SEO
Visualizing Hot Topics Using Python To Analyze News Sitemaps
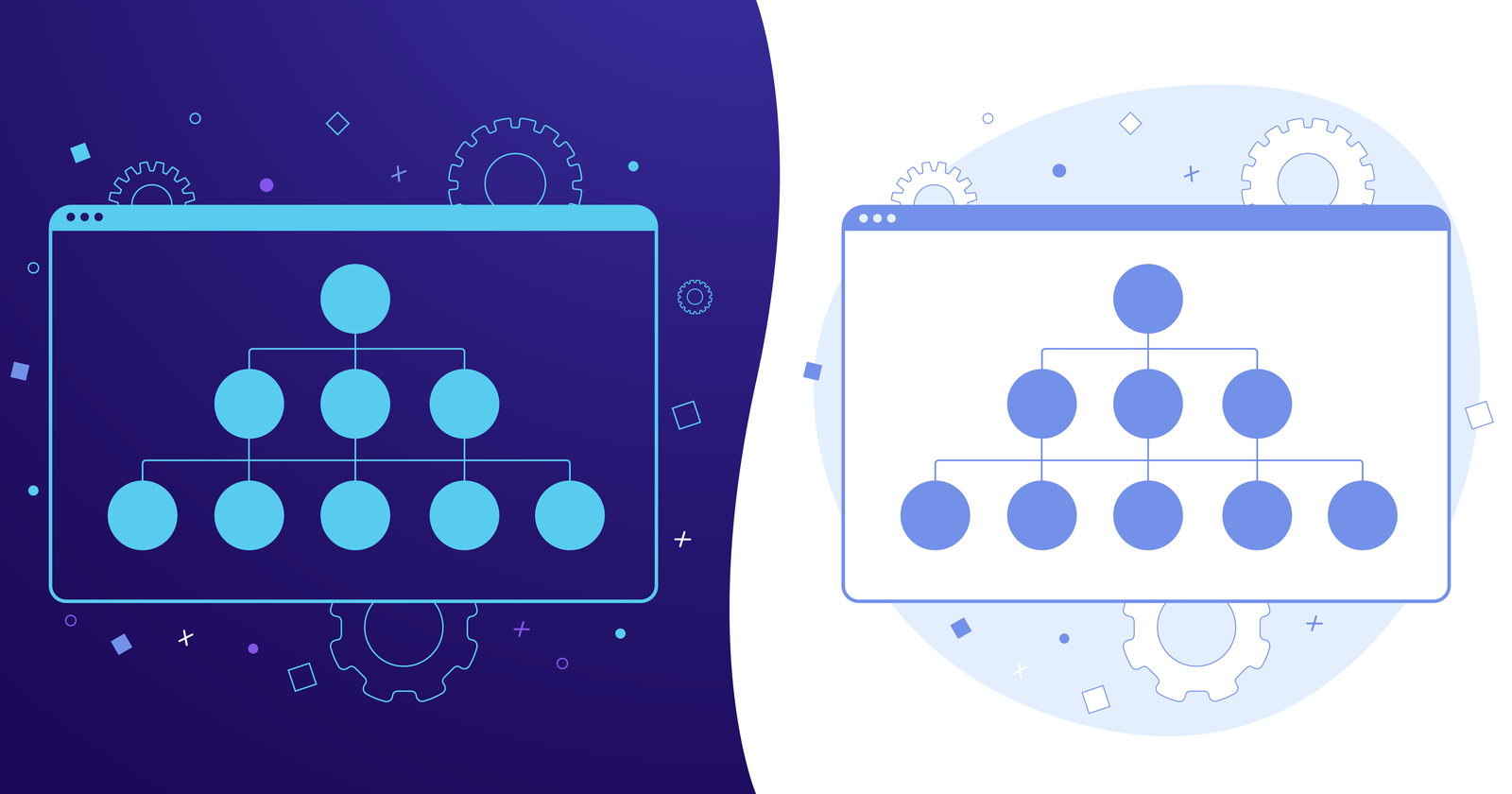
News sitemaps use different and unique sitemap protocols to provide more information for the news search engines.
A news sitemap contains the news published in the last 48 hours.
News sitemap tags include the news publication’s title, language, name, genre, publication date, keywords, and even stock tickers.
How can you use these sitemaps to your advantage for content research and competitive analysis?
In this Python tutorial, you’ll learn a 10-step process for analyzing news sitemaps and visualizing topical trends discovered therein.
Housekeeping Notes To Get Us Started
This tutorial was written during Russia’s invasion of Ukraine.
Using machine learning, we can even label news sources and articles according to which news source is “objective” and which news source is “sarcastic.”
But to keep things simple, we will focus on topics with frequency analysis.
We will use more than 10 global news sources across the U.S. and U.K.
Note: We would like to include Russian news sources, but they do not have a proper news sitemap. Even if they had, they block the external requests.
Comparing the word occurrence of “invasion” and “liberation” from Western and Eastern news sources shows the benefit of distributional frequency text analysis methods.
What You Need To Analyze News Content With Python
The related Python libraries for auditing a news sitemap to understand the news source’s content strategy are listed below:
- Advertools.
- Pandas.
- Plotly Express, Subplots, and Graph Objects.
- Re (Regex).
- String.
- NLTK (Corpus, Stopwords, Ngrams).
- Unicodedata.
- Matplotlib.
- Basic Python Syntax Understanding.
10 Steps For News Sitemap Analysis With Python
All set up? Let’s get to it.
