The new app is called watchGPT and as I tipped off already, it gives you access to ChatGPT from your Apple Watch. Now the $10,000 question (or more accurately the $3.99 question, as that is the one-time cost of the app) is why having ChatGPT on your wrist is remotely necessary, so let’s dive into what exactly the app can do.
NEWS
Google KELM Reduces Bias and Improves Factual Accuracy via @sejournal, @martinibuster
Google AI Blog announced KELM, a way that could be used to reduce bias and toxic content in search (open domain question answering). It uses a method called TEKGEN to convert Knowledge Graph facts into natural language text that can then be used to improve natural language processing models.
What is KELM?
KELM is an acronym for Knowledge-Enhanced Language Model Pre-training. Natural language processing models like BERT are typically trained on web and other documents. KELM proposes adding trustworthy factual content (knowledge-enhanced) to the language model pre-training in order to improve the factual accuracy and reduce bias.
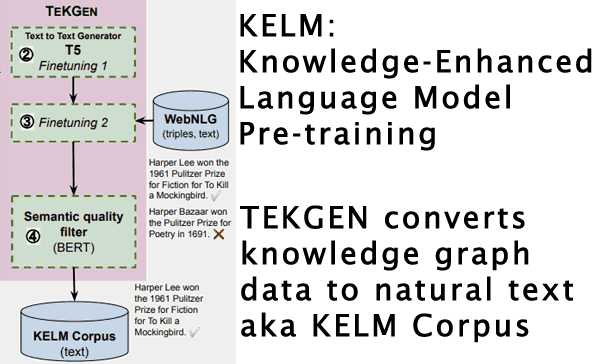
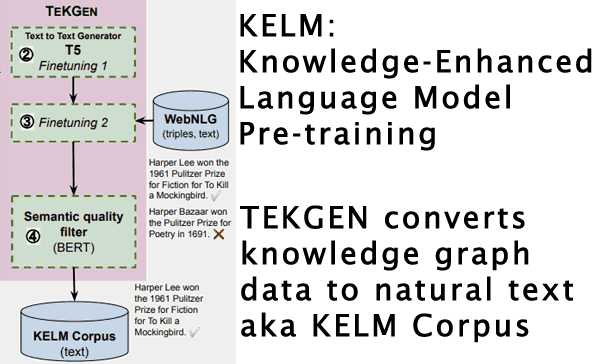
KELM Uses Trustworthy Data
The Google researchers proposed using knowledge graphs for improving factual accuracy because they’re a trusted source of facts.
Advertisement
Continue Reading Below
“Alternate sources of information are knowledge graphs (KGs), which consist of structured data. KGs are factual in nature because the information is usually extracted from more trusted sources, and post-processing filters and human editors ensure inappropriate and incorrect content are removed.”
Is Google Using KELM?
Google has not indicated whether or not KELM is in use. KELM is an approach to language model pre-training that shows strong promise and was summarized on the Google AI blog.
Bias, Factual Accuracy and Search Results
According to the research paper this approach improves factual accuracy:
“It carries the further advantages of improved factual accuracy and reduced toxicity in the resulting language model.”
This research is important because reducing bias and increasing factual accuracy could impact how sites are ranked.
But until KELM is put in use there is no way to predict what kind of impact it would have.
Google doesn’t currently fact check search results.
KELM, should it be introduced, could conceivably have an impact on sites that promote factually incorrect statements and ideas.
Advertisement
Continue Reading Below
KELM Could Impact More than Search
The KELM Corpus has been released under a Creative Commons license (CC BY-SA 2.0).
That means, in theory, any other company (like Bing, Facebook or Twitter) can use it to improve their natural language processing pre-training as well.
It’s possible then that the influence of KELM could extend across many search and social media platforms.
Indirect Ties to MUM
Google has also indicated that the next-generation MUM algorithm will not be released until Google is satisfied that bias does not negatively impact the answers it gives.
According to the Google MUM announcement:
“Just as we’ve carefully tested the many applications of BERT launched since 2019, MUM will undergo the same process as we apply these models in Search.
Specifically, we’ll look for patterns that may indicate bias in machine learning to avoid introducing bias into our systems.”
