SEO
Using Python + Streamlit To Find Striking Distance Keyword Opportunities
Python is an excellent tool to automate repetitive tasks as well as gain additional insights into data.
In this article, you’ll learn how to build a tool to check which keywords are close to ranking in positions one to three and advises whether there is an opportunity to naturally work those keywords into the page.
It’s perfect for Python beginners and pros alike and is a great introduction to using Python for SEO.
If you’d just like to get stuck in there’s a handy Streamlit app available for the code. This is simple to use and requires no coding experience.
There’s also a Google Colaboratory Sheet if you’d like to poke around with the code. If you can crawl a website, you can use this script!
Here’s an example of what we’ll be making today:

These keywords are found in the page title and H1, but not in the copy. Adding these keywords naturally to the existing copy would be an easy way to increase relevancy for these keywords.
By taking the hint from search engines and naturally including any missing keywords a site already ranks for, we increase the confidence of search engines to rank those keywords higher in the SERPs.
This report can be created manually, but it’s pretty time-consuming.
So, we’re going to automate the process using a Python SEO script.
Preview Of The Output
This is a sample of what the final output will look like after running the report:


The final output takes the top five opportunities by search volume for each page and neatly lays each one horizontally along with the estimated search volume.
It also shows the total search volume of all keywords a page has within striking distance, as well as the total number of keywords within reach.
The top five keywords by search volume are then checked to see if they are found in the title, H1, or copy, then flagged TRUE or FALSE.
This is great for finding quick wins! Just add the missing keyword naturally into the page copy, title, or H1.
Getting Started
The setup is fairly straightforward. We just need a crawl of the site (ideally with a custom extraction for the copy you’d like to check), and an exported file of all keywords a site ranks for.
This post will walk you through the setup, the code, and will link to a Google Colaboratory sheet if you just want to get stuck in without coding it yourself.
To get started you will need:
We’ve named this the Striking Distance Report as it flags keywords that are easily within striking distance.
(We have defined striking distance as keywords that rank in positions four to 20, but have made this a configurable option in case you would like to define your own parameters.)
Striking Distance SEO Report: Getting Started
1. Crawl The Target Website
- Set a custom extractor for the page copy (optional, but recommended).
- Filter out pagination pages from the crawl.
2. Export All Keywords The Site Ranks For Using Your Favorite Provider
- Filter keywords that trigger as a site link.
- Remove keywords that trigger as an image.
- Filter branded keywords.
- Use both exports to create an actionable Striking Distance report from the keyword and crawl data with Python.
Crawling The Site
I’ve opted to use Screaming Frog to get the initial crawl. Any crawler will work, so long as the CSV export uses the same column names or they’re renamed to match.
The script expects to find the following columns in the crawl CSV export:
"Address", "Title 1", "H1-1", "Copy 1", "Indexability"
Crawl Settings
The first thing to do is to head over to the main configuration settings within Screaming Frog:
Configuration > Spider > Crawl
The main settings to use are:
Crawl Internal Links, Canonicals, and the Pagination (Rel Next/Prev) setting.
(The script will work with everything else selected, but the crawl will take longer to complete!)


Next, it’s on to the Extraction tab.
Configuration > Spider > Extraction


At a bare minimum, we need to extract the page title, H1, and calculate whether the page is indexable as shown below.
Indexability is useful because it’s an easy way for the script to identify which URLs to drop in one go, leaving only keywords that are eligible to rank in the SERPs.
If the script cannot find the indexability column, it’ll still work as normal but won’t differentiate between pages that can and cannot rank.
Setting A Custom Extractor For Page Copy
In order to check whether a keyword is found within the page copy, we need to set a custom extractor in Screaming Frog.
Configuration > Custom > Extraction
Name the extractor “Copy” as seen below.


Important: The script expects the extractor to be named “Copy” as above, so please double check!
Lastly, make sure Extract Text is selected to export the copy as text, rather than HTML.
There are many guides on using custom extractors online if you need help setting one up, so I won’t go over it again here.
Once the extraction has been set it’s time to crawl the site and export the HTML file in CSV format.
Exporting The CSV File
Exporting the CSV file is as easy as changing the drop-down menu displayed underneath Internal to HTML and pressing the Export button.
Internal > HTML > Export


After clicking Export, It’s important to make sure the type is set to CSV format.
The export screen should look like the below:


Tip 1: Filtering Out Pagination Pages
I recommend filtering out pagination pages from your crawl either by selecting Respect Next/Prev under the Advanced settings (or just deleting them from the CSV file, if you prefer).


Tip 2: Saving The Crawl Settings
Once you have set the crawl up, it’s worth just saving the crawl settings (which will also remember the custom extraction).
This will save a lot of time if you want to use the script again in the future.
File > Configuration > Save As


Exporting Keywords
Once we have the crawl file, the next step is to load your favorite keyword research tool and export all of the keywords a site ranks for.
The goal here is to export all the keywords a site ranks for, filtering out branded keywords and any which triggered as a sitelink or image.
For this example, I’m using the Organic Keyword Report in Ahrefs, but it will work just as well with Semrush if that’s your preferred tool.
In Ahrefs, enter the domain you’d like to check in Site Explorer and choose Organic Keywords.


Site Explorer > Organic Keywords


This will bring up all keywords the site is ranking for.
Filtering Out Sitelinks And Image links
The next step is to filter out any keywords triggered as a sitelink or an image pack.
The reason we need to filter out sitelinks is that they have no influence on the parent URL ranking. This is because only the parent page technically ranks for the keyword, not the sitelink URLs displayed under it.
Filtering out sitelinks will ensure that we are optimizing the correct page.


Here’s how to do it in Ahrefs.


Lastly, I recommend filtering out any branded keywords. You can do this by filtering the CSV output directly, or by pre-filtering in the keyword tool of your choice before the export.
Finally, when exporting make sure to choose Full Export and the UTF-8 format as shown below.


By default, the script works with Ahrefs (v1/v2) and Semrush keyword exports. It can work with any keyword CSV file as long as the column names the script expects are present.
Processing
The following instructions pertain to running a Google Colaboratory sheet to execute the code.
There is now a simpler option for those that prefer it in the form of a Streamlit app. Simply follow the instructions provided to upload your crawl and keyword file.
Now that we have our exported files, all that’s left to be done is to upload them to the Google Colaboratory sheet for processing.
Select Runtime > Run all from the top navigation to run all cells in the sheet.


The script will prompt you to upload the keyword CSV from Ahrefs or Semrush first and the crawl file afterward.


That’s it! The script will automatically download an actionable CSV file you can use to optimize your site.


Once you’re familiar with the whole process, using the script is really straightforward.
Code Breakdown And Explanation
If you’re learning Python for SEO and interested in what the code is doing to produce the report, stick around for the code walkthrough!
Install The Libraries
Let’s install pandas to get the ball rolling.
!pip install pandas
Import The Modules
Next, we need to import the required modules.
import pandas as pd from pandas import DataFrame, Series from typing import Union from google.colab import files
Set The Variables
Now it’s time to set the variables.
The script considers any keywords between positions four and 20 as within striking distance.
Changing the variables here will let you define your own range if desired. It’s worth experimenting with the settings to get the best possible output for your needs.
# set all variables here min_volume = 10 # set the minimum search volume min_position = 4 # set the minimum position / default = 4 max_position = 20 # set the maximum position / default = 20 drop_all_true = True # If all checks (h1/title/copy) are true, remove the recommendation (Nothing to do) pagination_filters = "filterby|page|p=" # filter patterns used to detect and drop paginated pages
Upload The Keyword Export CSV File
The next step is to read in the list of keywords from the CSV file.
It is set up to accept an Ahrefs report (V1 and V2) as well as a Semrush export.
This code reads in the CSV file into a Pandas DataFrame.
upload = files.upload() upload = list(upload.keys())[0] df_keywords = pd.read_csv( (upload), error_bad_lines=False, low_memory=False, encoding="utf8", dtype={ "URL": "str", "Keyword": "str", "Volume": "str", "Position": int, "Current URL": "str", "Search Volume": int, }, ) print("Uploaded Keyword CSV File Successfully!")
If everything went to plan, you’ll see a preview of the DataFrame created from the keyword CSV export.