1. Take The News URLs From News Sitemap
We chose the “The Guardian,” “New York Times,” “Washington Post,” “Daily Mail,” “Sky News,” “BBC,” and “CNN” to examine the News URLs from the News Sitemaps.
df_guardian = adv.sitemap_to_df("http://www.theguardian.com/sitemaps/news.xml") df_nyt = adv.sitemap_to_df("https://www.nytimes.com/sitemaps/new/news.xml.gz") df_wp = adv.sitemap_to_df("https://www.washingtonpost.com/arcio/news-sitemap/") df_bbc = adv.sitemap_to_df("https://www.bbc.com/sitemaps/https-index-com-news.xml") df_dailymail = adv.sitemap_to_df("https://www.dailymail.co.uk/google-news-sitemap.xml") df_skynews = adv.sitemap_to_df("https://news.sky.com/sitemap-index.xml") df_cnn = adv.sitemap_to_df("https://edition.cnn.com/sitemaps/cnn/news.xml")
2. Examine An Example News Sitemap With Python
I have used BBC as an example to demonstrate what we just extracted from these news sitemaps.
df_bbc
The BBC Sitemap has the columns below.
df_bbc.columns

The general data structures of these columns are below.
df_bbc.info()

The BBC doesn’t use the “news_publication” column and others.
3. Find The Most Used Words In URLs From News Publications
To see the most used words in the news sites’ URLs, we need to use “str,” “explode”, and “split” methods.
df_dailymail["loc"].str.split("/").str[5].str.split("-").explode().value_counts().to_frame()
loc |
|
---|---|
article |
176 |
Russian |
50 |
Ukraine |
50 |
says |
38 |
reveals |
38 |
... |
... |
readers |
1 |
Red |
1 |
Cross |
1 |
provide |
1 |
weekend.html |
1 |
5445 rows × 1 column
We see that for the “Daily Mail,” “Russia and Ukraine” are the main topic.
4. Find The Most Used Language In News Publications
The URL structure or the “language” section of the news publication can be used to see the most used languages in news publications.
In this sample, we used “BBC” to see their language prioritization.
df_bbc["publication_language"].head(20).value_counts().to_frame()
publication_language | |
en |
698 |
fa |
52 |
sr |
52 |
ar |
47 |
mr |
43 |
hi |
43 |
gu |
41 |
ur |
35 |
pt |
33 |
te |
31 |
ta |
31 |
cy |
30 |
ha |
29 |
tr |
28 |
es |
25 |
sw |
22 |
cpe |
22 |
ne |
21 |
pa |
21 |
yo |
20 |
20 rows × 1 column
To reach out to the Russian population via Google News, every western news source should use the Russian language.
Some international news institutions started to perform this perspective.
If you are a news SEO, it’s helpful to watch Russian language publications from competitors to distribute the objective news to Russia and compete within the news industry.
5. Audit The News Titles For Frequency Of Words
We used BBC to see the “news titles” and which words are more frequent.
df_bbc["news_title"].str.split(" ").explode().value_counts().to_frame()
news_title |
|
---|---|
to |
232 |
in |
181 |
- |
141 |
of |
140 |
for |
138 |
... |
... |
ፊልም |
1 |
ብላክ |
1 |
ባንኪ |
1 |
ጕሒላ |
1 |
niile |
1 |
11916 rows × 1 columns
The problem here is that we have “every type of word in the news titles,” such as “contextless stop words.”
We need to clean these types of non-categorical terms to understand their focus better.
from nltk.corpus import stopwords stop = stopwords.words('english') df_bbc_news_title_most_used_words = df_bbc["news_title"].str.split(" ").explode().value_counts().to_frame() pat = r'b(?:{})b'.format('|'.join(stop)) df_bbc_news_title_most_used_words.reset_index(drop=True, inplace=True) df_bbc_news_title_most_used_words["without_stop_words"] = df_bbc_news_title_most_used_words["words"].str.replace(pat,"") df_bbc_news_title_most_used_words.drop(df_bbc_news_title_most_used_words.loc[df_bbc_news_title_most_used_words["without_stop_words"]==""].index, inplace=True) df_bbc_news_title_most_used_words

We have removed most of the stop words with the help of the “regex” and “replace” method of Pandas.
The second concern is removing the “punctuations.”
For that, we will use the “string” module of Python.
import string df_bbc_news_title_most_used_words["without_stop_word_and_punctation"] = df_bbc_news_title_most_used_words['without_stop_words'].str.replace('[{}]'.format(string.punctuation), '') df_bbc_news_title_most_used_words.drop(df_bbc_news_title_most_used_words.loc[df_bbc_news_title_most_used_words["without_stop_word_and_punctation"]==""].index, inplace=True) df_bbc_news_title_most_used_words.drop(["without_stop_words", "words"], axis=1, inplace=True) df_bbc_news_title_most_used_words
news_title |
without_stop_word_and_punctation |
|
---|---|---|
Ukraine |
110 |
Ukraine |
v |
83 |
v |
de |
61 |
de |
Ukraine: |
60 |
Ukraine |
da |
51 |
da |
... |
... |
... |
ፊልም |
1 |
ፊልም |
ብላክ |
1 |
ብላክ |
ባንኪ |
1 |
ባንኪ |
ጕሒላ |
1 |
ጕሒላ |
niile |
1 |
niile |
11767 rows × 2 columns
Or, use “df_bbc_news_title_most_used_words[“news_title”].to_frame()” to take a more clear picture of data.
news_title |
|
---|---|
Ukraine |
110 |
v |
83 |
de |
61 |
Ukraine: |
60 |
da |
51 |
... |
... |
ፊልም |
1 |
ብላክ |
1 |
ባንኪ |
1 |
ጕሒላ |
1 |
niile |
1 |
11767 rows × 1 columns
We see 11,767 unique words in the URLs of the BBC, and Ukraine is the most popular, with 110 occurrences.