The KELM approach specifically targets bias reduction, which could make it valuable for developing the MUM algorithm.
Machine Learning Can Generate Biased Results
The research paper states that the data that natural language models like BERT and GPT-3 use for training can result in “toxic content” and biases.
In computing there is an old acronym , GIGO that stands for Garbage In – Garbage Out. That means the quality of the output is determined by the quality of the input.
If what you’re training the algorithm with is high quality then the result is going to be high quality.
What the researchers are proposing is to improve the quality of the data that technologies like BERT and MUM are trained on in order to remove biases.
Knowledge Graph
The knowledge graph is a collection of facts in a structured data format. Structured data is a markup language that communicates specific information in a manner easily consumed by machines.
In this case the information is facts about people, places and things.
The Google Knowledge Graph was introduced in 2012 as a way to help Google understand the relationships between things. So when someone asks about Washington, Google could be able to discern if the person asking the question was asking about Washington the person, the state or the District of Columbia.
Advertisement
Continue Reading Below
Google’s knowledge graph was announced to be comprised of data from trusted sources of facts.
Google’s 2012 announcement characterized the knowledge graph as a first step towards building the next generation of search, which we are currently enjoying.
Knowledge Graph and Factual Accuracy
Knowledge graph data is used in this research paper for improving Google’s algorithms because the information is trustworthy and reliable.
The Google research paper proposes integrating knowledge graph information into the training process to remove the biases and increase factual accuracy.
What the Google research proposes is two-fold.
- First, they need to convert knowledge bases into natural language text.
- Secondly the resulting corpus, named Knowledge-Enhanced Language Model Pre-training (KELM), can then be integrated into the algorithm pre-training to reduce biases.
The researchers explain the problem like this:
“Large pre-trained natural language processing (NLP) models, such as BERT, RoBERTa, GPT-3, T5 and REALM, leverage natural language corpora that are derived from the Web and fine-tuned on task specific data…
However, natural language text alone represents a limited coverage of knowledge… Furthermore, existence of non-factual information and toxic content in text can eventually cause biases in the resulting models.”
Advertisement
Continue Reading Below
From Knowledge Graph Structured Data to Natural Language Text
The researchers state that a problem with integrating knowledge base information into the training is that the knowledge base data is in the form of structured data.
The solution is to convert the knowledge graph structured data to natural language text using a natural language task called, data-to-text-generation.
They explained that because data-to-text-generation is challenging they created what they called a new “pipeline” called “Text from KG Generator (TEKGEN)” to solve the problem.
Citation: Knowledge Graph Based Synthetic Corpus Generation for Knowledge-Enhanced Language Model Pre-training (PDF)
TEKGEN Natural Language Text Improved Factual Accuracy
TEKGEN is the technology the researchers created to convert structured data to natural language text. It is this end result, factual text, that can be used to create the KELM corpus which can then be used as part of machine learning pre-training to help prevent bias from making its way into algorithms.
The researchers noted that adding this additional knowledge graph information (corpora) into the training data resulted in improved factual accuracy.
Advertisement
Continue Reading Below
The TEKGEN/KELM paper states:
“We further show that verbalizing a comprehensive, encyclopedic KG like Wikidata can be used to integrate structured KGs and natural language corpora.
…our approach converts the KG into natural text, allowing it to be seamlessly integrated into existing language models. It carries the further advantages of improved factual accuracy and reduced toxicity in the resulting language model.”
The KELM article published an illustration showing how one structured data node is concatenated then converted from there to natural text (verbalized).
I broke up the illustration into two parts.
Below is an image representing a knowledge graph structured data. The data is concatenated to text.
Screenshot of First Part of TEKGEN Conversion Process
The image below represents the next step of the TEKGEN process that takes the concatenated text and converts it to a natural language text.
Advertisement
Continue Reading Below
Screenshot of Text Turned to Natural Language Text
Generating the KELM Corpus
There is another illustration that shows how the KELM natural language text that can be used for pre-training is generated.
The TEKGEN paper shows this illustration plus description:
- “In Step 1 , KG triples arealigned with Wikipedia text using distant supervision.
