SEO
How To Automate Ecommerce Category Page Creation With Python
Clustering product inventory and automatically aligning SKUs to search demand is a great way to find opportunities to create new ecommerce categories.
Niche category pages are a proven way for ecommerce sites to align with organic search demand while simultaneously assisting users in purchasing.
If a site stocks a range of products and there is search demand, creating a dedicated landing page is an easy way to align with the demand.
But how can SEO professionals find this opportunity?
Sure, you can eyeball it, but you’ll usually leave a lot of opportunity on the table.
This problem motivated me to script something in Python, which I’m sharing today in a simple to use Streamlit application. (No coding experience required!)
The app linked above created the following output automatically using nothing more than two crawl exports!
-
Screenshot from Microsoft Excel, May 2022
Notice how the suggested categories are automatically tied back to the existing parent category?
-
Screenshot from Microsoft Excel, May 2022
The app even shows how many products are available to populate the category.
-
Screenshot from Microsoft Excel, May 2022
Benefits And Uses
- Improve relevancy to high-demand, competitive queries by creating new landing pages.
- Increase the chance of relevant site links displaying underneath the parent category.
- Reduce CPCs to the landing page through increased relevancy.
- Potential to inform merchandising decisions. (If there is high search demand vs. low product count – there is a potential to widen the range.0
-
Mock-up Screenshot from Google Chrome, May 2022
-
Creating the suggested subcategories for the parent sofa category would align the site to an additional 3,500 searches per month with relatively little effort.
Features
- Create subcategory suggestions automatically.
- Tie subcategories back to the parent category (cuts out a lot of guesswork!).
- Match to a minimum of X products before recommending a category.
- Check similarity to an existing category (X % fuzzy match) before recommending a new category.
- Set minimum search volume/CPC cut-off for category suggestions.
- Supports search volume and CPC data from multiple countries.
Getting Started/Prepping The Files
To use this app you need two things.
At a high level, the goal is to crawl the target website with two custom extractions.
The internal_html.csv report is exported, along with an inlinks.csv export.
These exports are then uploaded to the Streamlit app, where the opportunities are processed.
Crawl And Extraction Setup
When crawling the site, you’ll need to set two extractions in Screaming Frog – one to uniquely identify product pages and another to uniquely identify category pages.
The Streamlit app understands the difference between the two types of pages when making recommendations for new pages.
The trick is to find a unique element for each page type.
(For a product page, this is usually the price or the returns policy, and for a category page, it’s usually a filter sort element.)
Extracting The Unique Page Elements
Screaming Frog allows for custom extractions of content or code from a web page when crawled.
This section may be daunting if you are unfamiliar with custom extractions, but it’s essential for getting the correct data into the Streamlit app.
The goal is to end up with something looking like the below image.
(A unique extraction for product and category pages with no overlap.)
-
Screenshot from Screaming Frog SEO Spider, May 2022
The steps below walk you through manually extracting the price element for a product page.
Then, repeat for a category page afterward.
If you’re stuck or would like to read more about the web scraper tool in Screaming Frog, the official documentation is worth your time.
Manually Extracting Page Elements
Let’s start by extracting a unique element only found on a product page (usually the price).
Highlight the price element on the page with the mouse, right-click and choose Inspect.
-
Screenshot from Google Chrome, May 2022
This will open up the elements window with the correct HTML line already selected.
Right-click the pre-selected line and choose Copy > Copy selector. That’s it!
-
Screenshot from Google Chrome, May 2022
Open Screaming Frog and paste the copied selector into the custom extraction section. (Configuration > Custom > Extraction).
-
Screenshot from Screaming Frog SEO Spider, May 2022
Name the extractor as “product,” select the CSSPath drop down and choose Extract Text.
Repeat the process to extract a unique element from a category page. It should look like this once completed for both product and category pages.
-
Screenshot from Screaming Frog SEO Spider, May 2022
Finally, start the crawl.
The crawl should look like this when viewing the Custom Extraction tab.
-
Screenshot from Screaming Frog SEO Spider, May 2022
Notice how the extractions are unique to each page type? Perfect.
The script uses the extractor to identify the page type.
Internally the app will convert the extractor to tags.
(I mention this to stress that the extractors can be anything as long as they uniquely identify both page types.)
-
Screenshot from Microsoft Excel, May 2022
Exporting The Files
Once the crawl has been completed, the last step is to export two types of CSV files.
- internal_html.csv.
- inlinks to product pages.
Go to the Custom Extraction tab in Screaming Frog and highlight all URLs that have an extraction for products.
(You will need to sort the column to group it.)
-
Screenshot from Screaming Frog SEO Spider, May 2022
Lastly, right-click the product URLs, select Export, and then Inlinks.
-
Screenshot from Screaming Frog SEO Spider, May 2022
You should now have a file called inlinks.csv.
Finally, we just need to export the internal_html.csv file.
Click the Internal tab, select HTML from the dropdown menu below and click on the adjacent Export button.
Finally, choose the option to save the file as a .csv
-
Screenshot from Screaming Frog SEO Spider, May 2022
Congratulations! You are now ready to use the Streamlit app!
Using The Streamlit App
Using the Streamlit app is relatively simple.
The various options are set to reasonable defaults, but feel free to adjust the cut-offs to better suit your needs.
I would highly recommend using a Keywords Everywhere API key (although it is not strictly necessary as this can be looked up manually later with an existing tool if preferred.
