TECHNOLOGY
How Computer Vision-Powered Pose Estimation Can Optimize Smart City Mobility
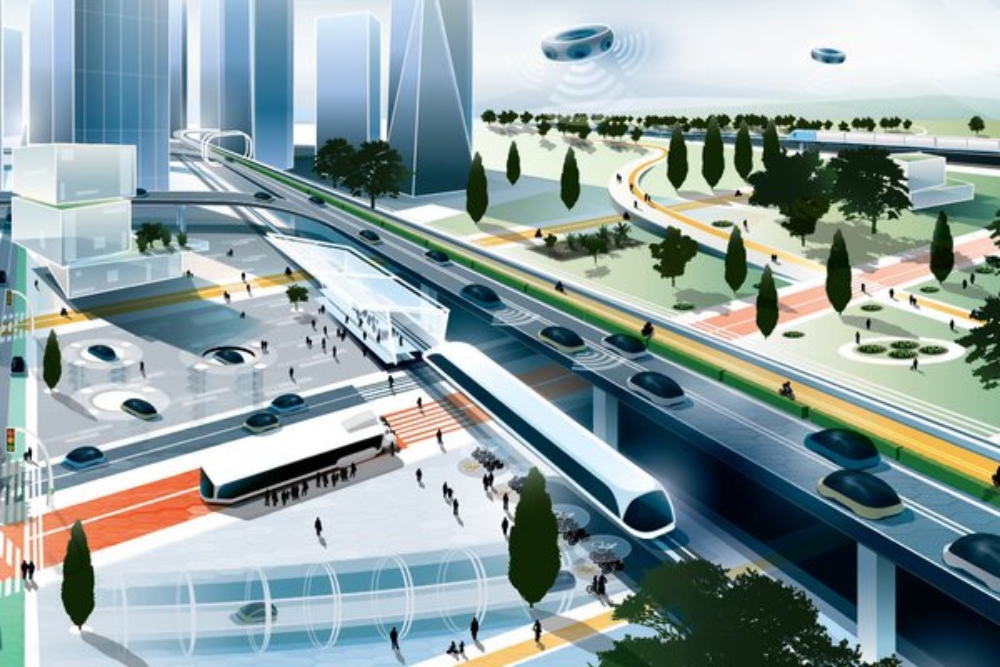
Pose estimation is an application of computer vision in smart cities.
It can be utilized for mobility management-related functions such as traffic control, elderly care in old age homes and several others.
Smart cities—or any cities, or towns, for that matter—are unrelenting cauldrons full of people and continual movement. Whether you talk about vehicular or pedestrian movement, one thing that everybody will unanimously agree on is the importance of mobility management. Poor traffic and vehicular management are directly responsible for approximately 1.3 million deaths every year. Smart cities, with all the technological and connectivity-based tools at their disposal, can use computer vision to make different kinds of mobility within them as frictionless as possible. Pose estimation, a computer vision-driven application, monitors the movement of people, vehicles, and other moving entities in smart cities. Based on this monitoring data, smart city administrators can optimize the mobility of different kinds in an urban environment.
In this way, the use of computer vision in smart cities to aid urban mobility improves the livability quotient of such zones.
Accident Prevention with Vehicle Pose Estimation
To state the obvious, behavioral monitoring—such an integral component of pose estimation—is not applicable to vehicles. In fact, vehicles may appear to move in different ways based on different CCTV camera angles alone. Computer vision algorithms can be trained to predict where a vehicle is headed or its future speed based on factors such as its alignment, the various road signs, status of its parking lamps or side-indicators—whether they’re engaged or not—and similar other factors to estimate its position in the immediate future. If the cameras preempt the possibility of a collision between moving vehicles or a vehicle and a pedestrian, they can communicate this with the vehicles over a connected smart city network, which can then prevent the mishap from happening. This feature is particularly useful in blind alley situations.
In this way, involving computer vision in smart cities simplifies the task of traffic management, accident prevention, and vehicular mobility regulation.
