MARKETING
What’s Media Mix Modeling? [Marketer’s Guide with Examples]
![What’s Media Mix Modeling? [Marketer’s Guide with Examples] What’s Media Mix Modeling? [Marketer’s Guide with Examples]](https://articles.entireweb.com/wp-content/uploads/2024/03/Whats-Media-Mix-Modeling-Marketers-Guide-with-Examples.jpg)
Have you ever felt in the dark when it comes to understanding the real impact your marketing dollars are having across multiple channels?
Determining where and how conversions are occurring is crucial in optimizing your budget to drive the most impact with your marketing budget. Media mix modeling (MMM) is an analytical approach used to gauge the effectiveness of various marketing channels in driving sales and conversions. This method allows us to decipher the true influence of advertising spend across diverse platforms by accounting for a myriad of factors, both within their control (like media channel spend, promotional strategies) and outside their control (such as economic conditions, competitor actions, and seasonal influences).
One of the key strengths of media mix modeling is its ability to incorporate long-term brand building effects alongside immediate sales impacts, offering a comprehensive view of marketing effectiveness. It helps in identifying which channels are most efficient, how different channels influence each other, and how external factors affect marketing performance.
Media mix modeling is a powerful tool for marketers seeking to optimize their marketing investments. By providing a holistic view of how various factors contribute to sales and conversions, MMM enables data-driven decisions that enhance marketing efficiency and business growth.
In this article, we explore how media mix modeling works, and how businesses can use analytics to drive smarter ad spend decisions.
What Is Media Mix Modeling?
Media mix modeling (MMM) is a type of analysis that measures the impact of media buys across multiple channels, showing the role various elements play in achieving a desired outcome—often a conversion or revenue KPI. With this information, marketing stakeholders are able to make specific adjustments to campaign spend to improve their progress toward reaching a given goal.
Media mix modeling can be used to address common brand marketing questions and pain points, including:
- Which of our marketing efforts are having the biggest impact on reaching our goals—or, more simply—what’s working?
- How big of an impact does seasonality have on our marketing performance?
- How closely is our performance tied to promotional efforts?
- Are shifting consumer trends negatively or positively impacting outcomes?
- Which specific mix of spend allocation drives the highest ROI?
- How will these channels likely perform in the future based on their optimized spend allocation?
“Media mix modeling is a top-down , privacy resilient approach that evaluates how historical media activity, promotions, pricing, seasonality, and uncontrollable factors—such as economic activity—impact key business outcomes such as sales revenue. MMM is a scientific approach to attribution in the sense that it applies statistical methods to analyze and interpret marketing data, providing a systematic understanding of how different marketing channels contribute to overall business goals in the broader context of the market. The quality of insights derived from MMM heavily depends on the quality and granularity of the data used.”
— Annica Nesty, Group Director of Marketing Science at Tinuiti
MMM leverages aggregate data, and can measure both online (digital) and offline (traditional) advertising channel performance, including (but not limited to): paid media channels such as social media channels, traditional print advertising, linear TV advertising, and other performance marketing efforts, organic media, operational factors like promotions, external factors like seasonality, economic conditions, outcome KPIs such as sales revenue, new customers, and conversions.
How Does Media Mix Modeling Work?
The MMM framework is a type of statistical analysis that uses statistical methods and econometric models such as a regression analysis. This econometric model helps analysts determine the strength of relationships between a single dependent variable and an array of independent variables.
Media mix modeling analysis measures the impact of your media spend today, and is also helpful in predicting the future outcome of your marketing investments on a given variable.
Example:
Let’s assume a scenario where our target metric, or dependent variable, is revenue, a critical indicator of business success. We aim to dissect the influence of various marketing initiatives on this revenue. These initiatives, our independent variables, encompass a diverse array of digital advertising campaigns, including those run on TikTok, Instagram, Snapchat, as well as broader Display and Streaming platforms.
The number of independent variables under scrutiny does not dilute our core objective. The mission is to measure the relationship between the marketing endeavors and the revenue they generate. This involves not only identifying the direct contributions of each campaign to revenue but also understanding the nuanced interplay between them by observing how changing aspects of those independent variables impacts the chosen business outcome
What can MMM Measure?
When using MMM to assess campaign success, marketers should leverage statistical methods and econometric models to get the most accurate picture possible. Data quality is essential in achieving an accurate media mix analysis, so take any needed time to clean your data before using it in your analysis.
Key elements an MMM equation can measure include:
- Base and incremental sales volume impact
- Channel effectiveness and return on investment
- Marketing spend saturation
Media Mix Modeling vs. Data-Driven Attribution Modeling
Like media mix modeling, attribution modeling also studies the efficiency of marketing strategies — but there are important differences.
Attribution modeling is a general term that refers to tracking engagement to better understand how specific tactics drive action at the user level. This modeling works well for analyzing specific customer touchpoints, focusing on elements like how a consumer converted, which creative on which channel led to that conversion, and what the expected ROI could be if more ad budget were shifted to that channel.
Media mix modeling takes a higher-level, more comprehensive picture. This modeling isn’t designed to measure user-level engagement like impressions and clicks, rather its primary function is measuring the impact of an entire touchpoint on specific marketing objectives.
