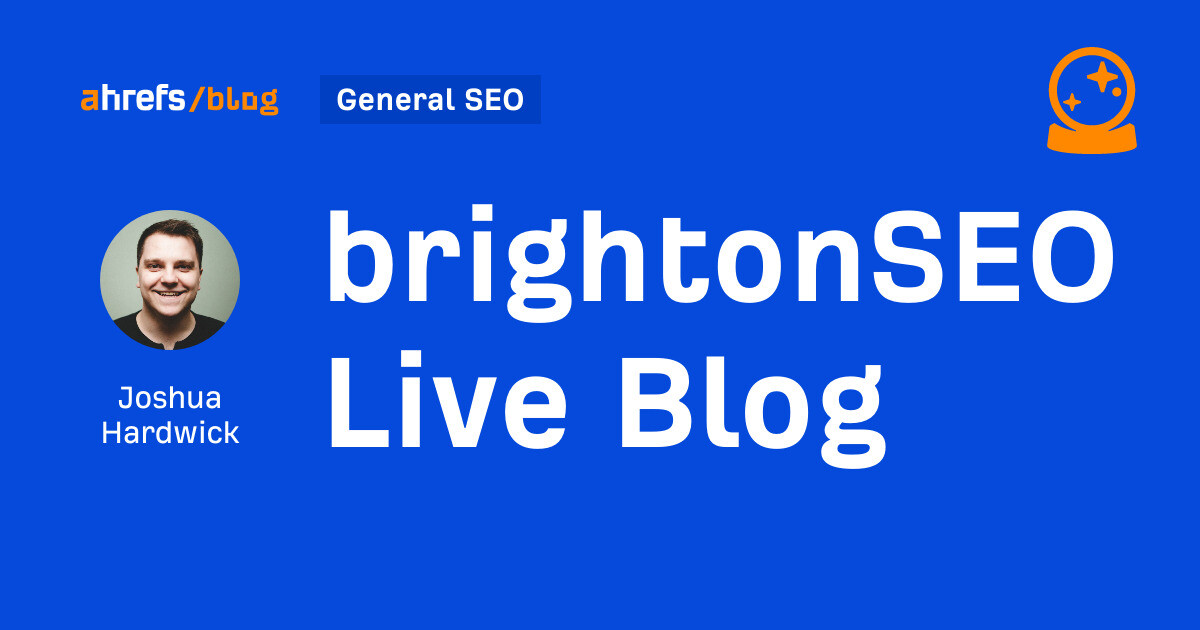
Hello everyone. It’s April again, so I’m back in Brighton for another two days of Being the introvert I am, my idea of fun isn’t hanging around our booth all day explaining we’ve run out of t-shirts (seriously, you need to be fast if you want swag!). So I decided to do something useful and live-blog the event instead.
Follow below for talk takeaways and (very) mildly humorous commentary. sun, sea, and SEO!
SEO
Google Further Postpones Third-Party Cookie Deprecation In Chrome
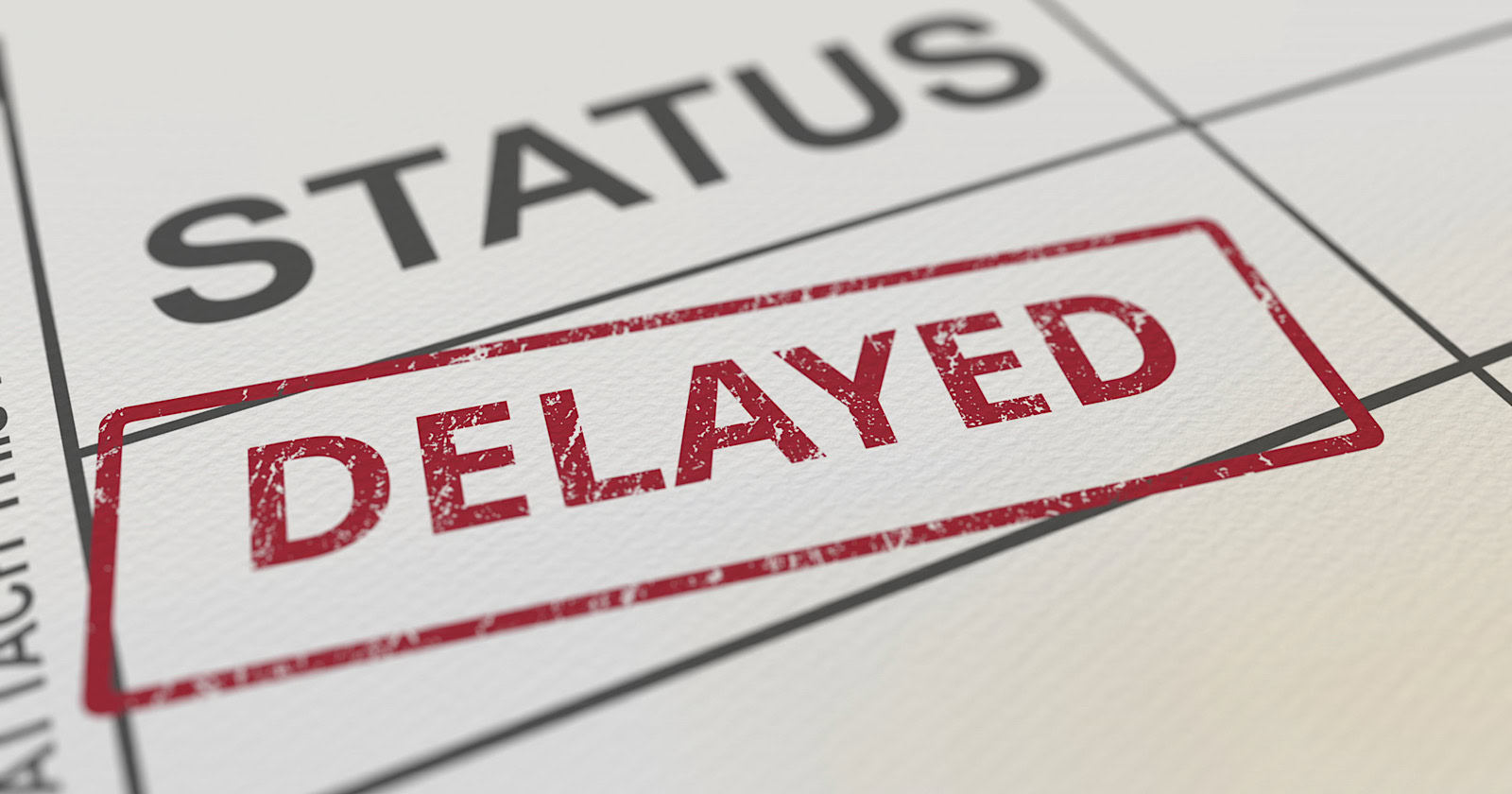
Google has again delayed its plan to phase out third-party cookies in the Chrome web browser. The latest postponement comes after ongoing challenges in reconciling feedback from industry stakeholders and regulators.
The announcement was made in Google and the UK’s Competition and Markets Authority (CMA) joint quarterly report on the Privacy Sandbox initiative, scheduled for release on April 26.
Chrome’s Third-Party Cookie Phaseout Pushed To 2025
Google states it “will not complete third-party cookie deprecation during the second half of Q4” this year as planned.
Instead, the tech giant aims to begin deprecating third-party cookies in Chrome “starting early next year,” assuming an agreement can be reached with the CMA and the UK’s Information Commissioner’s Office (ICO).
The statement reads:
“We recognize that there are ongoing challenges related to reconciling divergent feedback from the industry, regulators and developers, and will continue to engage closely with the entire ecosystem. It’s also critical that the CMA has sufficient time to review all evidence, including results from industry tests, which the CMA has asked market participants to provide by the end of June.”
Continued Engagement With Regulators
Google reiterated its commitment to “engaging closely with the CMA and ICO” throughout the process and hopes to conclude discussions this year.
This marks the third delay to Google’s plan to deprecate third-party cookies, initially aiming for a Q3 2023 phaseout before pushing it back to late 2024.
The postponements reflect the challenges in transitioning away from cross-site user tracking while balancing privacy and advertiser interests.