There are different Ukraine-related phrases from the data frame, such as “Ukraine:.”
The “NLTK Tokenize” can be used to unite these types of different variations.
The next section will use a different method to unite them.
Note: If you want to make things easier, use Advertools as below.
adv.word_frequency(df_bbc["news_title"],phrase_len=2, rm_words=adv.stopwords.keys())
The result is below.

“adv.word_frequency” has the attributes “phrase_len” and “rm_words” to determine the length of the phrase occurrence and remove the stop words.
You may tell me, why didn’t I use it in the first place?
I wanted to show you an educational example with “regex, NLTK, and the string” so that you can understand what’s happening behind the scenes.
6. Visualize The Most Used Words In News Titles
To visualize the most used words in the news titles, you can use the code block below.
df_bbc_news_title_most_used_words["news_title"] = df_bbc_news_title_most_used_words["news_title"].astype(int) df_bbc_news_title_most_used_words["without_stop_word_and_punctation"] = df_bbc_news_title_most_used_words["without_stop_word_and_punctation"].astype(str) df_bbc_news_title_most_used_words.index = df_bbc_news_title_most_used_words["without_stop_word_and_punctation"] df_bbc_news_title_most_used_words["news_title"].head(20).plot(title="The Most Used Words in BBC News Titles")

You realize that there is a “broken line.”
Do you remember the “Ukraine” and “Ukraine:” in the data frame?
When we remove the “punctuation,” the second and first values become the same.
That’s why the line graph says that Ukraine appeared 60 times and 110 times separately.
To prevent such a data discrepancy, use the code block below.
df_bbc_news_title_most_used_words_1 = df_bbc_news_title_most_used_words.drop_duplicates().groupby('without_stop_word_and_punctation', sort=False, as_index=True).sum() df_bbc_news_title_most_used_words_1
news_title |
|
---|---|
without_stop_word_and_punctation |
|
Ukraine |
175 |
v |
83 |
de |
61 |
da |
51 |
и |
41 |
... |
... |
ፊልም |
1 |
ብላክ |
1 |
ባንኪ |
1 |
ጕሒላ |
1 |
niile |
1 |
11109 rows × 1 columns
The duplicated rows are dropped, and their values are summed together.
Now, let’s visualize it again.
7. Extract Most Popular N-Grams From News Titles
Extracting n-grams from the news titles or normalizing the URL words and forming n-grams for understanding the overall topicality is useful to understand which news publication approaches which topic. Here’s how.
import nltk import unicodedata import re def text_clean(content):
lemmetizer = nltk.stem.WordNetLemmatizer() stopwords = nltk.corpus.stopwords.words('english') content = (unicodedata.normalize('NFKD', content) .encode('ascii', 'ignore') .decode('utf-8', 'ignore') .lower()) words = re.sub(r'[^ws]', '', content).split() return [lemmetizer.lemmatize(word) for word in words if word not in stopwords]
raw_words = text_clean(''.join(str(df_bbc['news_title'].tolist())))
raw_words[:10]
OUTPUT>>> ['oneminute', 'world', 'news', 'best', 'generation', 'make', 'agyarkos', 'dream', 'fight', 'card']
The output shows we have “lemmatized” all the words in the news titles and put them in a list.
The list comprehension provides a quick shortcut for filtering every stop word easily.
Using “nltk.corpus.stopwords.words(“english”)” provides all the stop words in English.
But you can add extra stop words to the list to expand the exclusion of words.
The “unicodedata” is to canonicalize the characters.
The characters that we see are actually Unicode bytes like “U+2160 ROMAN NUMERAL ONE” and the Roman Character “U+0049 LATIN CAPITAL LETTER I” are actually the same.
The “unicodedata.normalize” distinguishes the character differences so that the lemmatizer can differentiate the different words with similar characters from each other.
pd.set_option("display.max_colwidth",90) bbc_bigrams = (pd.Series(ngrams(words, n = 2)).value_counts())[:15].sort_values(ascending=False).to_frame() bbc_trigrams = (pd.Series(ngrams(words, n = 3)).value_counts())[:15].sort_values(ascending=False).to_frame()
Below, you will see the most popular “n-grams” from BBC News.

To simply visualize the most popular n-grams of a news source, use the code block below.
bbc_bigrams.plot.barh(color="red", width=.8,figsize=(10 , 7))
“Ukraine, war” is the trending news.
You can also filter the n-grams for “Ukraine” and create an “entity-attribute” pair.

Crawling these URLs and recognizing the “person type entities” can give you an idea about how BBC approaches newsworthy situations.
But it is beyond “news sitemaps.” Thus, it is for another day.
To visualize the popular n-grams from news source’s sitemaps, you can create a custom python function as below.
def ngram_visualize(dataframe:pd.DataFrame, color:str="blue") -> pd.DataFrame.plot: dataframe.plot.barh(color=color, width=.8,figsize=(10 ,7)) ngram_visualize(ngram_extractor(df_dailymail))
The result is below.

To make it interactive, add an extra parameter as below.
def ngram_visualize(dataframe:pd.DataFrame, backend:str, color:str="blue", ) -> pd.DataFrame.plot: if backend=="plotly": pd.options.plotting.backend=backend return dataframe.plot.bar() else: return dataframe.plot.barh(color=color, width=.8,figsize=(10 ,7))
ngram_visualize(ngram_extractor(df_dailymail), backend="plotly")
As a quick example, check below.