Data-driven attribution modeling and MMM each have their own set of strengths. It’s not a matter of one being better than the other, rather one being better-suited to different types of marketing analysis.
For example:
- The precision of the data-driven attribution: Let’s assume you want to invest more spend in a social ad campaign during the holiday season. While MMM is an option for determining where to allocate those dollars, data-driven attribution excels in dissecting the intricate customer journey, offering a microscopic view of user interactions. For instance, if you’re keen on understanding the exact value of a single click from your social media campaign, Data-Driven Attribution can illuminate the path.
- The holistic perspective of the media mix modeling: Media mix modeling, can consider the impact of offline actions and initiatives. Unlike the more narrowly focused attribution models, which might overemphasize the first or last touchpoint, MMM assesses the collective impact of all channels over time. This makes it an indispensable tool for strategic planning and long-term investment decisions in your marketing portfolio.
“Attribution modeling is based on a bottom-up approach while media mix modeling takes a top-down approach. Media mix modeling provides a long-term view of the marketing ROI of media activity, while attribution modeling evaluates individual-level activity to provide a short term view of marketing ROI.”
— Annica Nesty, Group Director of Marketing Science at Tinuiti
Why Does MMM Make Sense for a Post-cookie/Post-IDFA World?
In the post-cookie and post-IDFA landscape, where privacy concerns and regulatory changes limit access to individual user-level data, media mix modeling has become a pivotal analytical tool. MMM’s emphasis on overall marketing spend allocation and its proficiency in establishing cause-and-effect models, address the challenges posed by the diminishing availability of explicit conversion information, providing marketers with a privacy-respecting and insightful approach to navigate the evolving digital advertising ecosystem.
An Example of Media Mix Modeling
With the right media mix model, a business can measure their past marketing performance to improve future ROI by optimizing the allocation of the media budget by channel and/or tactic, including: traditional and digital media channels, promotions, pricing, competitor spend, economic conditions, weather, and more.
Example:
An international ecommerce brand wanted to forecast their second-half of the year and create an optimal media mix to make their marketing dollars work smarter. A combination of client data, marketing data, and machine learning were required to create a powerful, custom media mix model.
To build the model, the business used 2+ years of digital marketing and revenue data, analyzing it by market, tactic, and day. The data was then used to create model to assess future spend showing how changes in investment across channels could impact revenue and sales.
![What’s Media Mix Modeling? [Marketer’s Guide with Examples] media mix modeling](https://articles.entireweb.com/wp-content/uploads/2024/03/1710719763_910_Whats-Media-Mix-Modeling-Marketers-Guide-with-Examples.png)
The full digital media mix model gave the ecommerce brand a detailed analysis of where to optimize their spend across all digital marketing channels.
One recommendation was to shift dollars away from social—which historically had been at or near 30%—to paid search. This recommendation came with another layer of insight: The brand realized they were overinvesting in awareness campaigns, and needed to invest more heavily in capturing current demand during the 2nd half of the year.
Results: Working with a robust media mix model, the brand was able to break down how much media spend was needed by each channel in order to achieve the 30% YoY revenue goal they targeted.
The Benefits & Challenges of Media Mix Modeling
MMM helps you accurately connect all the dots, leveraging (ideally) a wealth of provided data, to understand how disparate aspects of marketing campaigns work together in helping you reach your business goals.
Benefits of Media Mix Modeling
The benefits of MMM are multifaceted, offering marketers a strategic edge in navigating the intricacies of their advertising efforts. Let’s dive into each benefit in detail…
Omnichannel Campaigns: MMM excels in providing insights for omnichannel campaigns, allowing marketers to understand and optimize the impact of their initiatives across various channels. This capability is crucial in today’s interconnected digital landscape, where consumers engage with brands through diverse platforms.
Improved Oversight Over Media Spend Impact: MMM provides a comprehensive view of the impact of media spend, enabling marketers to assess the effectiveness of their investments. This improved oversight ensures a clearer understanding of how each component of the media mix contributes to overall campaign success.
Media Spend Optimization: With MMM, marketers can optimize their media spend by identifying the most impactful channels and touchpoints. This data-driven approach allows for strategic adjustments in budget allocation, ensuring that resources are directed towards the avenues that yield the highest return on ad spend.
Effective Targeting of Audiences: MMM’s analysis helps in refining audience targeting strategies. By understanding which elements of the marketing mix resonate most with specific demographics, marketers can tailor their campaigns to effectively reach and engage their target audience segments.
Forecasting with Certainty: One of MMM’s strengths lies in its ability to forecast results with a high degree of certainty. This forecasting capability empowers marketers to make informed decisions based on predictive analytics, aiding in long-term planning and goal setting.
Reduced Reliance on Personally Identifiable Information (PII): MMM minimizes the reliance on personally identifiable information for analysis. This is especially crucial in an era where privacy concerns are more important than ever.
Media mix modeling is a comprehensive and powerful tool, offering a range of benefits that contribute to a more effective, data-driven, and privacy-conscious approach to marketing strategy and decision-making. While there are many benefits to MMM, there are challenges as well. Let’s look into common challenges of MMM in our next section.