(The script pre-qualifies opportunity by checking for search volume. If the key is missing, the final output will contain more irrelevant words.)
If you want to use a key, this is the section on the left to pay attention to.
-
Screenshot from Streamlit.io, May 2022
Once you have entered the API key and adjusted the cut-offs to your links, upload the inlinks.csv crawl.
-
Screenshot from Streamlit.io, May 2022
Once complete, a new prompt will appear adjacent to it, prompting you to upload the internal_html.csv crawl file.
-
Screenshot from Streamlit.io, May 2022
Finally, a new box will appear asking you to select the product and column names from the uploaded crawl file to be mapped correctly.
-
Screenshot from Streamlit.io, May 2022
Click submit and the script will run. Once complete, you will see the following screen and can download a handy .csv export.
-
Screenshot from Streamlit.io, May 2022
How The Script Works
Before we dive into the script’s output, it will help to explain what’s going on under the hood at a high level.
At a glance:
- Generate thousands of keywords by generating n-grams from product page H1 headings.
- Qualify keywords by checking whether the word is in an exact or fuzzy match in a product heading.
- Further qualify keywords by checking for search volume using the Keywords Everywhere API (optional but recommended).
- Check whether an existing category already exists using a fuzzy match (can find words out of order, different tenses, etc.).
- Uses the inlinks report to assign suggestions to a parent category automatically.
N-gram Generation
The script creates hundreds of thousands of n-grams from the product page H1s, most of which are completely nonsensical.
In my example for this article, n-grams generated 48,307 words – so this will need to be filtered!
-
Screenshot from Microsoft Excel, May 2022
The first step in the filtering process is to check whether the keywords generated via n-grams are found at least X times within the product name column.
(This can be in an exact or fuzzy match.)
Anything not found is immediately discarded, which usually removes around 90% of the generated keywords.
The second filtering stage is to check whether the remaining keywords have search demand.
Any keywords without search demand are then discarded too.
(This is why I recommend using the Keywords Everywhere API when running the script, which results in a more refined output.)
It’s worth noting you can do this manually afterward by searching Semrush/Ahrefs etc., discarding any keywords without search volume, and running a VLOOKUP in Microsoft Excel.
Cheaper if you have an existing subscription.
Recommendations Tied To Specific Landing Pages
Once the keyword list has been filtered the script uses the inlinks report to tie the suggested subcategory back to the landing page.
Earlier versions did not do this, but I realized that leveraging the inlinks.csv report meant it was possible.
It really helps understand the context of the suggestion at a glance during QA.
This is the reason the script requires two exports to work correctly.
Limitations
- Not checking search volumes will result in more results for QA. (Even if you don’t use the Keywords Everywhere API, I recommend shortlisting by filtering out 0 search volume afterward.)
- Some irrelevant keywords will have search volume and appear in the final report, even if keyword volume has been checked.
- Words will typically appear in the singular sense for the final output (because products are singular and categories are pluralized if they sell more than a single product). It’s easy enough to add an “s” to the end of the suggestion though.
User Configurable Variables
I’ve selected what I consider to be sensible default options.
But here is a run down if you’d like to tweak and experiment.
- Minimum products to match to (exact match) – The minimum number of products that must exist before suggesting the new category in an exact match.
- Minimum products to match to (fuzzy match) – The minimum number of products that must exist before suggesting the new category in a fuzzy match, (words can be found in any order).
- Minimum similarity to an existing category – This checks whether a category already exists in a fuzzy match before making the recommendation. The closer to 100 = stricter matching.
- Minimum CPC in $ – The minimum dollar amount of the suggested category keyword. (Requires the Keywords Everywhere API.)
- Minimum search volume – The minimum search volume of the suggested category keyword. (Requires Keywords Everywhere API.)
- Keywords Everywhere API key – Optional, but recommended. Used to pull in CPC/search volume data. (Useful for shortlisting categories.)
- Set the country to pull search data from – Country-specific search data is available. (Default is the USA.)
- Set the currency for CPC data – Country-specific CPC data is available. (Default USD.)
- Keep the longest word suggestion – With similar word suggestions, this option will keep the longest match.
- Enable fuzzy product matching – This will search for product names in a fuzzy match. (Words can be found out of order, recommended – but slow and CPU intensive.)
Conclusion
With a small amount of preparation, it is possible to tap into a large amount of organic opportunity while improving the user experience.
Although this script was created with an ecommerce focus, according to feedback, it works well for other site types such as job listing sites.
So even if your site isn’t an ecommerce site, it’s still worth a try.
Python enthusiast?
I released the source code for a non-Streamlit version here.