8. Create Your Own Custom Functions To Analyze The News Source Sitemaps
When you audit news sitemaps repeatedly, there will be a need for a small Python package.
Below, you can find four different quick Python function chain that uses every previous function as a callback.
To clean a textual content item, use the function below.
def text_clean(content): lemmetizer = nltk.stem.WordNetLemmatizer() stopwords = nltk.corpus.stopwords.words('english') content = (unicodedata.normalize('NFKD', content) .encode('ascii', 'ignore') .decode('utf-8', 'ignore') .lower()) words = re.sub(r'[^ws]', '', content).split() return [lemmetizer.lemmatize(word) for word in words if word not in stopwords]
To extract the n-grams from a specific news website’s sitemap’s news titles, use the function below.
def ngram_extractor(dataframe:pd.DataFrame|pd.Series): if "news_title" in dataframe.columns: return dataframe_ngram_extractor(dataframe, ngram=3, first=10)
Use the function below to turn the extracted n-grams into a data frame.
def dataframe_ngram_extractor(dataframe:pd.DataFrame|pd.Series, ngram:int, first:int): raw_words = text_clean(''.join(str(dataframe['news_title'].tolist()))) return (pd.Series(ngrams(raw_words, n = ngram)).value_counts())[:first].sort_values(ascending=False).to_frame()
To extract multiple news websites’ sitemaps, use the function below.
def ngram_df_constructor(df_1:pd.DataFrame, df_2:pd.DataFrame): df_1_bigrams = dataframe_ngram_extractor(df_1, ngram=2, first=500) df_1_trigrams = dataframe_ngram_extractor(df_1, ngram=3, first=500) df_2_bigrams = dataframe_ngram_extractor(df_2, ngram=2, first=500) df_2_trigrams = dataframe_ngram_extractor(df_2, ngram=3, first=500) ngrams_df = { "df_1_bigrams":df_1_bigrams.index, "df_1_trigrams": df_1_trigrams.index, "df_2_bigrams":df_2_bigrams.index, "df_2_trigrams": df_2_trigrams.index, } dict_df = (pd.DataFrame({ key:pd.Series(value) for key, value in ngrams_df.items() }).reset_index(drop=True) .rename(columns={"df_1_bigrams":adv.url_to_df(df_1["loc"])["netloc"][1].split("www.")[1].split(".")[0] + "_bigrams", "df_1_trigrams":adv.url_to_df(df_1["loc"])["netloc"][1].split("www.")[1].split(".")[0] + "_trigrams", "df_2_bigrams": adv.url_to_df(df_2["loc"])["netloc"][1].split("www.")[1].split(".")[0] + "_bigrams", "df_2_trigrams": adv.url_to_df(df_2["loc"])["netloc"][1].split("www.")[1].split(".")[0] + "_trigrams"})) return dict_df
Below, you can see an example use case.
ngram_df_constructor(df_bbc, df_guardian)

Only with these nested four custom python functions can you do the things below.
- Easily, you can visualize these n-grams and the news website counts to check.
- You can see the focus of the news websites for the same topic or different topics.
- You can compare their wording or the vocabulary for the same topics.
- You can see how many different sub-topics from the same topics or entities are processed in a comparative way.
I didn’t put the numbers for the frequencies of the n-grams.
But, the first ranked ones are the most popular ones from that specific news source.
To examine the next 500 rows, click here.
9. Extract The Most Used News Keywords From News Sitemaps
When it comes to news keywords, they are surprisingly still active on Google.
For example, Microsoft Bing and Google do not think that “meta keywords” are a useful signal anymore, unlike Yandex.
But, news keywords from the news sitemaps are still used.
Among all these news sources, only The Guardian uses the news keywords.
And understanding how they use news keywords to provide relevance is useful.
df_guardian["news_keywords"].str.split().explode().value_counts().to_frame().rename(columns={"news_keywords":"news_keyword_occurence"})
You can see the most used words in the news keywords for The Guardian.
news_keyword_occurence |
|
---|---|
news, |
250 |
World |
142 |
and |
142 |
Ukraine, |
127 |
UK |
116 |
... |
... |
Cumberbatch, |
1 |
Dune |
1 |
Saracens |
1 |
Pearson, |
1 |
Thailand |
1 |
1409 rows × 1 column
The visualization is below.
(df_guardian["news_keywords"].str.split().explode().value_counts() .to_frame().rename(columns={"news_keywords":"news_keyword_occurence"}) .head(25).plot.barh(figsize=(10,8), title="The Guardian Most Used Words in News Keywords", xlabel="News Keywords", legend=False, ylabel="Count of News Keyword"))

The “,” at the end of the news keywords represent whether it is a separate value or part of another.
I suggest you not remove the “punctuations” or “stop words” from news keywords so that you can see their news keyword usage style better.
For a different analysis, you can use “,” as a separator.
df_guardian["news_keywords"].str.split(",").explode().value_counts().to_frame().rename(columns={"news_keywords":"news_keyword_occurence"})
The result difference is below.
news_keyword_occurence |
|
---|---|
World news |
134 |
Europe |
116 |
UK news |
111 |
Sport |
109 |
Russia |
90 |
... |
... |
Women's shoes |
1 |
Men's shoes |
1 |
Body image |
1 |
Kae Tempest |
1 |
Thailand |
1 |
1080 rows × 1 column
Focus on the “split(“,”).”