Upload The Crawl Export CSV File
Once the keywords have been imported, it’s time to upload the crawl file.
This fairly simple piece of code reads in the crawl with some error handling option and creates a Pandas DataFrame named df_crawl. upload = files.upload() upload = list(upload.keys())[0] df_crawl = pd.read_csv( (upload), error_bad_lines=False, low_memory=False, encoding="utf8", dtype="str", ) print("Uploaded Crawl Dataframe Successfully!")
Once the CSV file has finished uploading, you’ll see a preview of the DataFrame.


Clean And Standardize The Keyword Data
The next step is to rename the column names to ensure standardization between the most common types of file exports.
Essentially, we’re getting the keyword DataFrame into a good state and filtering using cutoffs defined by the variables.
df_keywords.rename( columns={ "Current position": "Position", "Current URL": "URL", "Search Volume": "Volume", }, inplace=True, ) # keep only the following columns from the keyword dataframe cols = "URL", "Keyword", "Volume", "Position" df_keywords = df_keywords.reindex(columns=cols) try: # clean the data. (v1 of the ahrefs keyword export combines strings and ints in the volume column) df_keywords["Volume"] = df_keywords["Volume"].str.replace("0-10", "0") except AttributeError: pass # clean the keyword data df_keywords = df_keywords[df_keywords["URL"].notna()] # remove any missing values df_keywords = df_keywords[df_keywords["Volume"].notna()] # remove any missing values df_keywords = df_keywords.astype({"Volume": int}) # change data type to int df_keywords = df_keywords.sort_values(by="Volume", ascending=False) # sort by highest vol to keep the top opportunity # make new dataframe to merge search volume back in later df_keyword_vol = df_keywords[["Keyword", "Volume"]] # drop rows if minimum search volume doesn't match specified criteria df_keywords.loc[df_keywords["Volume"] < min_volume, "Volume_Too_Low"] = "drop" df_keywords = df_keywords[~df_keywords["Volume_Too_Low"].isin(["drop"])] # drop rows if minimum search position doesn't match specified criteria df_keywords.loc[df_keywords["Position"] <= min_position, "Position_Too_High"] = "drop" df_keywords = df_keywords[~df_keywords["Position_Too_High"].isin(["drop"])] # drop rows if maximum search position doesn't match specified criteria df_keywords.loc[df_keywords["Position"] >= max_position, "Position_Too_Low"] = "drop" df_keywords = df_keywords[~df_keywords["Position_Too_Low"].isin(["drop"])]
Clean And Standardize The Crawl Data
Next, we need to clean and standardize the crawl data.
Essentially, we use reindex to only keep the “Address,” “Indexability,” “Page Title,” “H1-1,” and “Copy 1” columns, discarding the rest.
We use the handy “Indexability” column to only keep rows that are indexable. This will drop canonicalized URLs, redirects, and so on. I recommend enabling this option in the crawl.
Lastly, we standardize the column names so they’re a little nicer to work with.
# keep only the following columns from the crawl dataframe cols = "Address", "Indexability", "Title 1", "H1-1", "Copy 1" df_crawl = df_crawl.reindex(columns=cols) # drop non-indexable rows df_crawl = df_crawl[~df_crawl["Indexability"].isin(["Non-Indexable"])] # standardise the column names df_crawl.rename(columns={"Address": "URL", "Title 1": "Title", "H1-1": "H1", "Copy 1": "Copy"}, inplace=True) df_crawl.head()
Group The Keywords
As we approach the final output, it’s necessary to group our keywords together to calculate the total opportunity for each page.
Here, we’re calculating how many keywords are within striking distance for each page, along with the combined search volume.
# groups the URLs (remove the dupes and combines stats) # make a copy of the keywords dataframe for grouping - this ensures stats can be merged back in later from the OG df df_keywords_group = df_keywords.copy() df_keywords_group["KWs in Striking Dist."] = 1 # used to count the number of keywords in striking distance df_keywords_group = ( df_keywords_group.groupby("URL") .agg({"Volume": "sum", "KWs in Striking Dist.": "count"}) .reset_index() ) df_keywords_group.head()


Once complete, you’ll see a preview of the DataFrame.
Display Keywords In Adjacent Rows
We use the grouped data as the basis for the final output. We use Pandas.unstack to reshape the DataFrame to display the keywords in the style of a GrepWords export.