- In Steps 2 & 3 , T5 is fine-tuned sequentially first on this corpus, followed by a small number of steps on the WebNLG corpus,
- In Step 4 , BERT is fine-tuned to generate a semantic quality score for generated sentences w.r.t. triples.
- Steps 2 , 3 & 4 together form TEKGEN.
- To generate the KELM corpus, in Step 5 , entity subgraphs are created using the relation pair alignment counts from the training corpus generated in step 1.
The subgraph triples are then converted into natural text using TEKGEN.”
Advertisement
Continue Reading Below
KELM Works to Reduce Bias and Promote Accuracy
The KELM article published on Google’s AI blog states that KELM has real-world applications, particularly for question answering tasks which are explicitly related to information retrieval (search) and natural language processing (technologies like BERT and MUM).
Google researches many things, some of which seem to be explorations into what is possible but otherwise seem like dead-ends. Research that probably won’t make it into Google’s algorithm usually concludes with a statement that more research is needed because the technology doesn’t fulfill expectations in one way or another.
But that is not the case with the KELM and TEKGEN research. The article is in fact optimistic about real-world application of the discoveries. That tends to give it a higher probability that KELM could eventually make it into search in one form or another.
This is how the researchers concluded the article on KELM for reducing bias:
“This has real-world applications for knowledge-intensive tasks, such as question answering, where providing factual knowledge is essential. Moreover, such corpora can be applied in pre-training of large language models, and can potentially reduce toxicity and improve factuality.”
Advertisement
Continue Reading Below
Will KELM be Used in Soon?
Google’s recent announcement of the MUM algorithm requires accuracy, something the KELM corpus was created for. But the application of KELM is not limited to MUM.
The fact that reducing bias and factual accuracy are a critical concern in society today and that the researchers are optimistic about the results tends to give it a higher probability of being used in some form in the future in search.
Citations
Google AI Article on KELM
KELM: Integrating Knowledge Graphs with Language Model Pre-training Corpora
KELM Research Paper (PDF)