Eldercare with Ambient Intelligence
Care homes are full of individuals with dementia and other old age-related mental conditions that may make them behave in unpredictable ways. Ambient intelligence involves sensors and computer vision cameras embedded into everyday objects for data collection. Without violating their privacy, such tools can be used to autonomously monitor them. Computer vision and machine learning algorithms can be modeled to detect potentially self-harming behavior and inform the concerned caretaker immediately.
Pose estimation serves to make the mobility of any kind smooth in a smart city. Machine learning and computer vision in smart cities simply make that necessity a possibility.
Source link
TECHNOLOGY
Next-gen chips, Amazon Q, and speedy S3
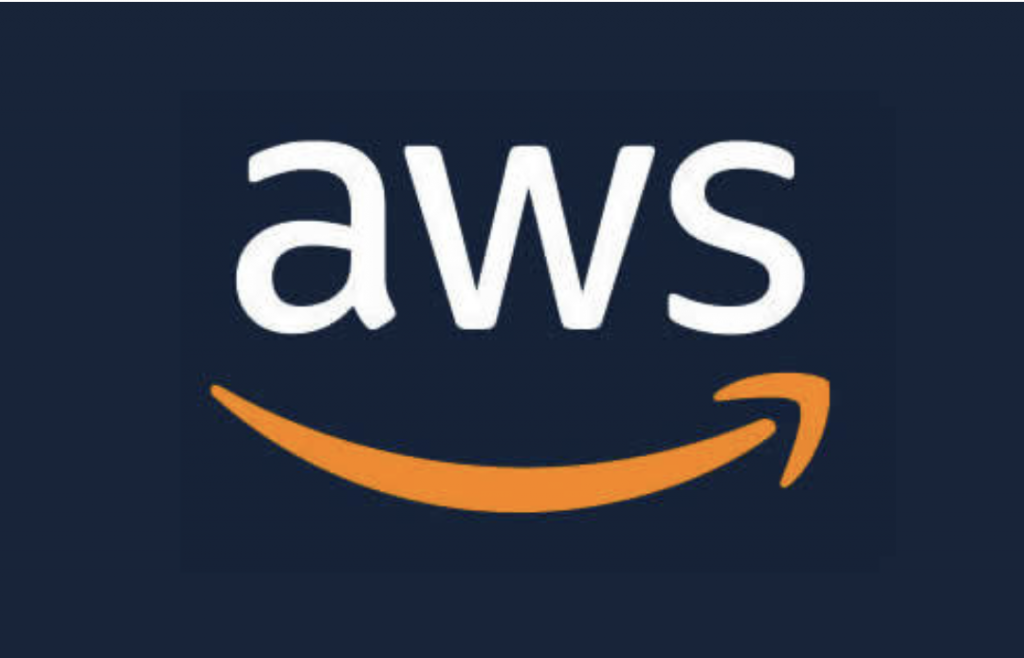
AWS re:Invent, which has been taking place from November 27 and runs to December 1, has had its usual plethora of announcements: a total of 21 at time of print.
Perhaps not surprisingly, given the huge potential impact of generative AI – ChatGPT officially turns one year old today – a lot of focus has been on the AI side for AWS’ announcements, including a major partnership inked with NVIDIA across infrastructure, software, and services.
Yet there has been plenty more announced at the Las Vegas jamboree besides. Here, CloudTech rounds up the best of the rest:
Next-generation chips
This was the other major AI-focused announcement at re:Invent: the launch of two new chips, AWS Graviton4 and AWS Trainium2, for training and running AI and machine learning (ML) models, among other customer workloads. Graviton4 shapes up against its predecessor with 30% better compute performance, 50% more cores and 75% more memory bandwidth, while Trainium2 delivers up to four times faster training than before and will be able to be deployed in EC2 UltraClusters of up to 100,000 chips.
The EC2 UltraClusters are designed to ‘deliver the highest performance, most energy efficient AI model training infrastructure in the cloud’, as AWS puts it. With it, customers will be able to train large language models in ‘a fraction of the time’, as well as double energy efficiency.