(df_guardian["news_keywords"].str.split(",").explode().value_counts() .to_frame().rename(columns={"news_keywords":"news_keyword_occurence"}) .head(25).plot.barh(figsize=(10,8), title="The Guardian Most Used Words in News Keywords", xlabel="News Keywords", legend=False, ylabel="Count of News Keyword"))
You can see the result difference for visualization below.

From “Chelsea” to “Vladamir Putin” or “Ukraine War” and “Roman Abramovich,” most of these phrases align with the early days of Russia’s Invasion of Ukraine.
Use the code block below to visualize two different news website sitemaps’ news keywords interactively.
df_1 = df_guardian["news_keywords"].str.split(",").explode().value_counts().to_frame().rename(columns={"news_keywords":"news_keyword_occurence"}) df_2 = df_nyt["news_keywords"].str.split(",").explode().value_counts().to_frame().rename(columns={"news_keywords":"news_keyword_occurence"}) fig = make_subplots(rows = 1, cols = 2) fig.add_trace( go.Bar(y = df_1["news_keyword_occurence"][:6].index, x = df_1["news_keyword_occurence"], orientation="h", name="The Guardian News Keywords"), row=1, col=2 ) fig.add_trace( go.Bar(y = df_2["news_keyword_occurence"][:6].index, x = df_2["news_keyword_occurence"], orientation="h", name="New York Times News Keywords"), row=1, col=1 ) fig.update_layout(height = 800, width = 1200, title_text="Side by Side Popular News Keywords") fig.show() fig.write_html("news_keywords.html")
You can see the result below.
To interact with the live chart, click here.
In the next section, you will find two different subplot samples to compare the n-grams of the news websites.
10. Create Subplots For Comparing News Sources
Use the code block below to put the news sources’ most popular n-grams from the news titles to a sub-plot.
import matplotlib.pyplot as plt import pandas as pd df1 = ngram_extractor(df_bbc) df2 = ngram_extractor(df_skynews) df3 = ngram_extractor(df_dailymail) df4 = ngram_extractor(df_guardian) df5 = ngram_extractor(df_nyt) df6 = ngram_extractor(df_cnn) nrow=3 ncol=2 df_list = [df1 ,df2, df3, df4, df5, df6] #df6 titles = ["BBC News Trigrams", "Skynews Trigrams", "Dailymail Trigrams", "The Guardian Trigrams", "New York Times Trigrams", "CNN News Ngrams"] fig, axes = plt.subplots(nrow, ncol, figsize=(25,32)) count=0 i = 0 for r in range(nrow): for c in range(ncol): (df_list[count].plot.barh(ax = axes[r,c], figsize = (40, 28), title = titles[i], fontsize = 10, legend = False, xlabel = "Trigrams", ylabel = "Count")) count+=1 i += 1
You can see the result below.

The example data visualization above is entirely static and doesn’t provide any interactivity.
Lately, Elias Dabbas, creator of Advertools, has shared a new script to take the article count, n-grams, and their counts from the news sources.
Check here for a better, more detailed, and interactive data dashboard.
The example above is from Elias Dabbas, and he demonstrates how to take the total article count, most frequent words, and n-grams from news websites in an interactive way.
Final Thoughts On News Sitemap Analysis With Python
This tutorial was designed to provide an educational Python coding session to take the keywords, n-grams, phrase patterns, languages, and other kinds of SEO-related information from news websites.
News SEO heavily relies on quick reflexes and always-on article creation.
Tracking your competitors’ angles and methods for covering a topic shows how the competitors have quick reflexes for the search trends.
Creating a Google Trends Dashboard and News Source Ngram Tracker for a comparative and complementary news SEO analysis would be better.
In this article, from time to time, I have put custom functions or advanced for loops, and sometimes, I have kept things simple.
Beginners to advanced Python practitioners can benefit from it to improve their tracking, reporting, and analyzing methodologies for news SEO and beyond.
More resources:
Featured Image: BestForBest/Shutterstock
SEO
Google March 2024 Core Update Officially Completed A Week Ago
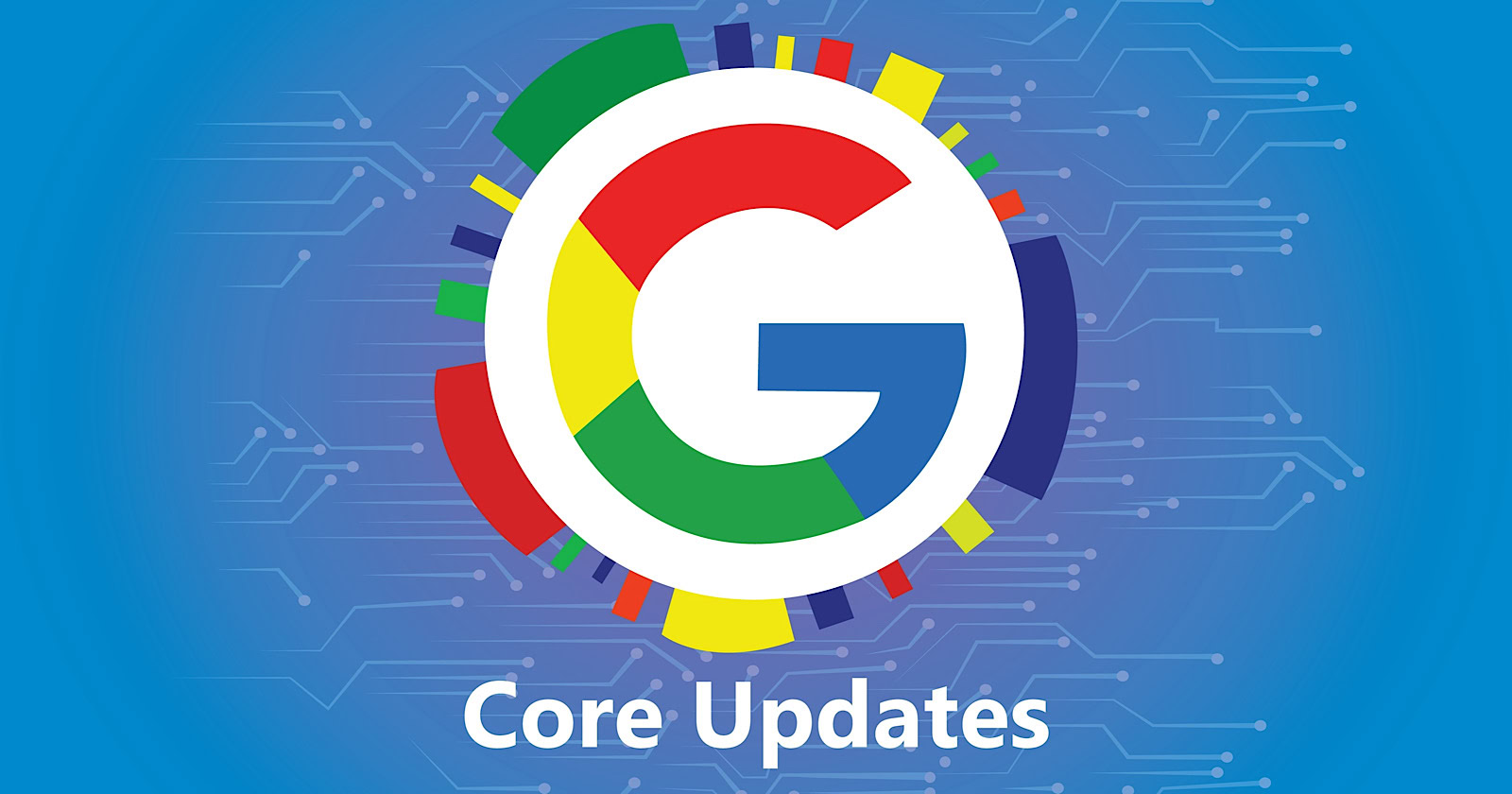
Google has officially completed its March 2024 Core Update, ending over a month of ranking volatility across the web.