More resources:
Featured Image: patpitchaya/Shutterstock
!function(f,b,e,v,n,t,s)
{if(f.fbq)return;n=f.fbq=function(){n.callMethod?
n.callMethod.apply(n,arguments):n.queue.push(arguments)};
if(!f._fbq)f._fbq=n;n.push=n;n.loaded=!0;n.version=’2.0′;
n.queue=[];t=b.createElement(e);t.async=!0;
t.src=v;s=b.getElementsByTagName(e)[0];
s.parentNode.insertBefore(t,s)}(window,document,’script’,
‘https://connect.facebook.net/en_US/fbevents.js’);
if( typeof sopp !== “undefined” && sopp === ‘yes’ ){
fbq(‘dataProcessingOptions’, [‘LDU’], 1, 1000);
}else{
fbq(‘dataProcessingOptions’, []);
}
fbq(‘init’, ‘1321385257908563’);
fbq(‘track’, ‘PageView’);
fbq(‘trackSingle’, ‘1321385257908563’, ‘ViewContent’, {
content_name: ‘python-ecommerce-category-pages’,
content_category: ‘ecommerce technical-seo’
});
SEO
OpenAI Expected to Integrate Real-Time Data In ChatGPT
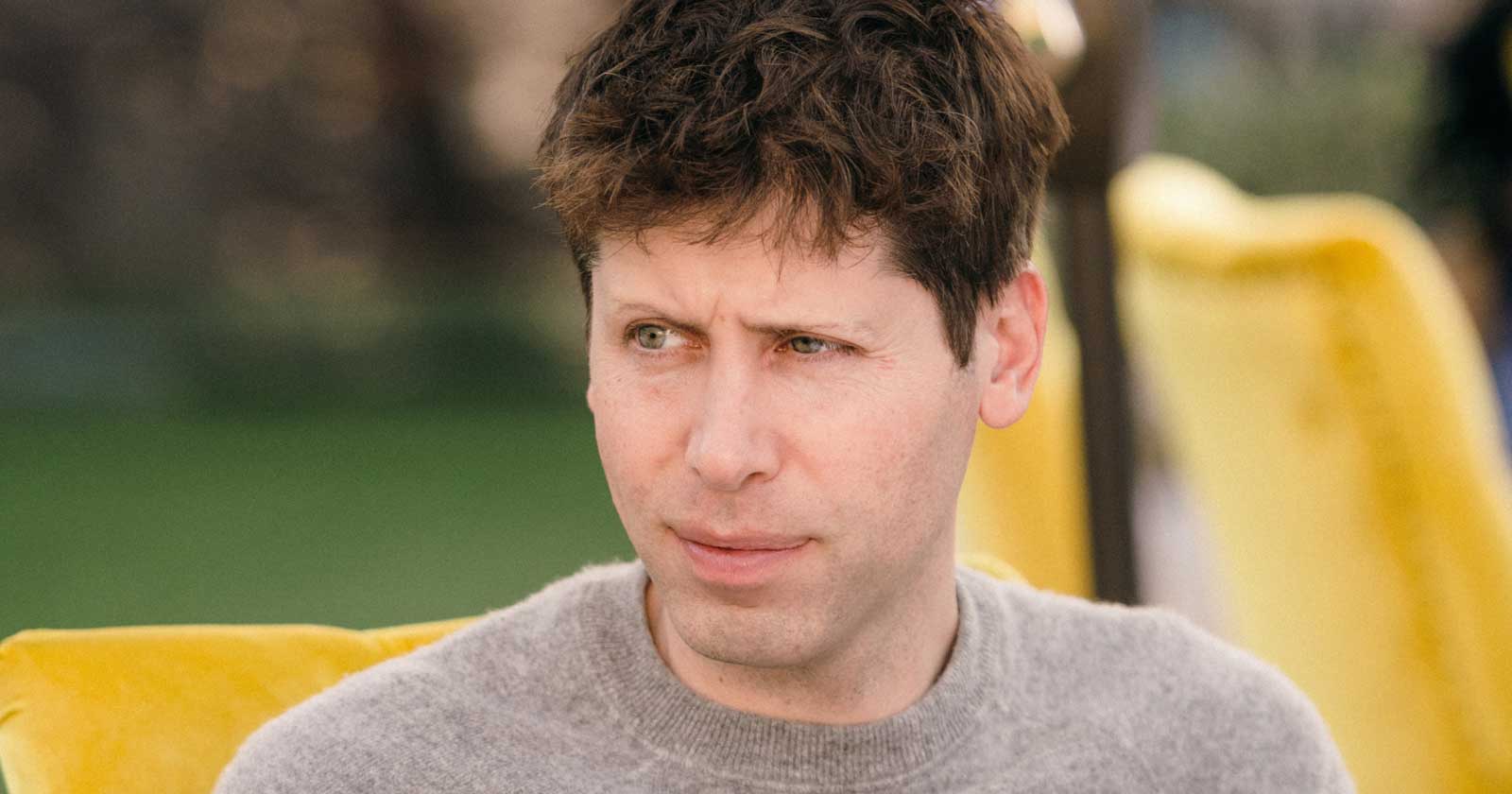
Sam Altman, CEO of OpenAI, dispelled rumors that a new search engine would be announced on Monday, May 13. Recent deals have raised the expectation that OpenAI will announce the integration of real-time content from English, Spanish, and French publications into ChatGPT, complete with links to the original sources.
OpenAI Search Is Not Happening
Many competing search engines have tried and failed to challenge Google as the leading search engine. A new wave of hybrid generative AI search engines is currently trying to knock Google from the top spot with arguably very little success.
Sam Altman is on record saying that creating a search engine to compete against Google is not a viable approach. He suggested that technological disruption was the way to replace Google by changing the search paradigm altogether. The speculation that Altman is going to announce a me-too search engine on Monday never made sense given his recent history of dismissing the concept as a non-starter.
So perhaps it’s not a surprise that he recently ended the speculation by explicitly saying that he will not be announcing a search engine on Monday.
He tweeted:
“not gpt-5, not a search engine, but we’ve been hard at work on some new stuff we think people will love! feels like magic to me.”