As ever, AWS offers customers who are already utilising these tools. Databricks, Epic and SAP are among the companies cited as using the new AWS-designed chips.
Zero-ETL integrations
AWS announced new Amazon Aurora PostgreSQL, Amazon DynamoDB, and Amazon Relational Database Services (Amazon RDS) for MySQL integrations with Amazon Redshift, AWS’ cloud data warehouse. The zero-ETL integrations – eliminating the need to build ETL (extract, transform, load) data pipelines – make it easier to connect and analyse transactional data across various relational and non-relational databases in Amazon Redshift.
A simple example of how zero-ETL functions can be seen is in a hypothetical company which stores transactional data – time of transaction, items bought, where the transaction occurred – in a relational database, but use another analytics tool to analyse data in a non-relational database. To connect it all up, companies would previously have to construct ETL data pipelines which are a time and money sink.
The latest integrations “build on AWS’s zero-ETL foundation… so customers can quickly and easily connect all of their data, no matter where it lives,” the company said.
Amazon S3 Express One Zone
AWS announced the general availability of Amazon S3 Express One Zone, a new storage class purpose-built for customers’ most frequently-accessed data. Data access speed is up to 10 times faster and request costs up to 50% lower than standard S3. Companies can also opt to collocate their Amazon S3 Express One Zone data in the same availability zone as their compute resources.
Companies and partners who are using Amazon S3 Express One Zone include ChaosSearch, Cloudera, and Pinterest.
Amazon Q
A new product, and an interesting pivot, again with generative AI at its core. Amazon Q was announced as a ‘new type of generative AI-powered assistant’ which can be tailored to a customer’s business. “Customers can get fast, relevant answers to pressing questions, generate content, and take actions – all informed by a customer’s information repositories, code, and enterprise systems,” AWS added. The service also can assist companies building on AWS, as well as companies using AWS applications for business intelligence, contact centres, and supply chain management.
Customers cited as early adopters include Accenture, BMW and Wunderkind.