# create a new df, combine the merged data with the original data. display in adjacent rows ala grepwords df_merged_all_kws = df_keywords_group.merge( df_keywords.groupby("URL")["Keyword"] .apply(lambda x: x.reset_index(drop=True)) .unstack() .reset_index() ) # sort by biggest opportunity df_merged_all_kws = df_merged_all_kws.sort_values( by="KWs in Striking Dist.", ascending=False ) # reindex the columns to keep just the top five keywords cols = "URL", "Volume", "KWs in Striking Dist.", 0, 1, 2, 3, 4 df_merged_all_kws = df_merged_all_kws.reindex(columns=cols) # create union and rename the columns df_striking: Union[Series, DataFrame, None] = df_merged_all_kws.rename( columns={ "Volume": "Striking Dist. Vol", 0: "KW1", 1: "KW2", 2: "KW3", 3: "KW4", 4: "KW5", } ) # merges striking distance df with crawl df to merge in the title, h1 and category description df_striking = pd.merge(df_striking, df_crawl, on="URL", how="inner")
Set The Final Column Order And Insert Placeholder Columns
Lastly, we set the final column order and merge in the original keyword data.
There are a lot of columns to sort and create!
# set the final column order and merge the keyword data in cols = [ "URL", "Title", "H1", "Copy", "Striking Dist. Vol", "KWs in Striking Dist.", "KW1", "KW1 Vol", "KW1 in Title", "KW1 in H1", "KW1 in Copy", "KW2", "KW2 Vol", "KW2 in Title", "KW2 in H1", "KW2 in Copy", "KW3", "KW3 Vol", "KW3 in Title", "KW3 in H1", "KW3 in Copy", "KW4", "KW4 Vol", "KW4 in Title", "KW4 in H1", "KW4 in Copy", "KW5", "KW5 Vol", "KW5 in Title", "KW5 in H1", "KW5 in Copy", ] # re-index the columns to place them in a logical order + inserts new blank columns for kw checks. df_striking = df_striking.reindex(columns=cols)
Merge In The Keyword Data For Each Column
This code merges the keyword volume data back into the DataFrame. It’s more or less the equivalent of an Excel VLOOKUP function.
# merge in keyword data for each keyword column (KW1 - KW5) df_striking = pd.merge(df_striking, df_keyword_vol, left_on="KW1", right_on="Keyword", how="left") df_striking['KW1 Vol'] = df_striking['Volume'] df_striking.drop(['Keyword', 'Volume'], axis=1, inplace=True) df_striking = pd.merge(df_striking, df_keyword_vol, left_on="KW2", right_on="Keyword", how="left") df_striking['KW2 Vol'] = df_striking['Volume'] df_striking.drop(['Keyword', 'Volume'], axis=1, inplace=True) df_striking = pd.merge(df_striking, df_keyword_vol, left_on="KW3", right_on="Keyword", how="left") df_striking['KW3 Vol'] = df_striking['Volume'] df_striking.drop(['Keyword', 'Volume'], axis=1, inplace=True) df_striking = pd.merge(df_striking, df_keyword_vol, left_on="KW4", right_on="Keyword", how="left") df_striking['KW4 Vol'] = df_striking['Volume'] df_striking.drop(['Keyword', 'Volume'], axis=1, inplace=True) df_striking = pd.merge(df_striking, df_keyword_vol, left_on="KW5", right_on="Keyword", how="left") df_striking['KW5 Vol'] = df_striking['Volume'] df_striking.drop(['Keyword', 'Volume'], axis=1, inplace=True)
Clean The Data Some More
The data requires additional cleaning to populate empty values, (NaNs), as empty strings. This improves the readability of the final output by creating blank cells, instead of cells populated with NaN string values.
Next, we convert the columns to lowercase so that they match when checking whether a target keyword is featured in a specific column.
# replace nan values with empty strings df_striking = df_striking.fillna("") # drop the title, h1 and category description to lower case so kws can be matched to them df_striking["Title"] = df_striking["Title"].str.lower() df_striking["H1"] = df_striking["H1"].str.lower() df_striking["Copy"] = df_striking["Copy"].str.lower()
Check Whether The Keyword Appears In The Title/H1/Copy and Return True Or False
This code checks if the target keyword is found in the page title/H1 or copy.
It’ll flag true or false depending on whether a keyword was found within the on-page elements.