“New Stuff” May Be Iterative Improvement
It’s quite likely that what’s going to be announced is iterative which means it improves ChatGPT but not replaces it. This fits into how Altman recently expressed his approach with ChatGPT.
He remarked:
“And it does kind of suck to ship a product that you’re embarrassed about, but it’s much better than the alternative. And in this case in particular, where I think we really owe it to society to deploy iteratively.
There could totally be things in the future that would change where we think iterative deployment isn’t such a good strategy, but it does feel like the current best approach that we have and I think we’ve gained a lot from from doing this and… hopefully the larger world has gained something too.”
Improving ChatGPT iteratively is Sam Altman’s preference and recent clues point to what those changes may be.
Recent Deals Contain Clues
OpenAI has been making deals with news media and User Generated Content publishers since December 2023. Mainstream media has reported these deals as being about licensing content for training large language models. But they overlooked a a key detail that we reported on last month which is that these deals give OpenAI access to real-time information that they stated will be used to give attribution to that real-time data in the form of links.
That means that ChatGPT users will gain the ability to access real-time news and to use that information creatively within ChatGPT.
Dotdash Meredith Deal
Dotdash Meredith (DDM) is the publisher of big brand publications such as Better Homes & Gardens, FOOD & WINE, InStyle, Investopedia, and People magazine. The deal that was announced goes way beyond using the content as training data. The deal is explicitly about surfacing the Dotdash Meredith content itself in ChatGPT.
The announcement stated:
“As part of the agreement, OpenAI will display content and links attributed to DDM in relevant ChatGPT responses. …This deal is a testament to the great work OpenAI is doing on both fronts to partner with creators and publishers and ensure a healthy Internet for the future.
Over 200 million Americans each month trust our content to help them make decisions, solve problems, find inspiration, and live fuller lives. This partnership delivers the best, most relevant content right to the heart of ChatGPT.”
A statement from OpenAI gives credibility to the speculation that OpenAI intends to directly show licensed third-party content as part of ChatGPT answers.
OpenAI explained:
“We’re thrilled to partner with Dotdash Meredith to bring its trusted brands to ChatGPT and to explore new approaches in advancing the publishing and marketing industries.”
Something that DDM also gets out of this deal is that OpenAI will enhance DDM’s in-house ad targeting in order show more tightly focused contextual advertising.
Le Monde And Prisa Media Deals
In March 2024 OpenAI announced a deal with two global media companies, Le Monde and Prisa Media. Le Monde is a French news publication and Prisa Media is a Spanish language multimedia company. The interesting aspects of these two deals is that it gives OpenAI access to real-time data in French and Spanish.
Prisa Media is a global Spanish language media company based in Madrid, Spain that is comprised of magazines, newspapers, podcasts, radio stations, and television networks. It’s reach extends from Spain to America. American media companies include publications in the United States, Argentina, Bolivia, Chile, Colombia, Costa Rica, Ecuador, Mexico, and Panama. That is a massive amount of real-time information in addition to a massive audience of millions.
OpenAI explicitly announced that the purpose of this deal was to bring this content directly to ChatGPT users.
The announcement explained:
“We are continually making improvements to ChatGPT and are supporting the essential role of the news industry in delivering real-time, authoritative information to users. …Our partnerships will enable ChatGPT users to engage with Le Monde and Prisa Media’s high-quality content on recent events in ChatGPT, and their content will also contribute to the training of our models.”
That deal is not just about training data. It’s about bringing current events data to ChatGPT users.
The announcement elaborated in more detail:
“…our goal is to enable ChatGPT users around the world to connect with the news in new ways that are interactive and insightful.”
As noted in our April 30th article that revealed that OpenAI will show links in ChatGPT, OpenAI intends to show third party content with links to that content.
OpenAI commented on the purpose of the Le Monde and Prisa Media partnership:
“Over the coming months, ChatGPT users will be able to interact with relevant news content from these publishers through select summaries with attribution and enhanced links to the original articles, giving users the ability to access additional information or related articles from their news sites.”
There are additional deals with other groups like The Financial Times which also stress that this deal will result in a new ChatGPT feature that will allow users to interact with real-time news and current events .
OpenAI’s Monday May 13 Announcement
There are many clues that the announcement on Monday will be that ChatGPT users will gain the ability to interact with content about current events. This fits into the terms of recent deals with news media organizations. There may be other features announced as well but this part is something that there are many clues pointing to.