Want to learn more about cybersecurity and the cloud from industry leaders? Check out Cyber Security & Cloud Expo taking place in Amsterdam, California, and London. Explore other upcoming enterprise technology events and webinars powered by TechForge here.
TECHNOLOGY
HCLTech and Cisco create collaborative hybrid workplaces
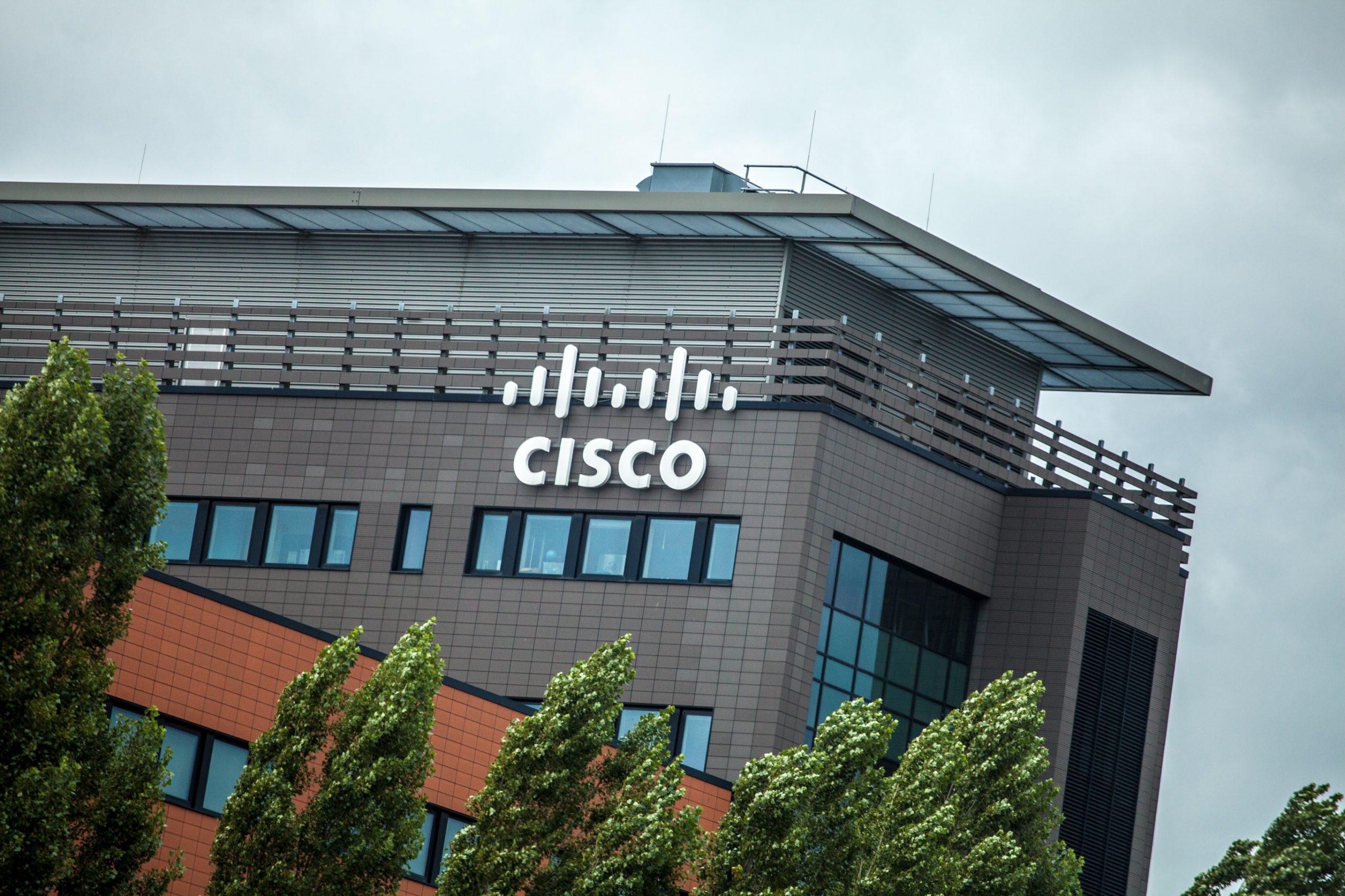
Digital comms specialist Cisco and global tech firm HCLTech have teamed up to launch Meeting-Rooms-as-a-Service (MRaaS).
Available on a subscription model, this solution modernises legacy meeting rooms and enables users to join meetings from any meeting solution provider using Webex devices.
The MRaaS solution helps enterprises simplify the design, implementation and maintenance of integrated meeting rooms, enabling seamless collaboration for their globally distributed hybrid workforces.
Rakshit Ghura, senior VP and Global head of digital workplace services, HCLTech, said: “MRaaS combines our consulting and managed services expertise with Cisco’s proficiency in Webex devices to change the way employees conceptualise, organise and interact in a collaborative environment for a modern hybrid work model.
“The common vision of our partnership is to elevate the collaboration experience at work and drive productivity through modern meeting rooms.”
Alexandra Zagury, VP of partner managed and as-a-Service Sales at Cisco, said: “Our partnership with HCLTech helps our clients transform their offices through cost-effective managed services that support the ongoing evolution of workspaces.
“As we reimagine the modern office, we are making it easier to support collaboration and productivity among workers, whether they are in the office or elsewhere.”
Cisco’s Webex collaboration devices harness the power of artificial intelligence to offer intuitive, seamless collaboration experiences, enabling meeting rooms with smart features such as meeting zones, intelligent people framing, optimised attendee audio and background noise removal, among others.
Want to learn more about cybersecurity and the cloud from industry leaders? Check out Cyber Security & Cloud Expo taking place in Amsterdam, California, and London. Explore other upcoming enterprise technology events and webinars powered by TechForge here.