Knowledge Graph Based Synthetic Corpus Generation for Knowledge-Enhanced Language Model Pre-training
TEKGEN Training Corpus at GitHub
Facebook Faces Yet Another Outage: Platform Encounters Technical Issues Again
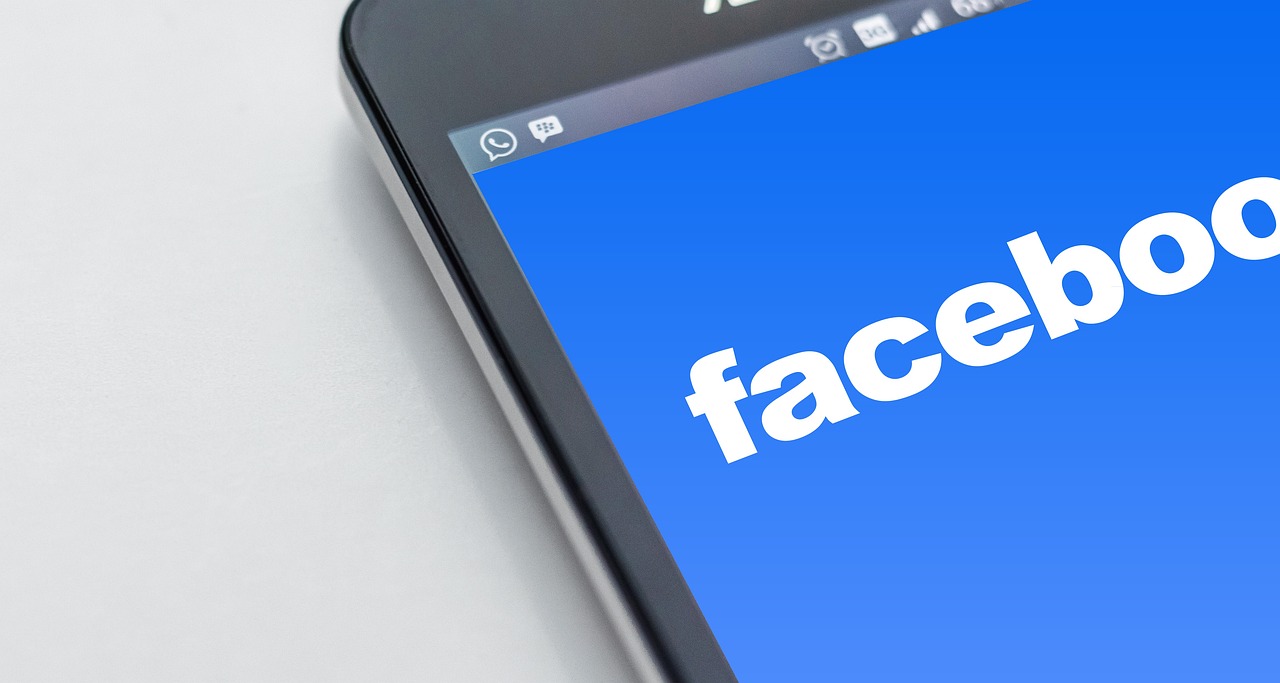
Uppdated: It seems that today’s issues with Facebook haven’t affected as many users as the last time. A smaller group of people appears to be impacted this time around, which is a relief compared to the larger incident before. Nevertheless, it’s still frustrating for those affected, and hopefully, the issues will be resolved soon by the Facebook team.
Facebook had another problem today (March 20, 2024). According to Downdetector, a website that shows when other websites are not working, many people had trouble using Facebook.
This isn’t the first time Facebook has had issues. Just a little while ago, there was another problem that stopped people from using the site. Today, when people tried to use Facebook, it didn’t work like it should. People couldn’t see their friends’ posts, and sometimes the website wouldn’t even load.
Downdetector, which watches out for problems on websites, showed that lots of people were having trouble with Facebook. People from all over the world said they couldn’t use the site, and they were not happy about it.
When websites like Facebook have problems, it affects a lot of people. It’s not just about not being able to see posts or chat with friends. It can also impact businesses that use Facebook to reach customers.
Since Facebook owns Messenger and Instagram, the problems with Facebook also meant that people had trouble using these apps. It made the situation even more frustrating for many users, who rely on these apps to stay connected with others.
During this recent problem, one thing is obvious: the internet is always changing, and even big websites like Facebook can have problems. While people wait for Facebook to fix the issue, it shows us how easily things online can go wrong. It’s a good reminder that we should have backup plans for staying connected online, just in case something like this happens again.
NEWS
We asked ChatGPT what will be Google (GOOG) stock price for 2030
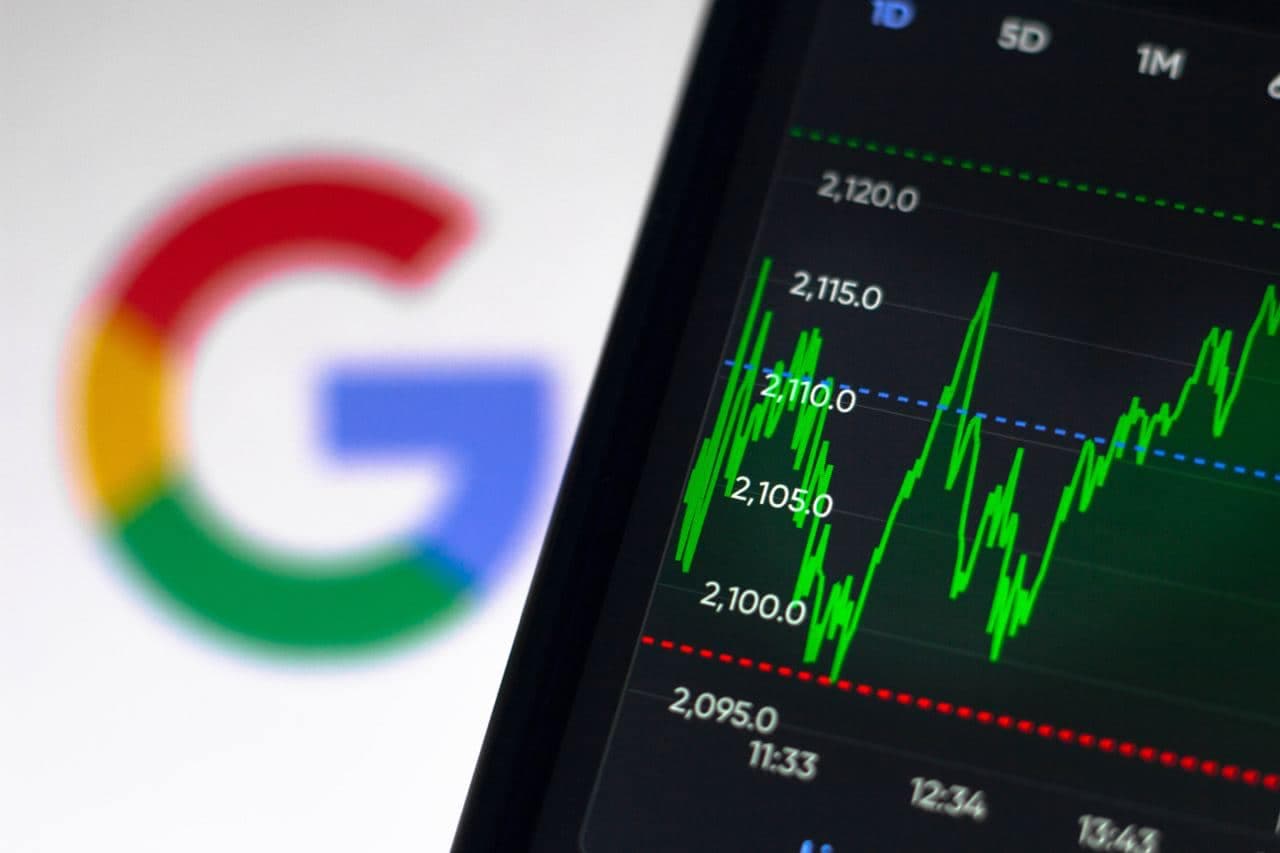
Investors who have invested in Alphabet Inc. (NASDAQ: GOOG) stock have reaped significant benefits from the company’s robust financial performance over the last five years. Google’s dominance in the online advertising market has been a key driver of the company’s consistent revenue growth and impressive profit margins.