df_striking["KW1 in Title"] = df_striking.apply(lambda row: row["KW1"] in row["Title"], axis=1) df_striking["KW1 in H1"] = df_striking.apply(lambda row: row["KW1"] in row["H1"], axis=1) df_striking["KW1 in Copy"] = df_striking.apply(lambda row: row["KW1"] in row["Copy"], axis=1) df_striking["KW2 in Title"] = df_striking.apply(lambda row: row["KW2"] in row["Title"], axis=1) df_striking["KW2 in H1"] = df_striking.apply(lambda row: row["KW2"] in row["H1"], axis=1) df_striking["KW2 in Copy"] = df_striking.apply(lambda row: row["KW2"] in row["Copy"], axis=1) df_striking["KW3 in Title"] = df_striking.apply(lambda row: row["KW3"] in row["Title"], axis=1) df_striking["KW3 in H1"] = df_striking.apply(lambda row: row["KW3"] in row["H1"], axis=1) df_striking["KW3 in Copy"] = df_striking.apply(lambda row: row["KW3"] in row["Copy"], axis=1) df_striking["KW4 in Title"] = df_striking.apply(lambda row: row["KW4"] in row["Title"], axis=1) df_striking["KW4 in H1"] = df_striking.apply(lambda row: row["KW4"] in row["H1"], axis=1) df_striking["KW4 in Copy"] = df_striking.apply(lambda row: row["KW4"] in row["Copy"], axis=1) df_striking["KW5 in Title"] = df_striking.apply(lambda row: row["KW5"] in row["Title"], axis=1) df_striking["KW5 in H1"] = df_striking.apply(lambda row: row["KW5"] in row["H1"], axis=1) df_striking["KW5 in Copy"] = df_striking.apply(lambda row: row["KW5"] in row["Copy"], axis=1)
Delete True/False Values If There Is No Keyword
This will delete true/false values when there is no keyword adjacent.
# delete true / false values if there is no keyword df_striking.loc[df_striking["KW1"] == "", ["KW1 in Title", "KW1 in H1", "KW1 in Copy"]] = "" df_striking.loc[df_striking["KW2"] == "", ["KW2 in Title", "KW2 in H1", "KW2 in Copy"]] = "" df_striking.loc[df_striking["KW3"] == "", ["KW3 in Title", "KW3 in H1", "KW3 in Copy"]] = "" df_striking.loc[df_striking["KW4"] == "", ["KW4 in Title", "KW4 in H1", "KW4 in Copy"]] = "" df_striking.loc[df_striking["KW5"] == "", ["KW5 in Title", "KW5 in H1", "KW5 in Copy"]] = "" df_striking.head()
Drop Rows If All Values == True
This configurable option is really useful for reducing the amount of QA time required for the final output by dropping the keyword opportunity from the final output if it is found in all three columns.
def true_dropper(col1, col2, col3): drop = df_striking.drop( df_striking[ (df_striking[col1] == True) & (df_striking[col2] == True) & (df_striking[col3] == True) ].index ) return drop if drop_all_true == True: df_striking = true_dropper("KW1 in Title", "KW1 in H1", "KW1 in Copy") df_striking = true_dropper("KW2 in Title", "KW2 in H1", "KW2 in Copy") df_striking = true_dropper("KW3 in Title", "KW3 in H1", "KW3 in Copy") df_striking = true_dropper("KW4 in Title", "KW4 in H1", "KW4 in Copy") df_striking = true_dropper("KW5 in Title", "KW5 in H1", "KW5 in Copy")
Download The CSV File
The last step is to download the CSV file and start the optimization process.
df_striking.to_csv('Keywords in Striking Distance.csv', index=False) files.download("Keywords in Striking Distance.csv")
Conclusion
If you are looking for quick wins for any website, the striking distance report is a really easy way to find them.
Don’t let the number of steps fool you. It’s not as complex as it seems. It’s as simple as uploading a crawl and keyword export to the supplied Google Colab sheet or using the Streamlit app.
The results are definitely worth it!
More Resources:
Featured Image: aurielaki/Shutterstock
!function(f,b,e,v,n,t,s)
{if(f.fbq)return;n=f.fbq=function(){n.callMethod?
n.callMethod.apply(n,arguments):n.queue.push(arguments)};
if(!f._fbq)f._fbq=n;n.push=n;n.loaded=!0;n.version=’2.0′;
n.queue=[];t=b.createElement(e);t.async=!0;
t.src=v;s=b.getElementsByTagName(e)[0];
s.parentNode.insertBefore(t,s)}(window,document,’script’,
‘https://connect.facebook.net/en_US/fbevents.js’);
if( typeof sopp !== “undefined” && sopp === ‘yes’ ){
fbq(‘dataProcessingOptions’, [‘LDU’], 1, 1000);
}else{
fbq(‘dataProcessingOptions’, []);
}
fbq(‘init’, ‘1321385257908563’);
fbq(‘track’, ‘PageView’);
fbq(‘trackSingle’, ‘1321385257908563’, ‘ViewContent’, {
content_name: ‘striking-distance-keywords-python’,
content_category: ‘seo-strategy technical-seo ‘