TECHNOLOGY
Canonical releases low-touch private cloud MicroCloud
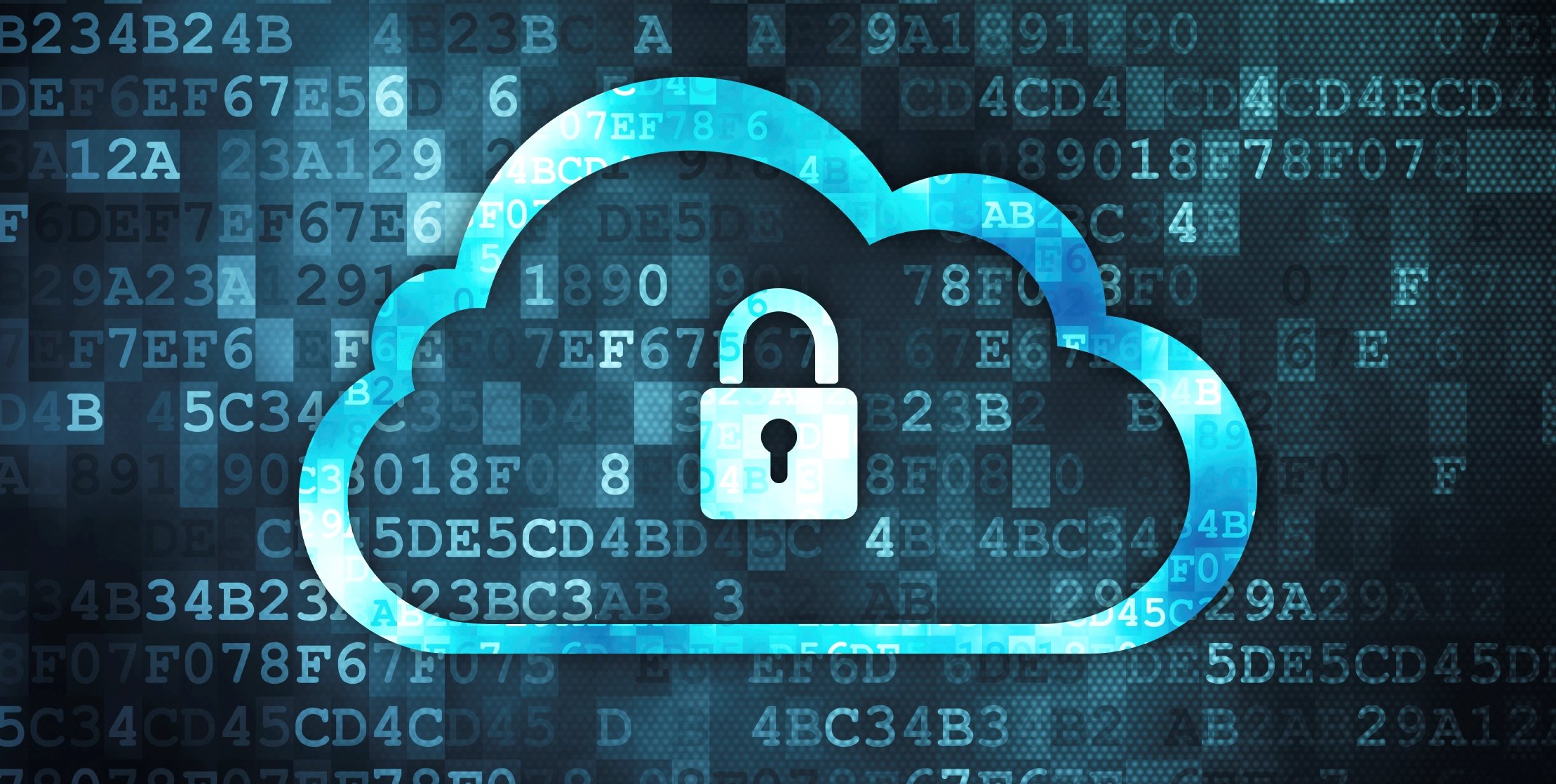
Canonical has announced the general availability of MicroCloud, a low-touch, open source cloud solution. MicroCloud is part of Canonical’s growing cloud infrastructure portfolio.