However, Google didn’t confirm the rollout’s conclusion on its data anomaly page until April 26—a whole week after the update was completed on April 19.
Many in the SEO community had been speculating for days about whether the turbulent update had wrapped up.
The delayed transparency exemplifies Google’s communication issues with publishers and the need for clarity during core updates
Google March 2024 Core Update Timeline & Status
First announced on March 5, the core algorithm update is complete as of April 19. It took 45 days to complete.
Unlike more routine core refreshes, Google warned this one was more complex.
Google’s documentation reads:
“As this is a complex update, the rollout may take up to a month. It’s likely there will be more fluctuations in rankings than with a regular core update, as different systems get fully updated and reinforce each other.”
The aftershocks were tangible, with some websites reporting losses of over 60% of their organic search traffic, according to data from industry observers.
The ripple effects also led to the deindexing of hundreds of sites that were allegedly violating Google’s guidelines.
Addressing Manipulation Attempts
In its official guidance, Google highlighted the criteria it looks for when targeting link spam and manipulation attempts:
- Creating “low-value content” purely to garner manipulative links and inflate rankings.
- Links intended to boost sites’ rankings artificially, including manipulative outgoing links.
- The “repurposing” of expired domains with radically different content to game search visibility.
The updated guidelines warn:
“Any links that are intended to manipulate rankings in Google Search results may be considered link spam. This includes any behavior that manipulates links to your site or outgoing links from your site.”
John Mueller, a Search Advocate at Google, responded to the turbulence by advising publishers not to make rash changes while the core update was ongoing.
However, he suggested sites could proactively fix issues like unnatural paid links.
“If you have noticed things that are worth improving on your site, I’d go ahead and get things done. The idea is not to make changes just for search engines, right? Your users will be happy if you can make things better even if search engines haven’t updated their view of your site yet.”
Emphasizing Quality Over Links
The core update made notable changes to how Google ranks websites.
Most significantly, Google reduced the importance of links in determining a website’s ranking.
In contrast to the description of links as “an important factor in determining relevancy,” Google’s updated spam policies stripped away the “important” designation, simply calling links “a factor.”
This change aligns with Google’s Gary Illyes’ statements that links aren’t among the top three most influential ranking signals.
Instead, Google is giving more weight to quality, credibility, and substantive content.
Consequently, long-running campaigns favoring low-quality link acquisition and keyword optimizations have been demoted.
With the update complete, SEOs and publishers are left to audit their strategies and websites to ensure alignment with Google’s new perspective on ranking.
Core Update Feedback
Google has opened a ranking feedback form related to this core update.
You can use this form until May 31 to provide feedback to Google’s Search team about any issues noticed after the core update.
While the feedback provided won’t be used to make changes for specific queries or websites, Google says it may help inform general improvements to its search ranking systems for future updates.
Google also updated its help documentation on “Debugging drops in Google Search traffic” to help people understand ranking changes after a core update.
Featured Image: Rohit-Tripathi/Shutterstock
FAQ
After the update, what steps should websites take to align with Google’s new ranking criteria?
After Google’s March 2024 Core Update, websites should:
- Improve the quality, trustworthiness, and depth of their website content.
- Stop heavily focusing on getting as many links as possible and prioritize relevant, high-quality links instead.