});
SEO
Why Google Can’t Tell You About Every Ranking Drop
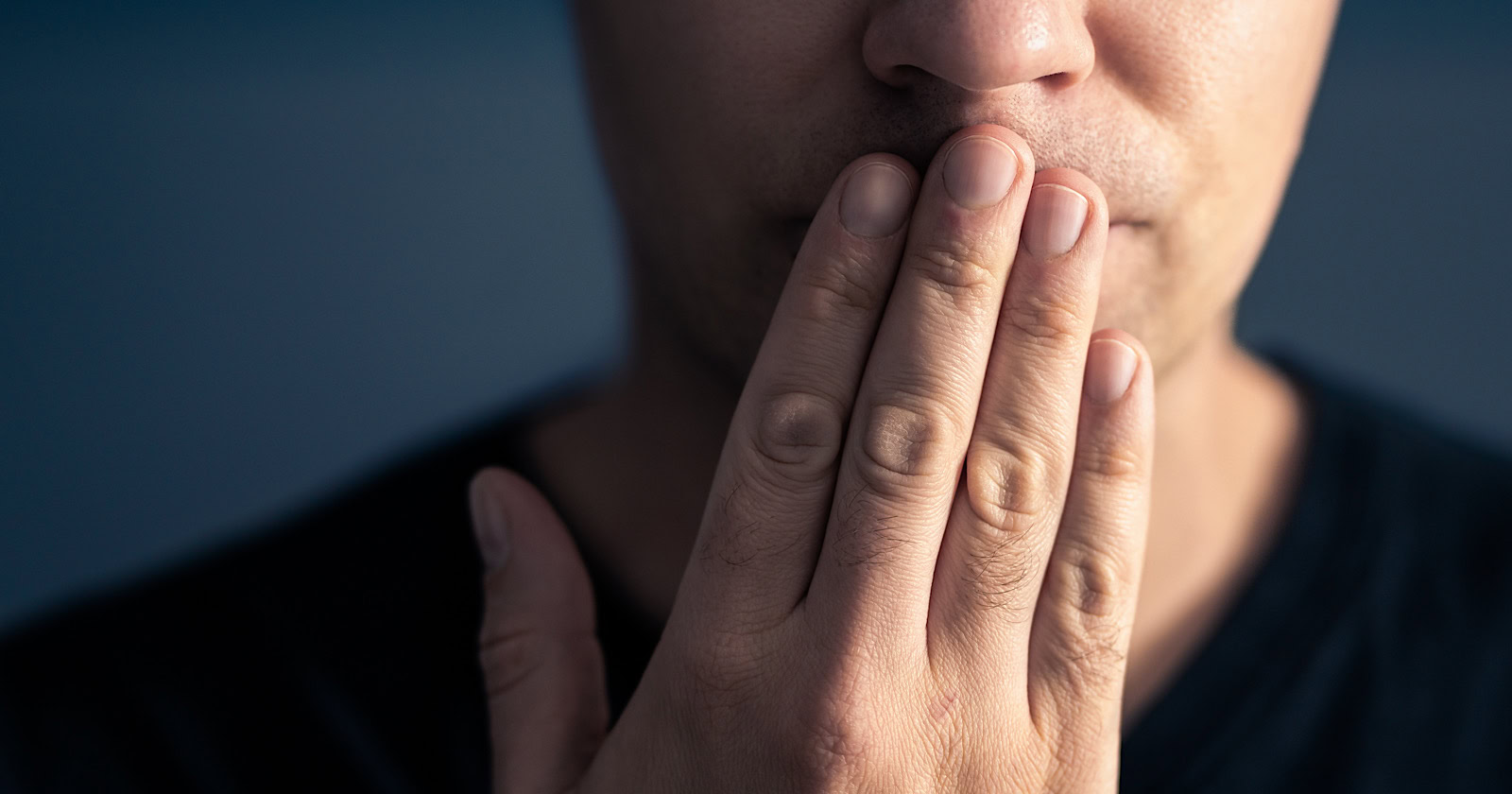
In a recent Twitter exchange, Google’s Search Liaison, Danny Sullivan, provided insight into how the search engine handles algorithmic spam actions and ranking drops.
The discussion was sparked by a website owner’s complaint about a significant traffic loss and the inability to request a manual review.
Sullivan clarified that a site could be affected by an algorithmic spam action or simply not ranking well due to other factors.
He emphasized that many sites experiencing ranking drops mistakenly attribute it to an algorithmic spam action when that may not be the case.
“I’ve looked at many sites where people have complained about losing rankings and decide they have a algorithmic spam action against them, but they don’t. “
Sullivan’s full statement will help you understand Google’s transparency challenges.
Additionally, he explains why the desire for manual review to override automated rankings may be misguided.
Two different things. A site could have an algorithmic spam action. A site could be not ranking well because other systems that *are not about spam* just don’t see it as helpful.
I’ve looked at many sites where people have complained about losing rankings and decide they have a…
— Google SearchLiaison (@searchliaison) May 13, 2024
Challenges In Transparency & Manual Intervention
Sullivan acknowledged the idea of providing more transparency in Search Console, potentially notifying site owners of algorithmic actions similar to manual actions.
However, he highlighted two key challenges:
- Revealing algorithmic spam indicators could allow bad actors to game the system.
- Algorithmic actions are not site-specific and cannot be manually lifted.
Sullivan expressed sympathy for the frustration of not knowing the cause of a traffic drop and the inability to communicate with someone about it.
However, he cautioned against the desire for a manual intervention to override the automated systems’ rankings.
Sullivan states:
“…you don’t really want to think “Oh, I just wish I had a manual action, that would be so much easier.” You really don’t want your individual site coming the attention of our spam analysts. First, it’s not like manual actions are somehow instantly processed. Second, it’s just something we know about a site going forward, especially if it says it has change but hasn’t really.”
Determining Content Helpfulness & Reliability
Moving beyond spam, Sullivan discussed various systems that assess the helpfulness, usefulness, and reliability of individual content and sites.
He acknowledged that these systems are imperfect and some high-quality sites may not be recognized as well as they should be.
“Some of them ranking really well. But they’ve moved down a bit in small positions enough that the traffic drop is notable. They assume they have fundamental issues but don’t, really — which is why we added a whole section about this to our debugging traffic drops page.”
Sullivan revealed ongoing discussions about providing more indicators in Search Console to help creators understand their content’s performance.
“Another thing I’ve been discussing, and I’m not alone in this, is could we do more in Search Console to show some of these indicators. This is all challenging similar to all the stuff I said about spam, about how not wanting to let the systems get gamed, and also how there’s then no button we would push that’s like “actually more useful than our automated systems think — rank it better!” But maybe there’s a way we can find to share more, in a way that helps everyone and coupled with better guidance, would help creators.”
Advocacy For Small Publishers & Positive Progress
In response to a suggestion from Brandon Saltalamacchia, founder of RetroDodo, about manually reviewing “good” sites and providing guidance, Sullivan shared his thoughts on potential solutions.
He mentioned exploring ideas such as self-declaration through structured data for small publishers and learning from that information to make positive changes.