It is purpose-built for scalable clusters and edge deployments for all types of enterprises. It is designed with simplicity, security and automation in mind, minimising the time and effort to both deploy and maintain it. Conveniently, enterprise support for MicroCloud is offered as part of Canonical’s Ubuntu Pro subscription, with several support tiers available, and priced per node.
MicroClouds are optimised for repeatable and reliable remote deployments. A single command initiates the orchestration and clustering of various components with minimal involvement by the user, resulting in a fully functional cloud within minutes. This simplified deployment process significantly reduces the barrier to entry, putting a production-grade cloud at everyone’s fingertips.
Juan Manuel Ventura, head of architectures & technologies at Spindox, said: “Cloud computing is not only about technology, it’s the beating heart of any modern industrial transformation, driving agility and innovation. Our mission is to provide our customers with the most effective ways to innovate and bring value; having a complexity-free cloud infrastructure is one important piece of that puzzle. With MicroCloud, the focus shifts away from struggling with cloud operations to solving real business challenges” says
In addition to seamless deployment, MicroCloud prioritises security and ease of maintenance. All MicroCloud components are built with strict confinement for increased security, with over-the-air transactional updates that preserve data and roll back on errors automatically. Upgrades to newer versions are handled automatically and without downtime, with the mechanisms to hold or schedule them as needed.
With this approach, MicroCloud caters to both on-premise clouds but also edge deployments at remote locations, allowing organisations to use the same infrastructure primitives and services wherever they are needed. It is suitable for business-in-branch office locations or industrial use inside a factory, as well as distributed locations where the focus is on replicability and unattended operations.
Cedric Gegout, VP of product at Canonical, said: “As data becomes more distributed, the infrastructure has to follow. Cloud computing is now distributed, spanning across data centres, far and near edge computing appliances. MicroCloud is our answer to that.
“By packaging known infrastructure primitives in a portable and unattended way, we are delivering a simpler, more prescriptive cloud experience that makes zero-ops a reality for many Industries.“
MicroCloud’s lightweight architecture makes it usable on both commodity and high-end hardware, with several ways to further reduce its footprint depending on your workload needs. In addition to the standard Ubuntu Server or Desktop, MicroClouds can be run on Ubuntu Core – a lightweight OS optimised for the edge. With Ubuntu Core, MicroClouds are a perfect solution for far-edge locations with limited computing capabilities. Users can choose to run their workloads using Kubernetes or via system containers. System containers based on LXD behave similarly to traditional VMs but consume fewer resources while providing bare-metal performance.
Coupled with Canonical’s Ubuntu Pro + Support subscription, MicroCloud users can benefit from an enterprise-grade open source cloud solution that is fully supported and with better economics. An Ubuntu Pro subscription offers security maintenance for the broadest collection of open-source software available from a single vendor today. It covers over 30k packages with a consistent security maintenance commitment, and additional features such as kernel livepatch, systems management at scale, certified compliance and hardening profiles enabling easy adoption for enterprises. With per-node pricing and no hidden fees, customers can rest assured that their environment is secure and supported without the expensive price tag typically associated with cloud solutions.
Want to learn more about cybersecurity and the cloud from industry leaders? Check out Cyber Security & Cloud Expo taking place in Amsterdam, California, and London. Explore other upcoming enterprise technology events and webinars powered by TechForge here.
-
PPC7 days ago
19 Best SEO Tools in 2024 (For Every Use Case)
-
SEARCHENGINES6 days ago
Daily Search Forum Recap: April 19, 2024
-
WORDPRESS7 days ago
How to Make $5000 of Passive Income Every Month in WordPress
-
WORDPRESS5 days ago
13 Best HubSpot Alternatives for 2024 (Free + Paid)
-
SEO7 days ago
25 WordPress Alternatives Best For SEO
-
WORDPRESS6 days ago
7 Best WooCommerce Points and Rewards Plugins (Free & Paid)
-
MARKETING6 days ago
Battling for Attention in the 2024 Election Year Media Frenzy
-
AFFILIATE MARKETING7 days ago
AI Will Transform the Workplace. Here’s How HR Can Prepare for It.
You must be logged in to post a comment Login