Watch Altman’s interview at Stanford University
Featured Image by Shutterstock/photosince
SEO
Google’s Strategies For Dealing With Content Decay
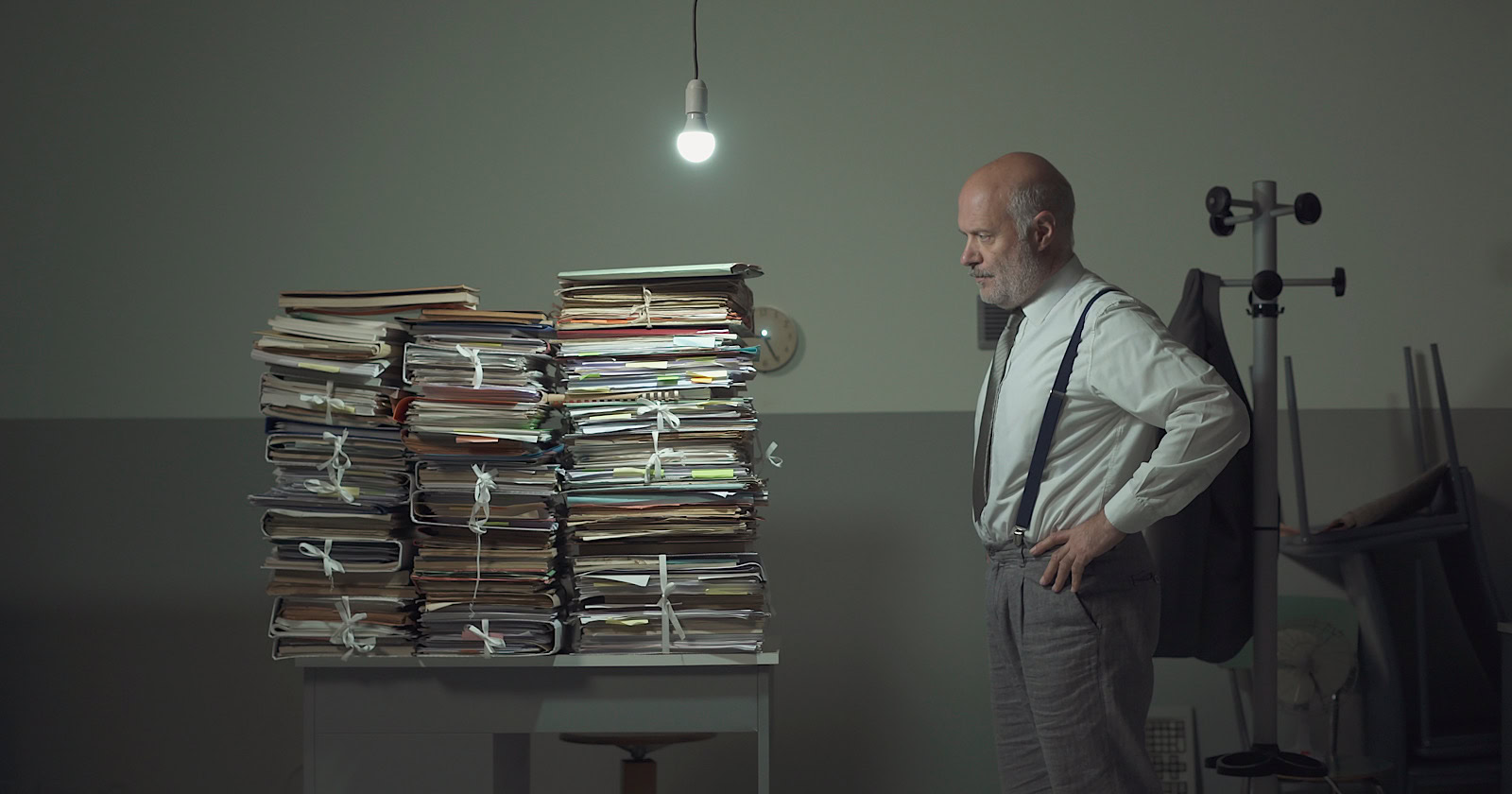
In the latest episode of the Search Off The Record podcast, Google Search Relations team members John Mueller and Lizzi Sassman did a deep dive into dealing with “content decay” on websites.
Outdated content is a natural issue all sites face over time, and Google has outlined strategies beyond just deleting old pages.
While removing stale content is sometimes necessary, Google recommends taking an intentional, format-specific approach to tackling content decay.
Archiving vs. Transitional Guides
Google advises against immediately removing content that becomes obsolete, like materials referencing discontinued products or services.
Removing content too soon could confuse readers and lead to a poor experience, Sassman explains:
“So, if I’m trying to find out like what happened, I almost need that first thing to know. Like, “What happened to you?” And, otherwise, it feels almost like an error. Like, “Did I click a wrong link or they redirect to the wrong thing?””
Sassman says you can avoid confusion by providing transitional “explainer” pages during deprecation periods.
A temporary transition guide informs readers of the outdated content while steering them toward updated resources.
Sassman continues:
“That could be like an intermediary step where maybe you don’t do that forever, but you do it during the transition period where, for like six months, you have them go funnel them to the explanation, and then after that, all right, call it a day. Like enough people know about it. Enough time has passed. We can just redirect right to the thing and people aren’t as confused anymore.”
When To Update Vs. When To Write New Content
For reference guides and content that provide authoritative overviews, Google suggests updating information to maintain accuracy and relevance.
However, for archival purposes, major updates may warrant creating a new piece instead of editing the original.
Sassman explains:
“I still want to retain the original piece of content as it was, in case we need to look back or refer to it, and to change it or rehabilitate it into a new thing would almost be worth republishing as a new blog post if we had that much additional things to say about it.”
Remove Potentially Harmful Content
Google recommends removing pages in cases where the outdated information is potentially harmful.
Sassman says she arrived at this conclusion when deciding what to do with a guide involving obsolete structured data:
“I think something that we deleted recently was the “How to Structure Data” documentation page, which I thought we should just get rid of it… it almost felt like that’s going to be more confusing to leave it up for a period of time.
And actually it would be negative if people are still adding markup, thinking they’re going to get something. So what we ended up doing was just delete the page and redirect to the changelog entry so that, if people clicked “How To Structure Data” still, if there was a link somewhere, they could still find out what happened to that feature.”