“I have some thoughts I’ve been exploring and proposing on what we might do with small publishers and self-declaring with structured data and how we might learn from that and use that in various ways. Which is getting way ahead of myself and the usual no promises but yes, I think and hope for ways to move ahead more positively.”
Sullivan said he can’t make promises or implement changes overnight, but he expressed hope for finding ways to move forward positively.
Featured Image: Tero Vesalainen/Shutterstock
SEO
56 Google Search Statistics to Bookmark for 2024
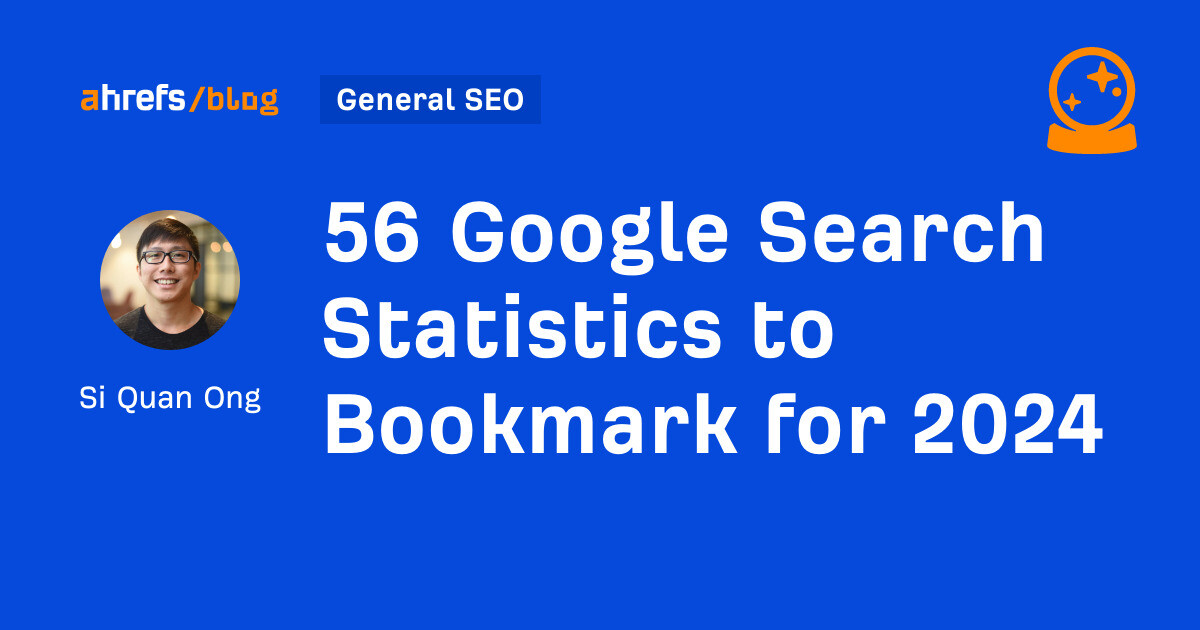
If you’re curious about the state of Google search in 2024, look no further.
Each year we pick, vet, and categorize a list of up-to-date statistics to give you insights from trusted sources on Google search trends.
- Google has a web index of “about 400 billion documents”. (The Capitol Forum)
- Google’s search index is over 100 million gigabytes in size. (Google)
- There are an estimated 3.5 billion searches on Google each day. (Internet Live Stats)
- 61.5% of desktop searches and 34.4% of mobile searches result in no clicks. (SparkToro)
- 15% of all Google searches have never been searched before. (Google)
- 94.74% of keywords get 10 monthly searches or fewer. (Ahrefs)
- The most searched keyword in the US and globally is “YouTube,” and youtube.com gets the most traffic from Google. (Ahrefs)
- 96.55% of all pages get zero search traffic from Google. (Ahrefs)
- 50-65% of all number-one spots are dominated by featured snippets. (Authority Hacker)
- Reddit is the most popular domain for product review queries. (Detailed)
- Google is the most used search engine in the world, with a mobile market share of 95.32% and a desktop market share of 81.95%. (Statista)
- Google.com generated 84.2 billion visits a month in 2023. (Statista)
- Google generated $307.4 billion in revenue in 2023. (Alphabet Investor Relations)
- 63.41% of all US web traffic referrals come from Google. (SparkToro)
- 92.96% of global traffic comes from Google Search, Google Images, and Google Maps. (SparkToro)
- Only 49% of Gen Z women use Google as their search engine. The rest use TikTok. (Search Engine Land)
- 58.67% of all website traffic worldwide comes from mobile phones. (Statista)
- 57% of local search queries are submitted using a mobile device or tablet. (ReviewTrackers)
- 51% of smartphone users have discovered a new company or product when conducting a search on their smartphones. (Think With Google)
- 54% of smartphone users search for business hours, and 53% search for directions to local stores. (Think With Google)
- 18% of local searches on smartphones lead to a purchase within a day vs. 7% of non-local searches. (Think With Google)
- 56% of in-store shoppers used their smartphones to shop or research items while they were in-store. (Think With Google)
- 60% of smartphone users have contacted a business directly using the search results (e.g., “click to call” option). (Think With Google)
- 63.6% of consumers say they are likely to check reviews on Google before visiting a business location. (ReviewTrackers)
- 88% of consumers would use a business that replies to all of its reviews. (BrightLocal)
- Customers are 2.7 times more likely to consider a business reputable if they find a complete Business Profile on Google Search and Maps. (Google)
- Customers are 70% more likely to visit and 50% more likely to consider purchasing from businesses with a complete Business Profile. (Google)
- 76% of people who search on their smartphones for something nearby visit a business within a day. (Think With Google)
- 28% of searches for something nearby result in a purchase. (Think With Google)
- Mobile searches for “store open near me” (such as, “grocery store open near me” have grown by over 250% in the last two years. (Think With Google)
- People use Google Lens for 12 billion visual searches a month. (Google)
- 50% of online shoppers say images helped them decide what to buy. (Think With Google)
- There are an estimated 136 billion indexed images on Google Image Search. (Photutorial)