- Fix any shady or spam-like SEO tactics on their sites.
- Carefully review their SEO strategies to ensure they follow Google’s new guidelines.
SEO
Google Declares It The “Gemini Era” As Revenue Grows 15%
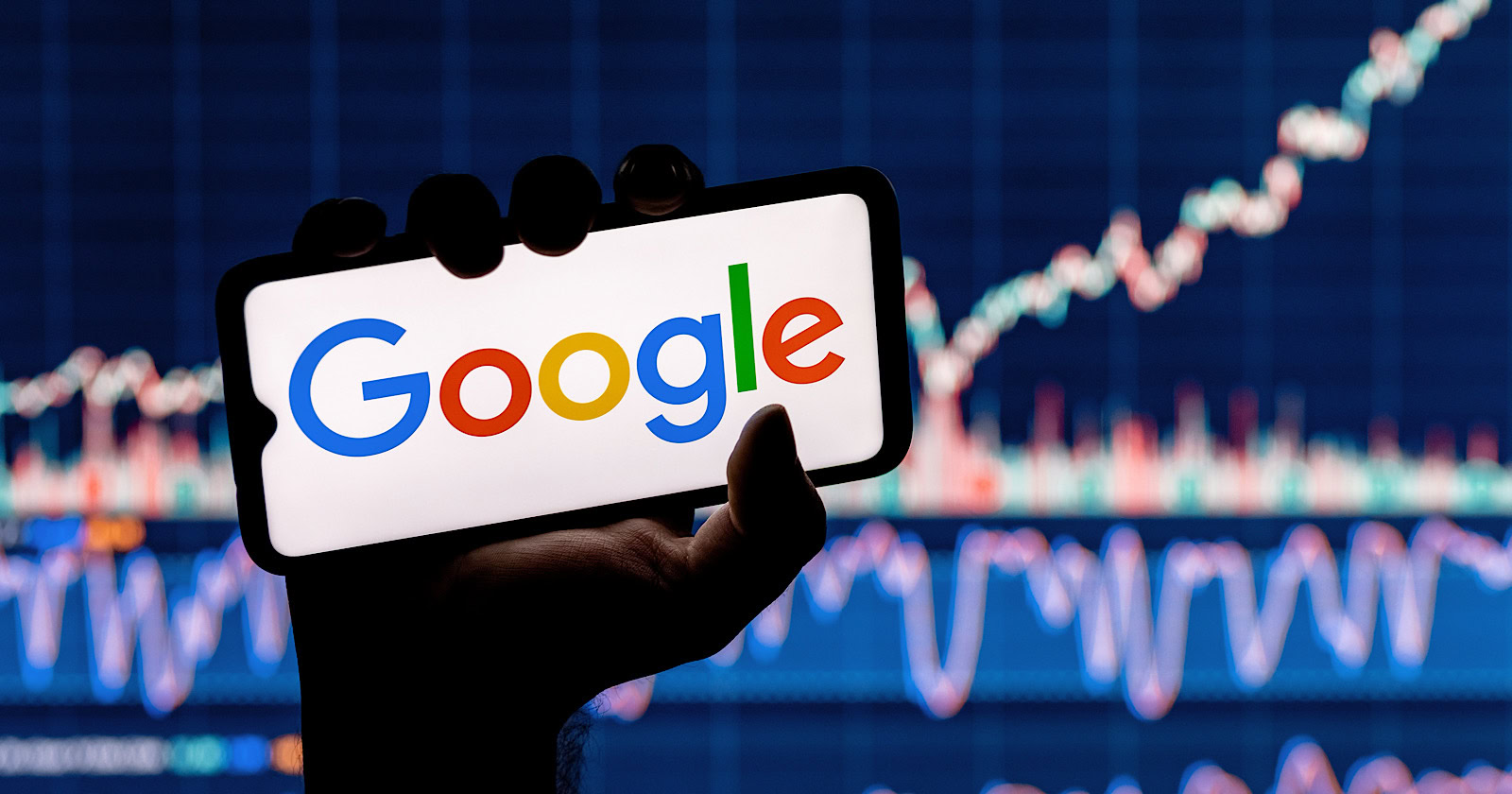
Alphabet Inc., Google’s parent company, announced its first quarter 2024 financial results today.
While Google reported double-digit growth in key revenue areas, the focus was on its AI developments, dubbed the “Gemini era” by CEO Sundar Pichai.
The Numbers: 15% Revenue Growth, Operating Margins Expand
Alphabet reported Q1 revenues of $80.5 billion, a 15% increase year-over-year, exceeding Wall Street’s projections.
Net income was $23.7 billion, with diluted earnings per share of $1.89. Operating margins expanded to 32%, up from 25% in the prior year.
Ruth Porat, Alphabet’s President and CFO, stated:
“Our strong financial results reflect revenue strength across the company and ongoing efforts to durably reengineer our cost base.”
Google’s core advertising units, such as Search and YouTube, drove growth. Google advertising revenues hit $61.7 billion for the quarter.
The Cloud division also maintained momentum, with revenues of $9.6 billion, up 28% year-over-year.
Pichai highlighted that YouTube and Cloud are expected to exit 2024 at a combined $100 billion annual revenue run rate.
Generative AI Integration in Search
Google experimented with AI-powered features in Search Labs before recently introducing AI overviews into the main search results page.
Regarding the gradual rollout, Pichai states:
“We are being measured in how we do this, focusing on areas where gen AI can improve the Search experience, while also prioritizing traffic to websites and merchants.”