Internal Auditing Processes
To keep your content current, Google advises implementing a system for auditing aging content and flagging it for review.
Sassman says she sets automated alerts for pages that haven’t been checked in set periods:
“Oh, so we have a little robot to come and remind us, “Hey, you should come investigate this documentation page. It’s been x amount of time. Please come and look at it again to make sure that all of your links are still up to date, that it’s still fresh.””
Context Is Key
Google’s tips for dealing with content decay center around understanding the context of outdated materials.
You want to prevent visitors from stumbling across obsolete pages without clarity.
Additional Google-recommended tactics include:
- Prominent banners or notices clarifying a page’s dated nature
- Listing original publish dates
- Providing inline annotations explaining how older references or screenshots may be obsolete
How This Can Help You
Following Google’s recommendations for tackling content decay can benefit you in several ways:
- Improved user experience: By providing clear explanations, transition guides, and redirects, you can ensure that visitors don’t encounter confusing or broken pages.
- Maintained trust and credibility: Removing potentially harmful or inaccurate content and keeping your information up-to-date demonstrates your commitment to providing reliable and trustworthy resources.
- Better SEO: Regularly auditing and updating your pages can benefit your website’s search rankings and visibility.
- Archival purposes: By creating new content instead of editing older pieces, you can maintain a historical record of your website’s evolution.
- Streamlined content management: Implementing internal auditing processes makes it easier to identify and address outdated or problematic pages.
By proactively tackling content decay, you can keep your website a valuable resource, improve SEO, and maintain an organized content library.
Listen to the full episode of Google’s podcast below:
Featured Image: Stokkete/Shutterstock
SEO
25 Snapchat Statistics & Facts For 2024
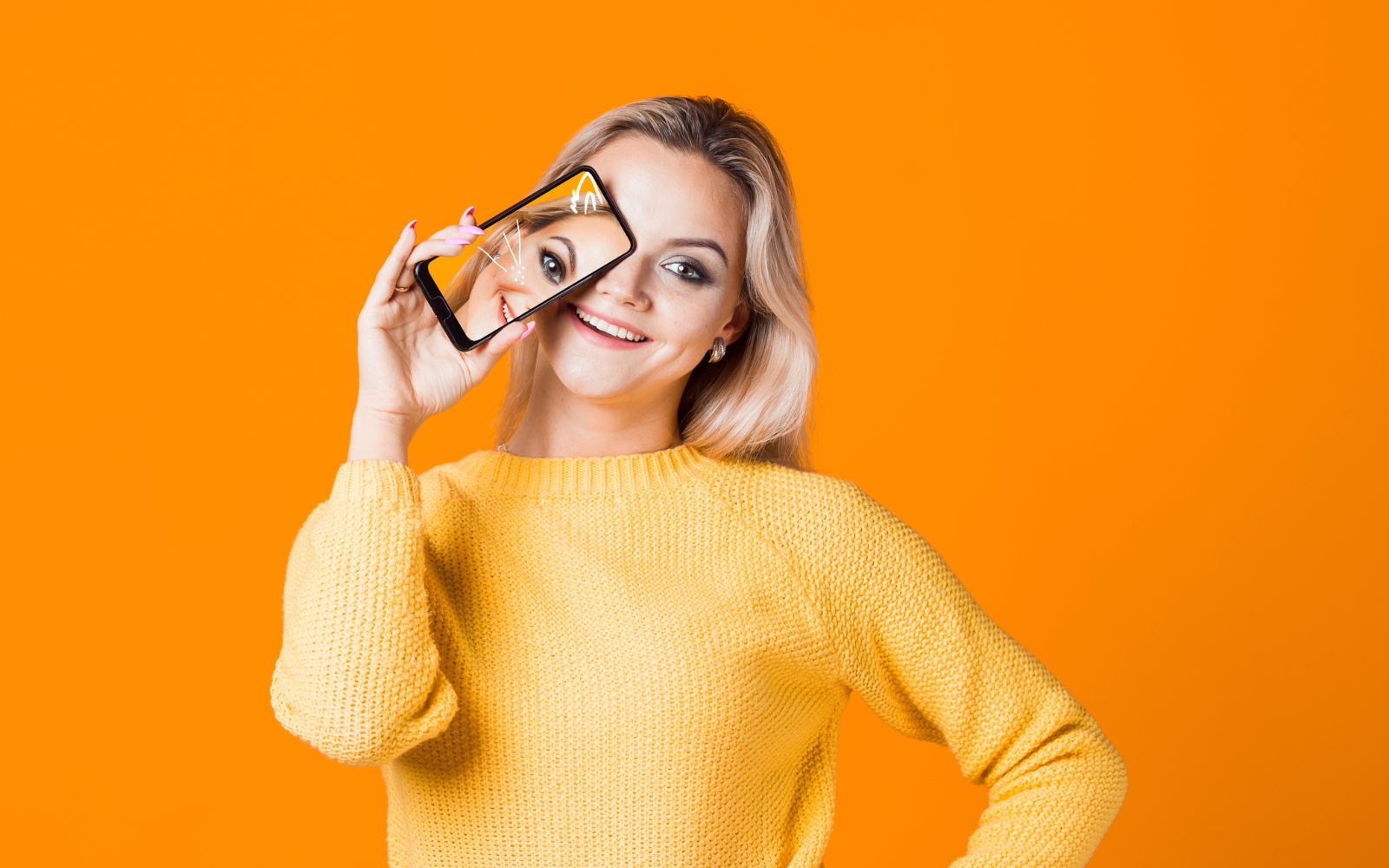
Snapchat, known for its ephemeral content, innovative augmented reality (AR) features, and fiercely loyal user base, is a vital player in the social media landscape.