- 15.8% of Google SERPs show images. (Moz)
- People click on 3D images almost 50% more than static ones. (Google)
- More than 800 million people use Google Discover monthly to stay updated on their interests. (Google)
- 46% of Google Discover URLs are news sites, 44% e-commerce, 7% entertainment, and 2% travel. (Search Engine Journal)
- Even though news sites accounted for under 50% of Google Discover URLs, they received 99% of Discover clicks. (Search Engine Journal)
- Most Google Discover URLs only receive traffic for three to four days, with most of that traffic occurring one to two days after publishing. (Search Engine Journal)
- The clickthrough rate (CTR) for Google Discover is 11%. (Search Engine Journal)
- 91.45% of search volumes in Google Ads Keyword Planner are overestimates. (Ahrefs)
- For every $1 a business spends on Google Ads, they receive $8 in profit through Google Search and Ads. (Google)
- Google removed 5.5 billion ads, suspended 12.7 million advertiser accounts, restricted over 6.9 billion ads, and restricted ads from showing up on 2.1 billion publisher pages in 2023. (Google)
- The average shopping click-through rate (CTR) across all industries is 0.86% for Google Ads. (Wordstream)
- The average shopping cost per click (CPC) across all industries is $0.66 for Google Ads. (Wordstream)
- The average shopping conversion rate (CVR) across all industries is 1.91% for Google Ads. (Wordstream)
- 58% of consumers ages 25-34 use voice search daily. (UpCity)
- 16% of people use voice search for local “near me” searches. (UpCity)
- 67% of consumers say they’re very likely to use voice search when seeking information. (UpCity)
- Active users of the Google Assistant grew 4X over the past year, as of 2019. (Think With Google)
- Google Assistant hit 1 billion app installs. (Android Police)
- AI-generated answers from SGE were available for 91% of entertainment queries but only 17% of healthcare queries. (Statista)
- The AI-generated answers in Google’s Search Generative Experience (SGE) do not match any links from the top 10 Google organic search results 93.8% of the time. (Search Engine Journal)
- Google displays a Search Generative element for 86.8% of all search queries. (Authoritas)
- 62% of generative links came from sources outside the top 10 ranking organic domains. Only 20.1% of generative URLs directly match an organic URL ranking on page one. (Authoritas)
- 70% of SEOs said that they were worried about the impact of SGE on organic search (Aira)
Learn more
Check out more resources on how Google works:
SEO
How To Use ChatGPT For Keyword Research
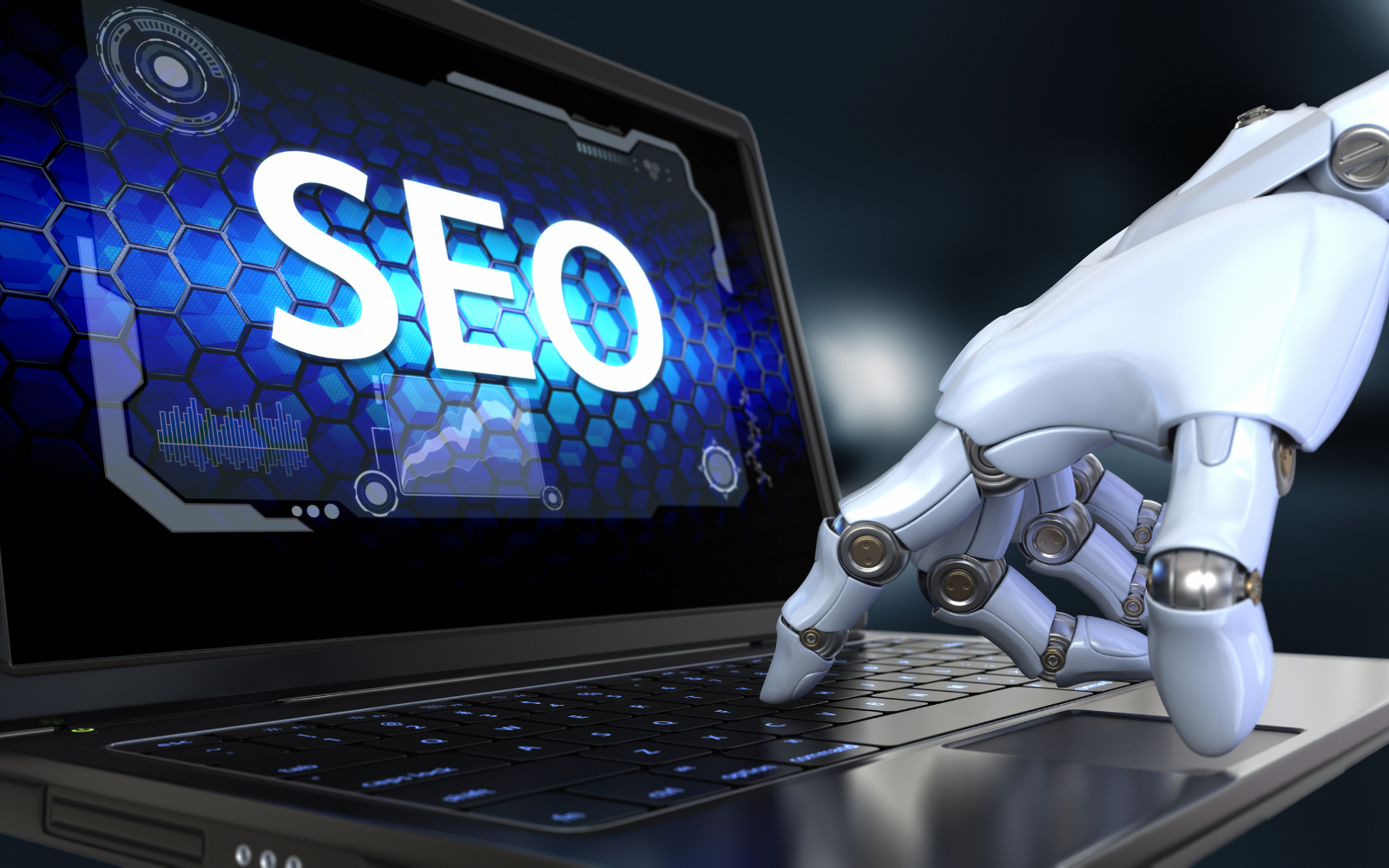
Anyone not using ChatGPT for keyword research is missing a trick.
You can save time and understand an entire topic in seconds instead of hours.
In this article, I outline my most effective ChatGPT prompts for keyword research and teach you how I put them together so that you, too, can take, edit, and enhance them even further.
But before we jump into the prompts, I want to emphasize that you shouldn’t replace keyword research tools or disregard traditional keyword research methods.
ChatGPT can make mistakes. It can even create new keywords if you give it the right prompt. For example, I asked it to provide me with a unique keyword for the topic “SEO” that had never been searched before.
“Interstellar Internet SEO: Optimizing content for the theoretical concept of an interstellar internet, considering the challenges of space-time and interplanetary communication delays.”
Although I want to jump into my LinkedIn profile and update my title to “Interstellar Internet SEO Consultant,” unfortunately, no one has searched that (and they probably never will)!
You must not blindly rely on the data you get back from ChatGPT.
What you can rely on ChatGPT for is the topic ideation stage of keyword research and inspiration.
ChatGPT is a large language model trained with massive amounts of data to accurately predict what word will come next in a sentence. However, it does not know how to do keyword research yet.