In addition, Google has expanded its operations into related fields such as cloud computing and artificial intelligence. These areas show great promise as future growth drivers, making them increasingly attractive to investors. Notably, Alphabet’s stock price has been rising due to investor interest in the company’s recent initiatives in the fast-developing field of artificial intelligence (AI), adding generative AI features to Gmail and Google Docs.
However, when it comes to predicting the future pricing of a corporation like Google, there are many factors to consider. With this in mind, Finbold turned to the artificial intelligence tool ChatGPT to suggest a likely pricing range for GOOG stock by 2030. Although the tool was unable to give a definitive price range, it did note the following:
“Over the long term, Google has a track record of strong financial performance and has shown an ability to adapt to changing market conditions. As such, it’s reasonable to expect that Google’s stock price may continue to appreciate over time.”
GOOG stock price prediction
While attempting to estimate the price range of future transactions, it is essential to consider a variety of measures in addition to the AI chat tool, which includes deep learning algorithms and stock market experts.
Finbold collected forecasts provided by CoinPriceForecast, a finance prediction tool that utilizes machine self-learning technology, to anticipate Google stock price by the end of 2030 to compare with ChatGPT’s projection.
According to the most recent long-term estimate, which Finbold obtained on March 20, the price of Google will rise beyond $200 in 2030 and touch $247 by the end of the year, which would indicate a 141% gain from today to the end of the year.
Google has been assigned a recommendation of ‘strong buy’ by the majority of analysts working on Wall Street for a more near-term time frame. Significantly, 36 analysts of the 48 have recommended a “strong buy,” while seven people have advocated a “buy.” The remaining five analysts had given a ‘hold’ rating.

The average price projection for Alphabet stock over the last three months has been $125.32; this objective represents a 22.31% upside from its current price. It’s interesting to note that the maximum price forecast for the next year is $160, representing a gain of 56.16% from the stock’s current price of $102.46.