Transition Period & Impact
In January, Chrome began restricting third-party cookie access for 1% of users globally. This percentage was expected to gradually increase until 100% of users were covered by Q3 2024.
However, the latest delay gives websites and services more time to migrate away from third-party cookie dependencies through Google’s limited “deprecation trials” program.
The trials offer temporary cookie access extensions until December 27, 2024, for non-advertising use cases that can demonstrate direct user impact and functional breakage.
While easing the transition, the trials have strict eligibility rules. Advertising-related services are ineligible, and origins matching known ad-related domains are rejected.
Google states the program aims to address functional issues rather than relieve general data collection inconveniences.
Publisher & Advertiser Implications
The repeated delays highlight the potential disruption for digital publishers and advertisers relying on third-party cookie tracking.
Industry groups have raised concerns that restricting cross-site tracking could push websites toward more opaque privacy-invasive practices.
However, privacy advocates view the phaseout as crucial in preventing covert user profiling across the web.
With the latest postponement, all parties have more time to prepare for the eventual loss of third-party cookies and adopt Google’s proposed Privacy Sandbox APIs as replacements.
Featured Image: Novikov Aleksey/Shutterstock
-
PPC7 days ago
19 Best SEO Tools in 2024 (For Every Use Case)
-
SEARCHENGINES6 days ago
Daily Search Forum Recap: April 19, 2024
-
WORDPRESS7 days ago
How to Make $5000 of Passive Income Every Month in WordPress
-
WORDPRESS5 days ago
13 Best HubSpot Alternatives for 2024 (Free + Paid)
-
MARKETING6 days ago
Battling for Attention in the 2024 Election Year Media Frenzy
-
SEO7 days ago
25 WordPress Alternatives Best For SEO
-
WORDPRESS6 days ago
7 Best WooCommerce Points and Rewards Plugins (Free & Paid)
-
AFFILIATE MARKETING7 days ago
AI Will Transform the Workplace. Here’s How HR Can Prepare for It.
You must be logged in to post a comment Login