Challenges of Media Mix Modeling
MMM grows increasingly complex as the media landscape becomes more fragmented, and the customer journey more personalized. Whereas in the past, advertisers may have wanted to measure something as simple as the impact of a print ad in a Cleveland newspaper, today’s consumers are exposed to brands in a wide variety of locations and formats, from a subway transit poster to a Sponsored post on Instagram.
Working with high-quality data is important in any measurement initiative, but for MMM to work effectively, it also needs a lot of data to build a reliable model. For example, if you wanted your model to consider the performance impact of seasonality, it would ideally need at least three full seasons (three years) of data to consider in its analysis.
This makes media mix modeling a ‘long game’ initiative with infrequent reporting by its nature. Brands and advertisers who are more accustomed to daily or weekly updates may struggle with ‘waiting out’ the analysis.
Because it’s not designed to make considerations based on user-level data, instead providing aggregate insights, media mix modeling offers limited insights on brand impact, personalized targeting, and customer experience. However, advanced models are available that can provide highly granular insights, but traditional MMM provides aggregate insights.
Common Misconceptions About Media Mix Modeling
Media mix modeling, like many other analytics solutions, has also become a marketing buzzword that has generated its fair share of misconceptions.
Here are a few of the most common misconceptions around media mix modeling.
Media Mix Models Are Not Transparent
With large datasets and statistical analysis involved in media mix modeling, the methods behind the technique have been critiqued for their obscurity. If there is no perceived transparency in the process, how does a brand know if its media mix model is really accurate?
Any organization specializing in media mix modeling should provide a transparent approach, with deliverables such as outlines, milestones, and performance reports. Additionally, you may want to consider partnering with an agency that truly understands how media mix modeling aligns with your needs and expectations. Every business is unique and each media mix model is based on multiple factors.
Media Mix Models Do Not Provide Real-time Data
Today, results are often measured by the timeliness of their delivery, with the current digital marketplace allowing for almost instantaneous real-time data. Media mix models do actually provide compelling real-time marketing insights, perfect for evaluating new campaigns, new competitors, and assessing pricing actions or changes in promotional strategies.
A powerful partner in media mix modeling will provide sophisticated tools and real-time approaches to satisfy your business performance assessments. Your partner should also be able to provide forecasting, simulation, or AI- and machine-learning-integrated models to suggest future movements.
Media Mix Modeling is Biased to Offline Channels
Though media mix strategies do integrate and consider offline channels in their approaches, media mix modeling also considers all digital channels — including display, email, paid search, social, and more. Remember—it’s considering your media mix. If that includes ten different channels and you provide enough high-quality data for each, they will all be considered in your marketing mix analysis.
In fact, as customers have become more intertwined with digital channels, media marketing models have adapted to go even deeper into the analyses provided by those channels’ respective insights to support better budgeting choices and customer segmentation reports.
Conclusion: MMM Closes the Loop on Marketing Performance
In an ever-evolving digital landscape, MMM’s adaptability to the post-cookie/post-IDFA world positions it as an essential tool for marketers. As businesses seek to connect the dots, leverage data, and make strategic decisions, MMM is a crucial ally in the dynamic realm of mixed media advertising.
“At Tinuiti, we leverage measurement best practices such as MMM and incrementality to understand media effectiveness, predict future outcomes, create deeper insights, analyzing what-if scenarios to provide recommendations that optimize media performance. This helps brands understand what channels they should be investing in, how they should shift budgets (media mix), creating a high-level view of what channels are driving overall sales and ROI. Our goal here is to deliver growth for our clients by maximizing the return on investment through best in class measurement”
— Annica Nesty, Group Director of Marketing Science at Tinuiti
At Tinuiti, we know, embrace, and utilize MMM. Our Rapid Media Mix Modeling sets a new standard in the market with its exceptional speed, precision, and transparency.
Our proprietary measurement technology, Bliss Point by Tinuiti, allows us to measure what marketers have previously struggled to measure – the optimal level of investment to maximize impact and efficiency. But this measurement is not just to go back and validate that we’ve done the right things. This measurement is real-time informing what needs to happen next.
Curious about how we can tailor strategies to hit your unique marketing bliss point, including Rapid Media Mix Modeling? We’re eager to chat. Contact us today for details.
MARKETING
The Current State of Google’s Search Generative Experience [What It Means for SEO in 2024]
![The Current State of Google’s Search Generative Experience [What It Means for SEO in 2024] person typing on laptop with](https://articles.entireweb.com/wp-content/uploads/2024/04/The-Current-State-of-Googles-Search-Generative-Experience-What-It.webp.webp)
SEO enthusiasts, known for naming algorithm updates after animals and embracing melodrama, find themselves in a landscape where the “adapt or die” mantra prevails. So when Google announced the launch of its Search Generative Experience (SGE) in May of 2023 at Google/IO, you can imagine the reaction was immense.
Although SGE has the potential to be a truly transformative force in the landscape, we’re still waiting for SGE to move out of the Google Labs Sandbox and integrate into standard search results.
Curious about our current take on SGE and its potential impact on SEO in the future? Read on for more.
Decoding Google’s Defensive Move
In response to potential threats from competitors like ChatGPT, Bing, TikTok, Reddit, and Amazon, Google introduced SGE as a defensive maneuver. However, its initial beta release raised questions about its readiness and global deployment.