While it sometimes flies under the radar – as other platforms like TikTok, YouTube, and Instagram tend to dominate the cultural conversation – Snapchat is an incredibly powerful marketing tool that holds a unique place in the hearts and minds of its users.
In this article, we’ll explore what you need to know about Snapchat, with insights that shed light on what audiences think of the app and where its strengths lie.
From user growth trends to advertising effectiveness, let’s look at the state of Snapchat right now.
What Is Snapchat?
Snapchat is a social media app that allows users to share photos and videos with friends and followers online.
Unlike other social platforms like Facebook, Instagram, and TikTok – where much of the content is stored permanently – Snapchat prioritizes ephemeral content only.
Once viewed, Snapchat content disappears, which adds a layer of spontaneity and privacy to digital interactions.
Snapchat leverages the power of augmented reality to entertain its audience by creating interactive and immersive experiences through features like AR lenses.
Users can also explore a variety of stickers, drawing tools, and emojis to add a personal touch to everything they post.
What started as a small collection of tools in 2011 has now expanded to a massive library of innovative features, such as a personalized 3D Snap Map, gesture recognition, audio recommendations for lenses, generative AI capabilities, and much more.
Creating an account on Snapchat is easy. Simply download the app on Google Play or the App Store. Install it on your device, and you’re ready!
-
Screenshot from Google Play, December 2023
25 Surprising Facts You Didn’t Know About Snapchat
Let’s dive in!
1. Snapchat Has 406 Million Daily Active Users
That number, released by the company in October 2023, represents an increase of 43 million year-over-year – a 12% increase.
Here’s a chart from Statista showing Snapchat’s user growth from 2014 to 2023:
-
Screenshot from Statista.com, December 2023
And with 750 million monthly active users (MAUs), Snapchat is the fifth-biggest social media network in the world.
2. Users 18-24 Years Old Account For The Biggest Chunk Of Snapchat’s Audience
According to Snapchat’s own advertising data, the platform has 243.5 million users aged 18 to 24 – representing 38.6% of its total ad audience.
The second largest group of users are between the ages of 25 and 34, followed by 13-17-year-olds – proving that Snapchat is reaching young people around the world.
On the flip side, the platform isn’t huge with older users; people aged 50 and over account for only 3.8% of Snapchat’s total ad audience.
As a marketer, you can take a hint on what your campaign should focus on if you use Snapchat. As Snapchat’s own report puts it:
“From its inception, Snapchat has inherently created a frictionless space where Gen Z creatives can experiment with their identities, yet not have to feel like they’re ‘on brand’ in communicating to their close friend groups.”
3. Snapchat Reaches 90% Of The 13 To 24-Year-Old Population
It also reaches 75% of people between the ages of 13 and 34 in over 25 countries, according to Snapchat’s estimates.
In the US, 59% of American teenagers (between the ages of 13 and 17) report using Snapchat. This number amounts to roughly six in 10 US teens.
4. Snapchat Users Open The App Nearly 40 Times A Day
According to the company, this means people interact with their social circles on Snapchat more than any other social network.
In the US, about half of teenagers (51%) report using Snapchat at least once a day – making it slightly more popular than Instagram, but not quite as popular as YouTube or TikTok.
5. Taco Bell Paid $75,000 For 24 Hours Of The Taco Filter/Ad
To boost sales, Taco Bell launched the taco filter on Snapchat. Here’s what it looked like.
Today only: turn yourself into a taco using our @Snapchat lens. Because Cinco de Mayo. pic.twitter.com/P4KwLdFNFZ
— Taco Bell (@tacobell) May 5, 2016
The filter is humorous, relevant, and unique. Users adored it, and it got 224 million views.
That’s great, considering Taco Bell paid $75,000 for the ad – which actually proved to be a great investment for the exposure the brand received.
6. More Than Half (50.6%) Of Snapchat Users Are Female
In contrast, 48.7% of the platform’s global users are male.
While there is not a huge discrepancy between the demographics here, it’s helpful information for any marketers looking to put together Snapchat campaigns.
7. Snapchat Is The No. 1 App People Use To Share What They Bought
Is your brand looking to reach young social media users around the world? Snapchat could be the perfect platform for you.
People are 45% more likely to recommend brands to friends on Snapchat compared to other platforms.
They’re also 2X more likely to post about a gift after receiving it – making Snapchat a powerful tool for influencer marketing and brand partnerships.
8. Snapchat Pioneered Vertical Video Ads
Once a novelty in the social media industry, vertical video ads have become one of the most popular ways to advertise on social media and reach global audiences.
What are vertical video ads? It’s self-explanatory: They’re ads that can be viewed with your phone held vertically. The ad format is optimized for how we use our mobile devices and designed to create a non-disruptive experience for users.
You’ve definitely seen countless video ads by now, but did you know Snapchat pioneered them?
9. You Can Follow Rock Star Business Experts On Snapchat
Who knew Snapchat could be a powerful business tool? Here are the top three experts you should follow right now:
10. More Than 250 Million Snapchatters Engage With AR Every Day, On Average
Snapchat was the first social media app to really prioritize the development of AR features, and it’s paid off.