While the outlook for Google stock may be positive, it’s important to keep in mind that some potential challenges and risks could impact its performance, including competition from ChatGPT itself, which could affect Google’s price.
Disclaimer: The content on this site should not be considered investment advice. Investing is speculative. When investing, your capital is at risk.
NEWS
This Apple Watch app brings ChatGPT to your wrist — here’s why you want it
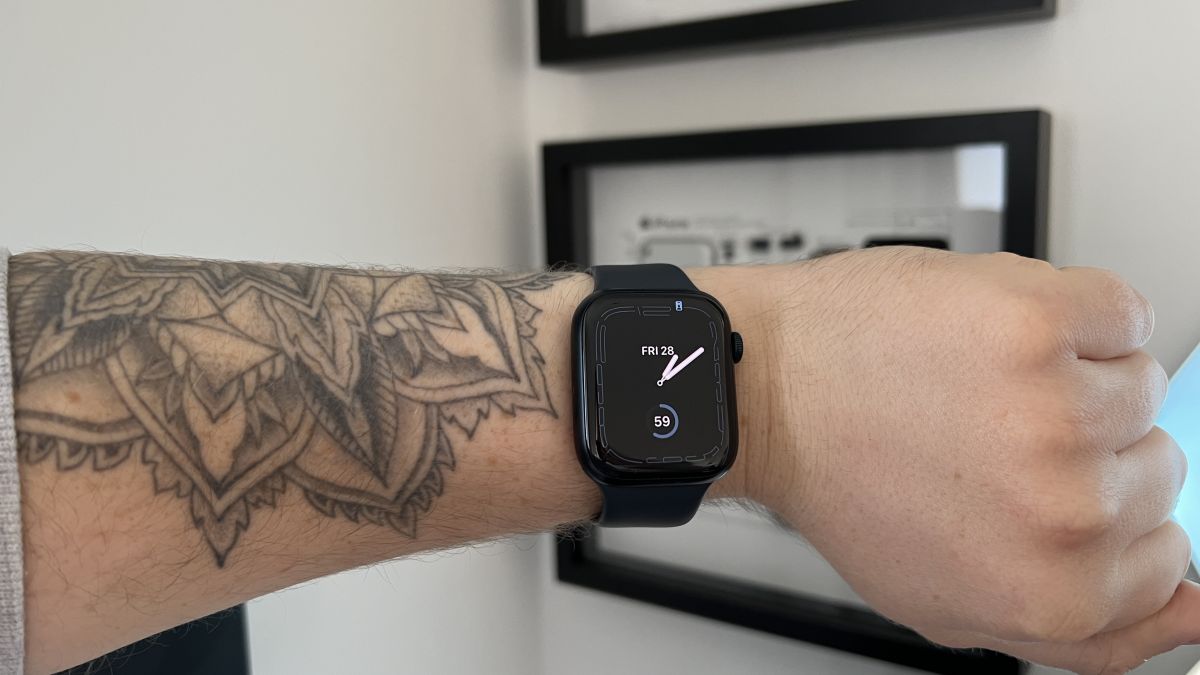
ChatGPT feels like it is everywhere at the moment; the AI-powered tool is rapidly starting to feel like internet connected home devices where you are left wondering if your flower pot really needed Bluetooth. However, after hearing about a new Apple Watch app that brings ChatGPT to your favorite wrist computer, I’m actually convinced this one is worth checking out.
-
MARKETING7 days ago
The key to correcting the C-suite trust deficit
-
MARKETING6 days ago
A Recap of Everything Marketers & Advertisers Need to Know
-
PPC4 days ago
How the TikTok Algorithm Works in 2024 (+9 Ways to Go Viral)
-
MARKETING4 days ago
How To Protect Your People and Brand
-
SEARCHENGINES5 days ago
Google Started Enforcing The Site Reputation Abuse Policy
-
SEO7 days ago
128 Top SEO Tools That Are 100% Free
-
PPC5 days ago
How to Craft Compelling Google Ads for eCommerce
-
SEO5 days ago
Blog Post Checklist: Check All Prior to Hitting “Publish”