ChatGPT provided an existential threat that had the potential to eat into Google’s market share. When Bing started incorporating it into its search results, it was one of the most significant wins for Bing in a decade. In combination with threats from TikTok, Reddit, and Amazon, we see a more fractured search landscape less dominated by Google. Upon its launch, the expectation was that Google would push its SGE solution globally, impact most queries, and massively shake up organic search results and strategies to improve organic visibility.
Now, industry leaders are starting to question if Google is better off leaving SGE in the testing ground in Google labs. According to Google’s recent update, it appears that SGE will remain an opt-in experience in Google Labs (for at least the short term). If SGE was released, there could be a fundamental reset in understanding SEO. Everything from organic traffic to optimization tactics to tracking tools would need adjustments for the new experience. Therefore, the prospect of SGE staying in Google Labs is comforting if not entirely reliable.
The ever-present option is that Google can change its mind at any point and push SGE out broadly as part of its standard search experience. For this reason, we see value in learning from our observations with SGE and continuing to stay on top of the experience.
SGE User Experience and Operational Challenges
If you’ve signed up for search labs and have been experimenting with SGE for a while, you know firsthand there are various issues that Google should address before rolling it out broadly to the public.
At a high level, these issues fall into two broad categories including user experience issues and operational issues.
Below are some significant issues we’ve come across, with Google making notable progress in addressing certain ones, while others still require improvement:
- Load time – Too many AI-generated answers take longer to load than a user is willing to wait. Google recommends less than a 3-second load time to meet expectations. They’ll need to figure out how to consistently return results quickly if they want to see a higher adoption rate.
- Layout – The SGE layout is massive. We believe any major rollout will be more streamlined to make it a less intrusive experience for users and allow more visibility for ads, and if we’re lucky, organic results. Unfortunately, there is still a decent chance that organic results will move below the fold, especially on mobile devices. Recently, Google has incorporated more results where users are prompted to generate the AI result if they’d like to see it. The hope is Google makes this the default in the event of a broad rollout where users can generate an AI result if they want one instead of assuming that’s what a user would like to see.
- Redundancy – The AI result duplicates features from the map pack and quick answer results.
- Attribution – Due to user feedback, Google includes sources on several of their AI-powered overviews where you can see relevant web pages if there is an arrow next to the result. Currently, the best way to appear as one of these relevant pages is to be one of the top-ranked results, which is convenient from an optimization standpoint. Changes to how attribution and sourcing are handled could heavily impact organic strategies.
On the operational side, Google also faces significant hurdles to making SGE a viable product for its traditional search product. The biggest obstacle appears to be making the cost associated with the technology worth the business outcomes it provides. If this was a necessary investment to maintain market share, Google might be willing to eat the cost, but if their current position is relatively stable, Google doesn’t have much of an incentive to take on the additional cost burden of heavily leveraging generative AI while also presumably taking a hit to their ad revenue. Especially since slow user adoption doesn’t indicate this is something users are demanding at the moment.
While the current experience of SGE is including ads above the generative results now, the earliest iterations didn’t heavily feature sponsored ads. While they are now included, the current SGE layout would still significantly disrupt the ad experience we’re used to. During the Google I/O announcement, they made a statement to reassure advertisers they would be mindful of maintaining a distinct ad experience in search.
“In this new generative experience, Search ads will continue to appear in dedicated ad slots throughout the page. And we’ll continue to uphold our commitment to ads transparency and making sure ads are distinguishable from organic search results” – Elizabeth Reid, VP, Search at Google
Google is trying to thread a delicate needle here of staying on the cutting edge with their search features, while trying not to upset their advertisers and needlessly hinder their own revenue stream. Roger Montti details more of the operational issues in a recent article digging into the surprising reasons SGE is stuck in Google Labs.
He lists three big problems that need to be solved before SGE will be integrated into the foreground of search:
- Large Language Models being inadequate as an information retrieval system
- The inefficiency and cost of transformer architecture
- Hallucinating (providing inaccurate answers)
Until SGE provides more user value and checks more boxes on the business sense side, the traditional search experience is here to stay. Unfortunately, we don’t know when or if Google will ever feel confident they’ve addressed all of these concerns, so we’ll need to stay prepared for change.
Experts Chime in on Search Generative Experience
Our team has been actively engaging with SGE, here’s a closer look at their thoughts and opinions on the experience so far:
“With SGE still in its early stages, I’ve noticed consistent changes in how the generative results are produced and weaved naturally into the SERPs. Because of this, I feel it is imperative to stay on top of these on-going changes to ensure we can continue to educate our clients on what to expect when SGE is officially incorporated into our everyday lives. Although an official launch date is currently unknown, I believe proactively testing various prompt types and recording our learnings is important to prepare our clients for this next evolution of Google search.”
– Jon Pagano, SEO Sr. Specialist at Tinuiti
“It’s been exciting to watch SGE grow through different variations over the last year, but like other AI solutions its potential still outweighs its functionality and usefulness. What’s interesting to see is that SGE doesn’t just cite its sources of information, but also provides an enhanced preview of each webpage referenced. This presents a unique organic opportunity where previously untouchable top 10 rankings are far more accessible to the average website. Time will tell what the top ranking factors for SGE are, but verifiable content with strong E-E-A-T signals will be imperative.”