Over 70% of users engage with AR on the first day that they download the app – and, to date, there have been more than 3 million lenses launched on Snapchat.
11. People Are 34% More Likely To Purchase Products They See Advertised On Snapchat
When compared to watching the same ad on other social media platform, Snapchat proves to be an effective way to reach and convert.
12. Snapchat Is The King Of Ephemeral Content Marketing
Ephemeral content marketing uses video, photos, and media that are only accessible for a limited time.
Here are three reasons it works:
- It creates a sense of urgency.
- It appeals to buyers who don’t want to feel “sold.”
- It’s more personalized than traditional sales funnel marketing.
Guess who’s one of the kings of ephemeral content marketing? That’s right: Snapchat.
Consider that if it weren’t for Snapchat, Instagram Stories would likely not exist right now.
13. More Than 5 Million People Subscribe To Snapchat+
Snapchat+ is the platform’s paid subscription service that gives users access to exclusive and pre-release features on the platform.
Subscribers also receive a range of other perks, including options to customize their app experience and the ability to see how many times their content has been rewatched.
The fact that so many millions of users are willing to pay for special access and features to Snapchat should be a sign to brands and marketers everywhere that the platform has a strong pull with its audience.
Beyond that, the fact that Snapchat+ drew 5 million subscribers within just a year or so of launching is impressive on its own.
14. Snapchat Reaches Nearly Half Of US Smartphone Users
According to Statista, approximately 309 million American adults use smartphones today.
Snapchat’s ability to reach such a considerable portion of US smartphone users is notable.
15. Snapchat Users Spend An Average Of 19 Minutes Per Day On The App
That’s 19 minutes brands can use to connect with people, grow brand awareness, and convey their message.
16. Snapchat’s Original Name Was Picaboo
In fact, Snapchat did run as Picaboo for about a year.
17. Snapchat Was Created After 34 Failures
Snapchat creators Evan Spiegel, Bobby Murphy, and Frank Reginald Brown worked on the Snapchat project while they were studying at Stanford University.
After 34 failures, they finally developed the app as we know it today.
18. Snapchat’s Creators Had A Major Falling-Out Before The App Was Released
Frank Reginald Brown was ousted from the Snapchat project by his friends.
Although no one knows the real story, Brown claims Spiegel and Murphy changed the server passwords and ceased communication with him a month before Snapchat was launched.
19. Snapchat Downloads Doubled After The Launch Of The Toddler & Gender Swap Filters
Users downloaded Snapchat 41.5 million times in a month after the release of these filters!
20. Mark Zuckerberg Tried To Buy Snapchat
Snapchat’s owners refused to sell Snapchat to Zuckerberg (even though the offer went as high as $3 billion!).
21. Snapchat’s Mascot Is Called Ghostface Chillah
The mascot was inspired by Ghostface Killah of the Wu-Tang Clan – and when you consider that the app was once called “Picaboo,” the ghost logo makes more sense.
Apparently, Snapchat co-founder and CEO Evan Spiegel has said that he developed the mascot himself and chose a ghost based on the ephemeral nature of Snapchat content.
22. Facebook And Instagram Borrowed Ephemeral Content From Snapchat
As we mentioned above, we have Snapchat to thank for Facebook and Instagram Stories, which have since become integral to the social media experience.
Snapchat also pioneered the use of AR filters, which were adopted by Instagram and paved the way for the filters that dominate the world of TikTok today.
23. 75% Of Gen Z And Millennials Say Snapchat Is The No. 1 Platform For Sharing Real-Life Experiences
Social media is all about authentic moments and human connection – and social media marketing is no different.
With such a large number of young people preferring Snapchat over other platforms for sharing their life experiences, marketers should follow suit.
Find ways to share behind-the-scenes moments with your team and company, and emphasize the humans behind the brand.
24. Snapchat Users Have Over $4.4 Trillion In Global Spending Power
That’s nothing to sneeze at.
25. In 2022, Snapchat Generated $4.6 Billion In Revenue
It is currently valued at over $20 billion.
Looking Ahead With Snapchat
Snapchat’s ephemeral content, intimacy, and spontaneity are strong points for everyday users, content creators, and businesses alike.
Marketers should keep a keen eye on emerging trends within the platform, such as new AR advancements and evolving user demographics.
Those looking to reach younger audiences or show an authentic, human side of their brand should consider wading into the waters of Snapchat.
By harnessing the power of ephemeral content and engaging features, brands can effectively use Snapchat to grow their brand awareness, engage with audiences on a more personal level, and stay relevant in the fast-paced world of digital marketing.
More resources:
Featured Image: Trismegist san/Shutterstock
-
MARKETING7 days ago
The key to correcting the C-suite trust deficit
-
MARKETING6 days ago
A Recap of Everything Marketers & Advertisers Need to Know
-
MARKETING4 days ago
How To Protect Your People and Brand
-
PPC4 days ago
How the TikTok Algorithm Works in 2024 (+9 Ways to Go Viral)
-
SEARCHENGINES5 days ago
Google Started Enforcing The Site Reputation Abuse Policy
-
SEO7 days ago
128 Top SEO Tools That Are 100% Free
-
SEO5 days ago
Blog Post Checklist: Check All Prior to Hitting “Publish”
-
SEO3 days ago
How to Use Keywords for SEO: The Complete Beginner’s Guide
You must be logged in to post a comment Login