Pichai reports that Google’s generative AI features have answered over a billion queries already:
“We’ve already served billions of queries with our generative AI features. It’s enabling people to access new information, to ask questions in new ways, and to ask more complex questions.”
Google reports increased Search usage and user satisfaction among those interacting with the new AI overview results.
The company also highlighted its “Circle to Search” feature on Android, which allows users to circle objects on their screen or in videos to get instant AI-powered answers via Google Lens.
Reorganizing For The “Gemini Era”
As part of the AI roadmap, Alphabet is consolidating all teams building AI models under the Google DeepMind umbrella.
Pichai revealed that, through hardware and software improvements, the company has reduced machine costs associated with its generative AI search results by 80% over the past year.
He states:
“Our data centers are some of the most high-performing, secure, reliable and efficient in the world. We’ve developed new AI models and algorithms that are more than one hundred times more efficient than they were 18 months ago.
How Will Google Make Money With AI?
Alphabet sees opportunities to monetize AI through its advertising products, Cloud offerings, and subscription services.
Google is integrating Gemini into ad products like Performance Max. The company’s Cloud division is bringing “the best of Google AI” to enterprise customers worldwide.
Google One, the company’s subscription service, surpassed 100 million paid subscribers in Q1 and introduced a new premium plan featuring advanced generative AI capabilities powered by Gemini models.
Future Outlook
Pichai outlined six key advantages positioning Alphabet to lead the “next wave of AI innovation”:
- Research leadership in AI breakthroughs like the multimodal Gemini model
- Robust AI infrastructure and custom TPU chips
- Integrating generative AI into Search to enhance the user experience
- A global product footprint reaching billions
- Streamlined teams and improved execution velocity
- Multiple revenue streams to monetize AI through advertising and cloud
With upcoming events like Google I/O and Google Marketing Live, the company is expected to share further updates on its AI initiatives and product roadmap.
Featured Image: Sergei Elagin/Shutterstock
SEO
brightonSEO Live Blog
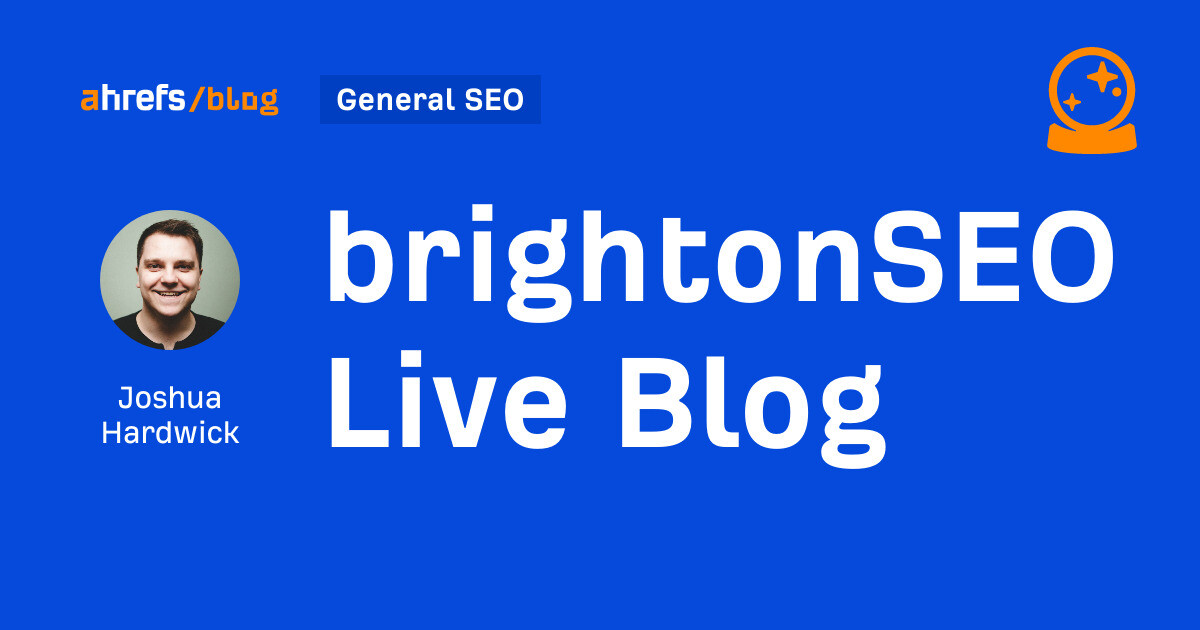
Hello everyone. It’s April again, so I’m back in Brighton for another two days of Being the introvert I am, my idea of fun isn’t hanging around our booth all day explaining we’ve run out of t-shirts (seriously, you need to be fast if you want swag!). So I decided to do something useful and live-blog the event instead.
Follow below for talk takeaways and (very) mildly humorous commentary. sun, sea, and SEO!
-
SEARCHENGINES7 days ago
Daily Search Forum Recap: April 19, 2024
-
WORDPRESS6 days ago
13 Best HubSpot Alternatives for 2024 (Free + Paid)
-
WORDPRESS7 days ago
7 Best WooCommerce Points and Rewards Plugins (Free & Paid)
-
MARKETING6 days ago
Battling for Attention in the 2024 Election Year Media Frenzy
-
MARKETING6 days ago
Advertising in local markets: A playbook for success
-
SEO7 days ago
Google Answers Whether Having Two Sites Affects Rankings
-
SEARCHENGINES6 days ago
Google Core Update Flux, AdSense Ad Intent, California Link Tax & More
-
AFFILIATE MARKETING6 days ago
Grab Microsoft Project Professional 2021 for $20 During This Flash Sale
You must be logged in to post a comment Login