–Kate Fischer, SEO Specialist at Tinuiti
“Traditionally, AI tools were very good at analytical tasks. With the rise of ChatGPT, users can have long-form, multi-question conversations not yet available in search results. When, not if, released, Google’s Generative Experience will transform how we view AI and search. Because there are so many unknowns, some of the most impactful ways we prepare our clients are to discover and develop SEO strategies that AI tools can’t directly disrupt, like mid to low funnel content.”
– Brandon Miller, SEO Specialist at Tinuiti
“SGE is going to make a huge impact on the ecommerce industry by changing the way users interact with the search results. Improved shopping experience will allow users to compare products, price match, and read reviews in order to make it quicker and easier for a user to find the best deals and purchase. Although this leads to more competitive results, it also improves organic visibility and expands our product reach. It is more important than ever to ensure all elements of a page are uniquely and specifically optimized for search. With the SGE updates expected to continue to impact search results, the best way to stay ahead is by focusing on strong user focused content and detailed product page optimizations.”
– Kellie Daley, SEO Sr. Specialist at Tinuiti
Navigating the Clash of Trends
One of the most interesting aspects of the generative AI trend in search is that it appears to be in direct opposition to other recent trends.
One of the ways Google has historically evaluated the efficacy of its search ranking systems is through the manual review of quality raters. In their quality rater guidelines, raters were instructed to review for things like expertise, authority, and trustworthiness (EAT) in results to determine if Google results are providing users the information they deserve.
In 2022, Google updated their search guidelines to include another ‘e’ in the form of experience (EEAT). In their words, Google wanted to better assess if the content a user was consuming was created by someone with, “a degree of experience, such as with actual use of a product, having actually visited a place or communicating what a person has experienced. There are some situations where really what you value most is content produced by someone who has firsthand, life experience on the topic at hand.”
Generative AI results, while cutting-edge technology and wildly impressive in some cases, stand in direct opposition to the principles of E-E-A-T. That’s not to say that there’s no room for both in search, but Google will have to determine what it thinks users value more between these competing trends. The slow adoption of SGE could be an indication that a preference for human experience, expertise, authority, and trust is winning round one in this fight.
Along these lines, Google is also diversifying its search results to cater to the format in which users get their information. This takes the form of their Perspectives Filter. Also announced at Google I/O 2023, the perspectives filter incorporates more video, image, and discussion board posts from places like TikTok, YouTube, Reddit, and Quora. Once again, this trend shows the emphasis and value searchers place on experience and perspective. Users value individual experience over the impersonal conveyance of information. AI will never have these two things, even if it can provide a convincing imitation.
The current iteration of SGE seems to go too far in dismissing these trends in favor of generative AI. It’s an interesting challenge Google faces. If they don’t determine the prevailing trend correctly, veering too far in one direction can push more market share to ChatGPT or platforms like YouTube and TikTok.
Final Thoughts
The range of outcomes remains broad and fascinating for SGE. We can see this developing in different ways, and prognostication offers little value, but it’s invaluable to know the potential outcomes and prepare for as many of them as possible.
It’s critical that you or your search agency be interacting and experimenting with SGE because:
- The format and results will most likely continue to see significant changes
- This space moves quickly and it’s easy to fall behind
- Google may fix all of the issues with SGE and decide to push it live, changing the landscape of search overnight
- SGE experiments could inform other AI elements incorporated into the search experience
Ultimately, optimizing for the specific SGE experience we see now is less important because we know it will inevitably continue changing. We see more value in recognizing the trends and problems Google is trying to solve with this technology. With how quickly this space moves, any specifics mentioned in this article could be outdated in a week. That’s why focusing on intention and process is important at this stage of the game.
By understanding the future needs and wants SGE is attempting to address, we can help you future-proof your search strategies as much as possible. To some extent we’re always at the whims of the algorithm, but by maintaining a user-centric approach, you can make your customers happy, regardless of how they find you.
MARKETING
How to create editorial guidelines that are useful + template
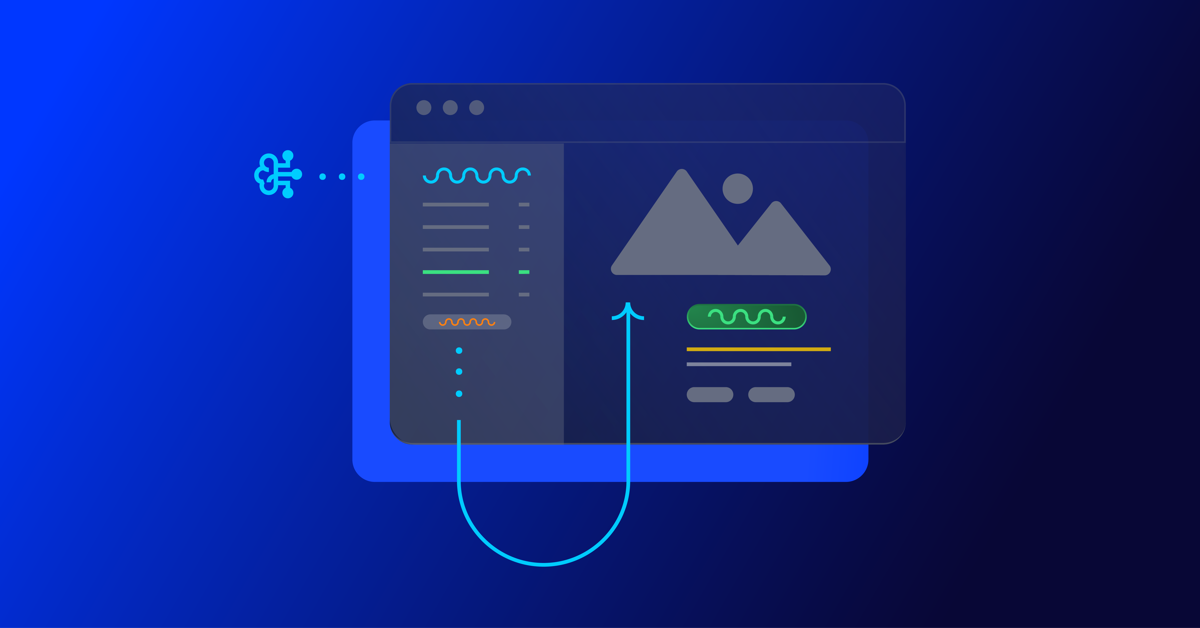
Before diving in to all things editorial guidelines, a quick introduction. I head up the content team here at Optimizely. I’m responsible for developing our content strategy and ensuring this aligns to our key business goals.
Here I’ll take you through the process we used to create new editorial guidelines; things that worked well and tackle some of the challenges that come with any good multi – stakeholder project, share some examples and leave you with a template you can use to set your own content standards.
What are editorial guidelines?
Editorial guidelines are a set of standards for any/all content contributors, etc. etc. This most often includes guidance on brand, tone of voice, grammar and style, your core content principles and the types of content you want to produce.
Editorial guidelines are a core component of any good content strategy and can help marketers achieve the following in their content creation process:
- Consistency: All content produced, regardless of who is creating it, maintains a consistent tone of voice and style, helping strengthen brand image and making it easier for your audience to recognize your company’s content
- Quality Control: Serves as a ‘North Star’ for content quality, drawing a line in the sand to communicate the standard of content we want to produce
- Boosts SEO efforts: Ensures content creation aligns with SEO efforts, improving company visibility and increasing traffic
- Efficiency: With clear guidelines in place, content creators – external and internal – can work more efficiently as they have a clear understanding of what is expected of them
Examples of editorial guidelines
There are some great examples of editorial guidelines out there to help you get started.
Here are a few I used:
1. Editorial Values and Standards, the BBC
Ah, the Beeb. This really helped me channel my inner journalist and learn from the folks that built the foundation for free quality journalism.
How to create editorial guidelines, Pepperland Marketing
After taking a more big picture view I recognized needed more focused guidance on the step by step of creating editorial guidelines.
I really liked the content the good folks at Pepperland Marketing have created, including a free template – thanks guys! – and in part what inspired me to create our own free template as a way of sharing learnings and helping others quickstart the process of creating their own guidelines.
3. Writing guidelines for the role of AI in your newsroom?… Nieman Lab
As well as provide guidance on content quality and the content creation process, I wanted to tackle the thorny topic of AI in our editorial guidelines. Specifically, to give content creators a steer on ‘fair’ use of AI when creating content, to ensure creators get to benefit from the amazing power of these tools, but also that content is not created 100% by AI and help them understand why we feel that contravenes our core content principles of content quality.
So, to learn more I devoured this fascinating article, sourcing guidance from major media outlets around the world. I know things change very quickly when it comes to AI, but I highly encourage reading this and taking inspiration from how these media outlets are tackling this topic.
Learn more: The Marketer’s Guide to AI-generated content
Why did we decide to create editorial guidelines?
1. Aligning content creators to a clear vision and process
Optimizely as a business has undergone a huge transformation over the last 3 years, going through rapid acquisition and all the joys and frustrations that can bring. As a content team, we quickly recognized the need to create a set of clear and engaging guidelines that helps content creators understand how and where they can contribute, and gave a clear process to follow when submitting a content idea for consideration.
2. Reinvigorated approach to brand and content
As a brand Optimizely is also going through a brand evolution – moving from a more formal, considered tone of voice to one that’s much more approachable, down to earth and not afraid to use humor, different in content and execution.
See, our latest CMS campaign creative:
It’s pretty out there in terms of creative and messaging. It’s an ad campaign that’s designed to capture attention yes, but also – to demonstrate our abilities as a marketing team to create this type of campaign that is normally reserved for other more quote unquote creative industries.
We wanted to give guidance to fellow content creators outside the team on how they can also create content that embraces this evolved tone of voice, while at the same time ensuring content adheres to our brand guidelines.
3. Streamline content creation process
Like many global enterprises we have many different content creators, working across different time zones and locations. Documenting a set of guidelines and making them easily available helps content creators quickly understand our content goals, the types of content we want to create and why. It would free up content team time spent with individual contributors reviewing and editing submissions, and would ensure creation and optimization aligns to broader content & business goals.
It was also clear that we needed to document a process for submitting content ideas, so we made sure to include this in the guidelines themselves to make it easy and accessible for all contributors.
4. 2023 retrospective priority
As a content team we regularly review our content strategy and processes to ensure we’re operating as efficiently as possible.
In our last retrospective. I asked my team ‘what was the one thing I could do as a manager to help them be more impactful in their role?’
Editorial guidelines was the number 1 item on their list.
So off we went…
What we did
- Defined a discrete scope of work for the first version of the editorial guidelines, focusing on the Blog and Resources section of the website. This is where the content team spends most of its time and so has most involvement in the content creation process. Also where the most challenging bottlenecks have been in the past
- Research. Reviewed what was out there, got my hands on a few free templates and assembled a framework to create a first version for inputs and feedback
- Asked content community – I put a few questions out to my network on LinkedIn on the topic of content guidelines and content strategy, seeking to get input and guidance from smart marketers.
- Invited feedback: Over the course of a few weekswe invited collaborators to comment in a shared doc as a way of taking iterative feedback, getting ideas for the next scope of work, and also – bringing people on the journey of creating the guidelines. Look at all those reviewers! Doing this within our Content Marketing Platform (CMP) ensured that all that feedback was captured in one place, and that we could manage the process clearly, step by step:
Look at all those collaborators! Thanks guys! And all of those beautiful ticks, so satisfying. So glad I could crop out the total outstanding tasks for this screen grab too (Source – Optimizely CMP)
- Updated content workflow: Now we have clear, documented guidance in place, we’ve included this as a step – the first step – in the workflow used for blog post creation:
Source: Optimizely CMP
Results
It’s early days but we’re already seeing more engagement with the content creation process, especially amongst the teams involved in building the guidelines (which was part of the rationale in the first place :))
Source: My Teams chat
It’s inspired teams to think differently about the types of content we want to produce going forwards – for the blog and beyond.
I’d also say it’s boosted team morale and collaboration, helping different teams work together on shared goals to produce better quality work.
What’s next?
We’re busy planning wider communication of the editorial guidelines beyond marketing. We’ve kept the original draft and regularly share this with existing and potential collaborators for ongoing commentary, ideas and feedback.
Creating guidelines has also sparked discussion about the types of briefs and templates we want and need to create in CMP to support creating different assets. Finding the right balance between creative approach and using templates to scale content production is key.
We’ll review these guidelines on a quarterly basis and evolve as needed, adding new formats and channels as we go.
Key takeaways
- Editorial guidelines are a useful way to guide content creators as part of your overall content strategy
- Taking the time to do research upfront can help accelerate seemingly complex projects. Don’t be afraid to ask your community for inputs and advice as you create
- Keep the scope small at first rather than trying to align everything all at once. Test and learn as you go
- Work with stakeholders to build guidelines from the ground up to ensure you create a framework that is useful, relevant and used
And lastly, here’s that free template we created to help you build or evolve your own editorial guidelines!
MARKETING
Effective Communication in Business as a Crisis Management Strategy
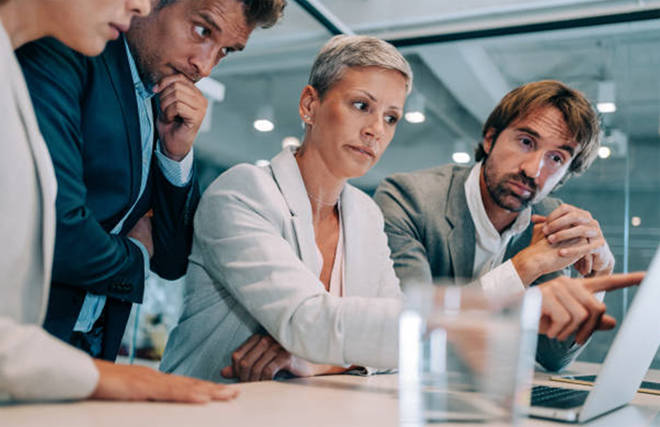
Everyday business life is full of challenges. These include data breaches, product recalls, market downturns and public relations conflicts that can erupt at any moment. Such situations pose a significant threat to a company’s financial health, brand image, or even its further existence. However, only 49% of businesses in the US have a crisis communications plan. It is a big mistake, as such a strategy can build trust, minimize damage, and even strengthen the company after it survives the crisis. Let’s discover how communication can transform your crisis and weather the chaos.
The ruining impact of the crisis on business
A crisis can ruin a company. Naturally, it brings losses. But the actual consequences are far worse than lost profits. It is about people behind the business – they feel the weight of uncertainty and fear. Employees start worrying about their jobs, customers might lose faith in the brand they once trusted, and investors could start looking elsewhere. It can affect the brand image and everything you build from the branding, business logo, social media can be ruined. Even after the crisis recovery, the company’s reputation can suffer, and costly efforts might be needed to rebuild trust and regain momentum. So, any sign of a coming crisis should be immediately addressed. Communication is one of the crisis management strategies that can exacerbate the situation.
The power of effective communication
Even a short-term crisis may have irreversible consequences – a damaged reputation, high employee turnover, and loss of investors. Communication becomes a tool that can efficiently navigate many crisis-caused challenges:
- Improved trust. Crisis is a synonym for uncertainty. Leaders may communicate trust within the company when the situation gets out of control. Employees feel valued when they get clear responses. The same applies to the customers – they also appreciate transparency and are more likely to continue cooperation when they understand what’s happening. In these times, documenting these moments through event photographers can visually reinforce the company’s messages and enhance trust by showing real, transparent actions.
- Reputation protection. Crises immediately spiral into gossip and PR nightmares. However, effective communication allows you to proactively address concerns and disseminate true information through the right channels. It minimizes speculation and negative media coverage.
- Saved business relationships. A crisis can cause unbelievable damage to relationships with employees, customers, and investors. Transparent communication shows the company’s efforts to find solutions and keeps stakeholders informed and engaged, preventing misunderstandings and painful outcomes.
- Faster recovery. With the help of communication, the company is more likely to receive support and cooperation. This collaborative approach allows you to focus on solutions and resume normal operations as quickly as possible.
It is impossible to predict when a crisis will come. So, a crisis management strategy mitigates potential problems long before they arise.
Tips on crafting an effective crisis communication plan.
To effectively deal with unforeseen critical situations in business, you must have a clear-cut communication action plan. This involves things like messages, FAQs, media posts, and awareness of everyone in the company. This approach saves precious time when the crisis actually hits. It allows you to focus on solving the problem instead of intensifying uncertainty and panic. Here is a step-by-step guide.
Identify your crisis scenarios.
Being caught off guard is the worst thing. So, do not let it happen. Conduct a risk assessment to pinpoint potential crises specific to your business niche. Consider both internal and external factors that could disrupt normal operations or damage the online reputation of your company. Study industry-specific issues, past incidents, and current trends. How will you communicate in each situation? Knowing your risks helps you prepare targeted communication strategies in advance. Of course, it is impossible to create a perfectly polished strategy, but at least you will build a strong foundation for it.
Form a crisis response team.
The next step is assembling a core team. It will manage communication during a crisis and should include top executives like the CEO, CFO, and CMO, and representatives from key departments like public relations and marketing. Select a confident spokesperson who will be the face of your company during the crisis. Define roles and responsibilities for each team member and establish communication channels they will work with, such as email, telephone, and live chat. Remember, everyone in your crisis response team must be media-savvy and know how to deliver difficult messages to the stakeholders.
Prepare communication templates.
When a crisis hits, things happen fast. That means communication needs to be quick, too. That’s why it is wise to have ready-to-go messages prepared for different types of crises your company may face. These messages can be adjusted to a particular situation when needed and shared on the company’s social media, website, and other platforms right away. These templates should include frequently asked questions and outline the company’s general responses. Make sure to approve these messages with your legal team for accuracy and compliance.
Establish communication protocols.
A crisis is always chaotic, so clear communication protocols are a must-have. Define trigger points – specific events that would launch the crisis communication plan. Establish a clear hierarchy for messages to avoid conflicting information. Determine the most suitable forms and channels, like press releases or social media, to reach different audiences. Here is an example of how you can structure a communication protocol:
- Immediate alert. A company crisis response team is notified about a problem.
- Internal briefing. The crisis team discusses the situation and decides on the next steps.
- External communication. A spokesperson reaches the media, customers, and suppliers.
- Social media updates. A trained social media team outlines the situation to the company audience and monitors these channels for misinformation or negative comments.
- Stakeholder notification. The crisis team reaches out to customers and partners to inform them of the incident and its risks. They also provide details on the company’s response efforts and measures.
- Ongoing updates. Regular updates guarantee transparency and trust and let stakeholders see the crisis development and its recovery.
Practice and improve.
Do not wait for the real crisis to test your plan. Conduct regular crisis communication drills to allow your team to use theoretical protocols in practice. Simulate different crisis scenarios and see how your people respond to these. It will immediately demonstrate the strong and weak points of your strategy. Remember, your crisis communication plan is not a static document. New technologies and evolving media platforms necessitate regular adjustments. So, you must continuously review and update it to reflect changes in your business and industry.
Wrapping up
The ability to handle communication well during tough times gives companies a chance to really connect with the people who matter most—stakeholders. And that connection is a foundation for long-term success. Trust is key, and it grows when companies speak honestly, openly, and clearly. When customers and investors trust the company, they are more likely to stay with it and even support it. So, when a crisis hits, smart communication not only helps overcome it but also allows you to do it with minimal losses to your reputation and profits.
-
WORDPRESS7 days ago
7 Best WooCommerce Points and Rewards Plugins (Free & Paid)
-
MARKETING7 days ago
Battling for Attention in the 2024 Election Year Media Frenzy
-
WORDPRESS6 days ago
13 Best HubSpot Alternatives for 2024 (Free + Paid)
-
MARKETING6 days ago
Advertising in local markets: A playbook for success
-
SEO7 days ago
Google Answers Whether Having Two Sites Affects Rankings
-
SEARCHENGINES6 days ago
Google Core Update Flux, AdSense Ad Intent, California Link Tax & More
-
AFFILIATE MARKETING7 days ago
Grab Microsoft Project Professional 2021 for $20 During This Flash Sale
-
PPC4 days ago
10 Most Effective Franchise Marketing Strategies