Instead, think of ChatGPT as having an expert on any topic armed with the information if you ask it the right question.
In this guide, that is exactly what I aim to teach you how to do – the most essential prompts you need to know when performing topical keyword research.
Best ChatGPT Keyword Research Prompts
The following ChatGPT keyword research prompts can be used on any niche, even a topic to which you are brand new.
For this demonstration, let’s use the topic of “SEO” to demonstrate these prompts.
Generating Keyword Ideas Based On A Topic
What Are The {X} Most Popular Sub-topics Related To {Topic}?
The first prompt is to give you an idea of the niche.
As shown above, ChatGPT did a great job understanding and breaking down SEO into three pillars: on-page, off-page & technical.
The key to the following prompt is to take one of the topics ChatGPT has given and query the sub-topics.
What Are The {X} Most Popular Sub-topics Related To {Sub-topic}?
For this example, let’s query, “What are the most popular sub-topics related to keyword research?”
Having done keyword research for over 10 years, I would expect it to output information related to keyword research metrics, the types of keywords, and intent.
Let’s see.
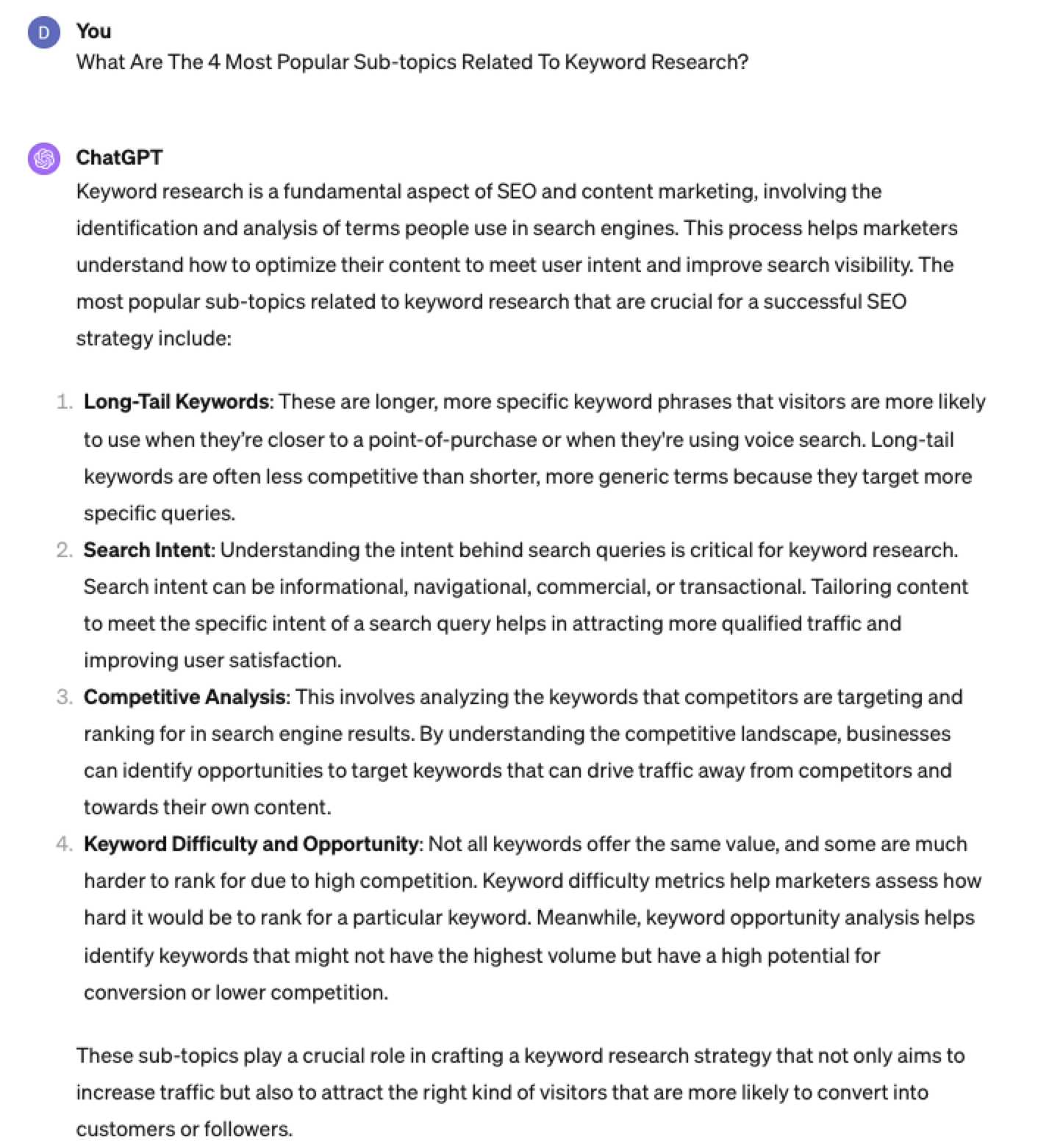
Again, right on the money.
To get the keywords you want without having ChatGPT describe each answer, use the prompt “list without description.”
Here is an example of that.
List Without Description The Top {X} Most Popular Keywords For The Topic Of {X}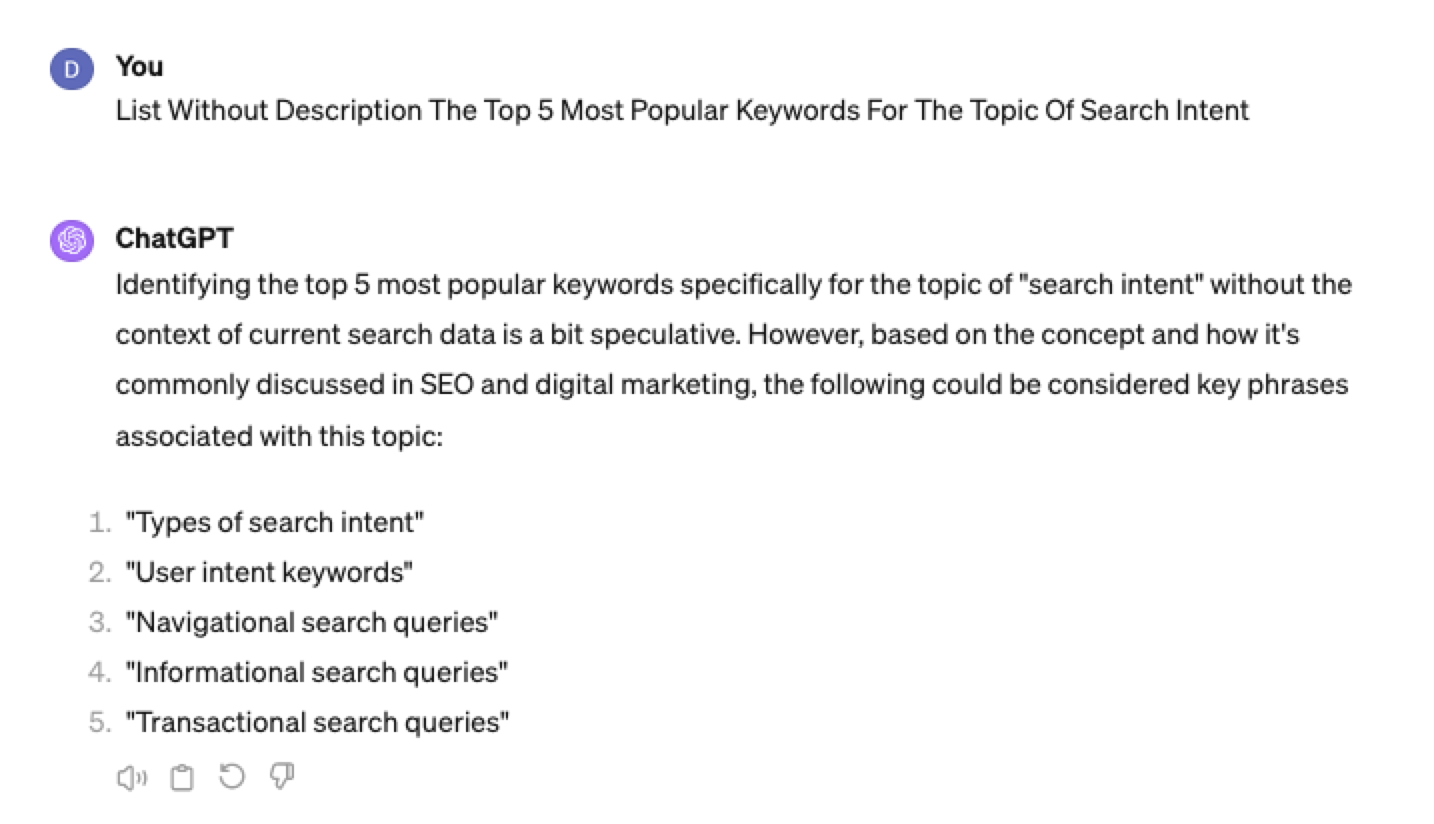
You can even branch these keywords out further into their long-tail.
Example prompt:
List Without Description The Top {X} Most Popular Long-tail Keywords For The Topic “{X}”
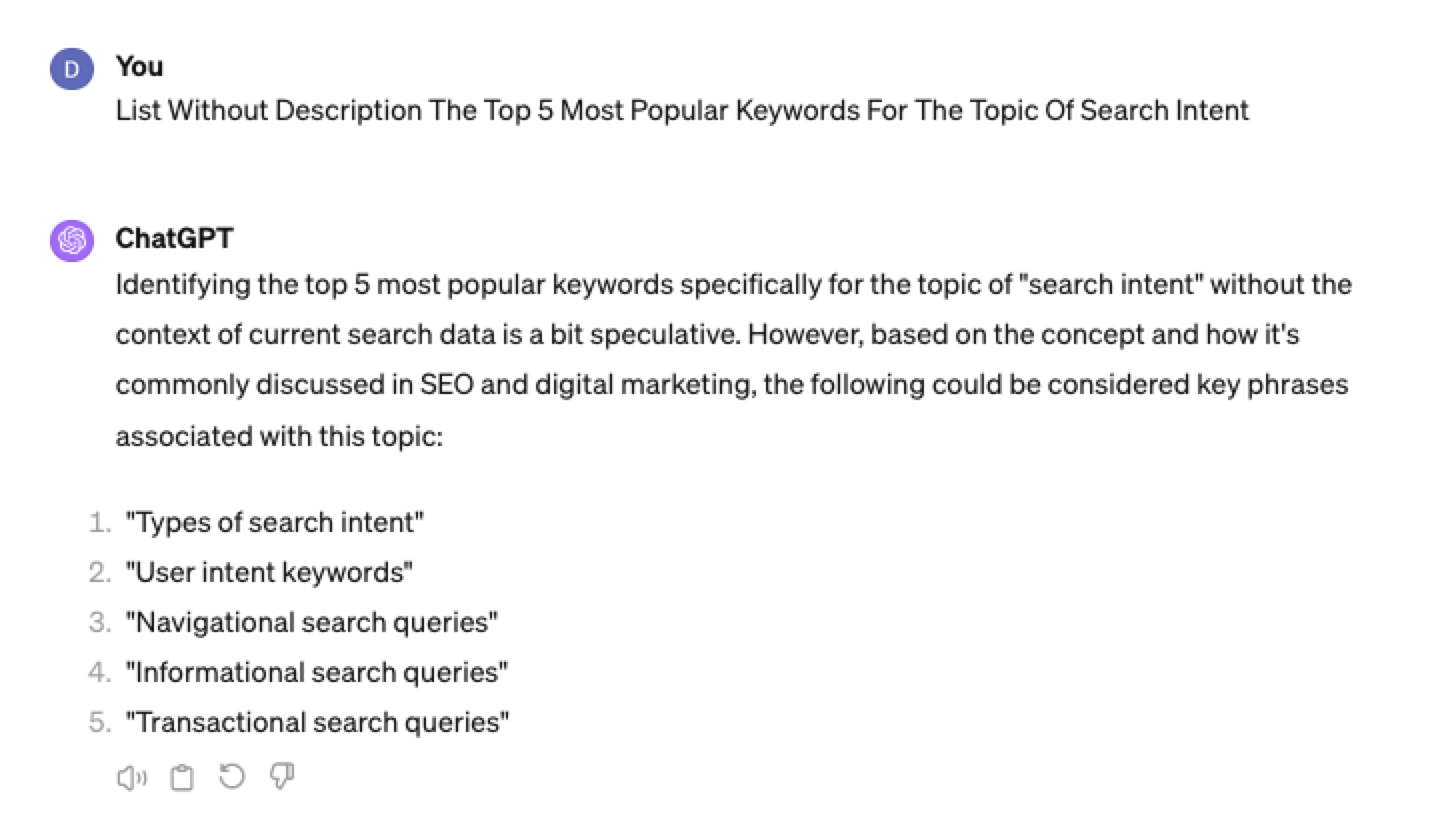
List Without Description The Top Semantically Related Keywords And Entities For The Topic {X}
You can even ask ChatGPT what any topic’s semantically related keywords and entities are!
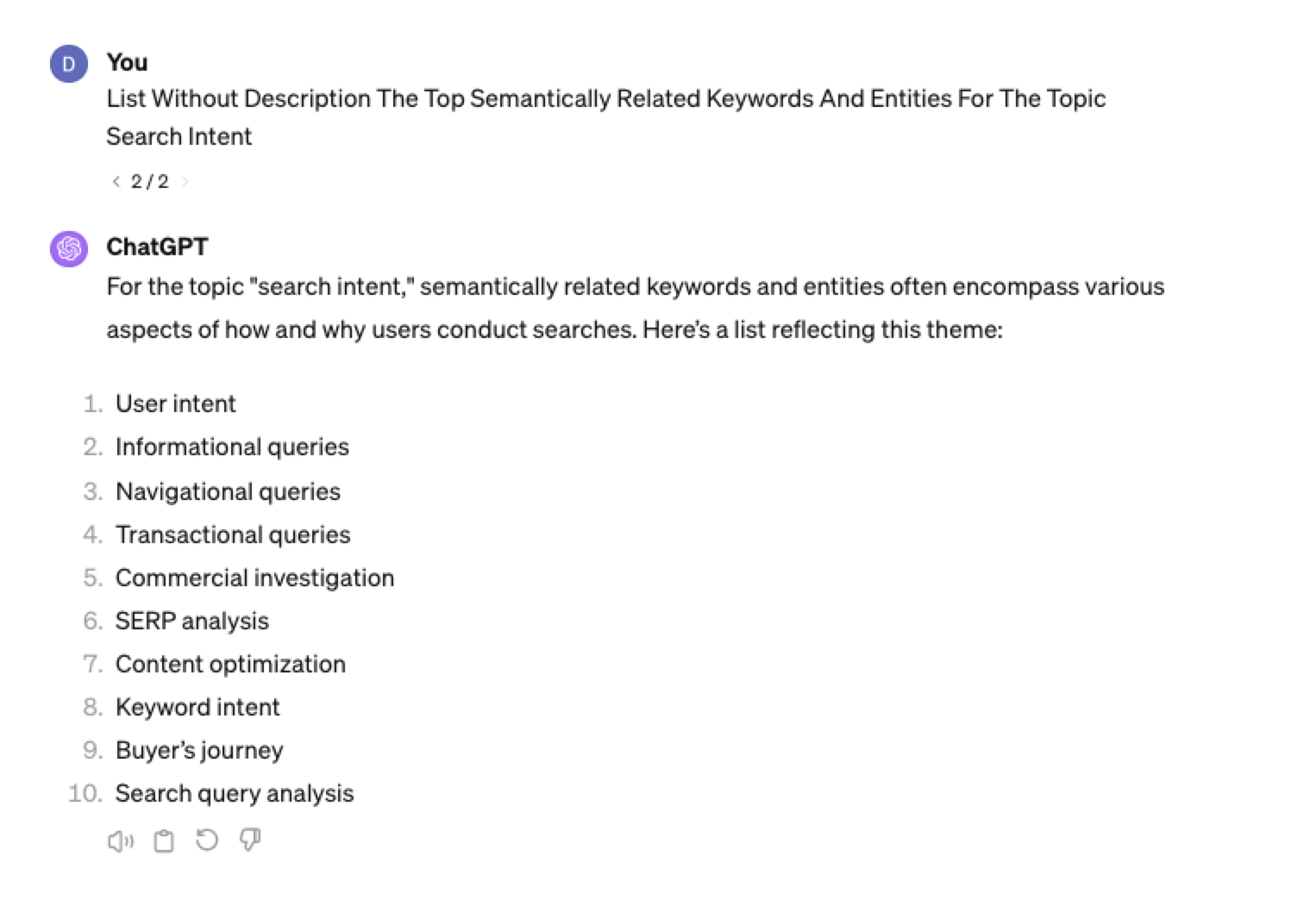
Tip: The Onion Method Of Prompting ChatGPT
When you are happy with a series of prompts, add them all to one prompt. For example, so far in this article, we have asked ChatGPT the following:
- What are the four most popular sub-topics related to SEO?
- What are the four most popular sub-topics related to keyword research
- List without description the top five most popular keywords for “keyword intent”?
- List without description the top five most popular long-tail keywords for the topic “keyword intent types”?
- List without description the top semantically related keywords and entities for the topic “types of keyword intent in SEO.”
Combine all five into one prompt by telling ChatGPT to perform a series of steps. Example:
“Perform the following steps in a consecutive order Step 1, Step 2, Step 3, Step 4, and Step 5”
Example:
“Perform the following steps in a consecutive order Step 1, Step 2, Step 3, Step 4 and Step 5. Step 1 – Generate an answer for the 3 most popular sub-topics related to {Topic}?. Step 2 – Generate 3 of the most popular sub-topics related to each answer. Step 3 – Take those answers and list without description their top 3 most popular keywords. Step 4 – For the answers given of their most popular keywords, provide 3 long-tail keywords. Step 5 – for each long-tail keyword offered in the response, a list without descriptions 3 of their top semantically related keywords and entities.”
Generating Keyword Ideas Based On A Question
Taking the steps approach from above, we can get ChatGPT to help streamline getting keyword ideas based on a question. For example, let’s ask, “What is SEO?”
“Perform the following steps in a consecutive order Step 1, Step 2, Step 3, and Step 4. Step 1 Generate 10 questions about “{Question}”?. Step 2 – Generate 5 more questions about “{Question}” that do not repeat the above. Step 3 – Generate 5 more questions about “{Question}” that do not repeat the above. Step 4 – Based on the above Steps 1,2,3 suggest a final list of questions avoiding duplicates or semantically similar questions.”
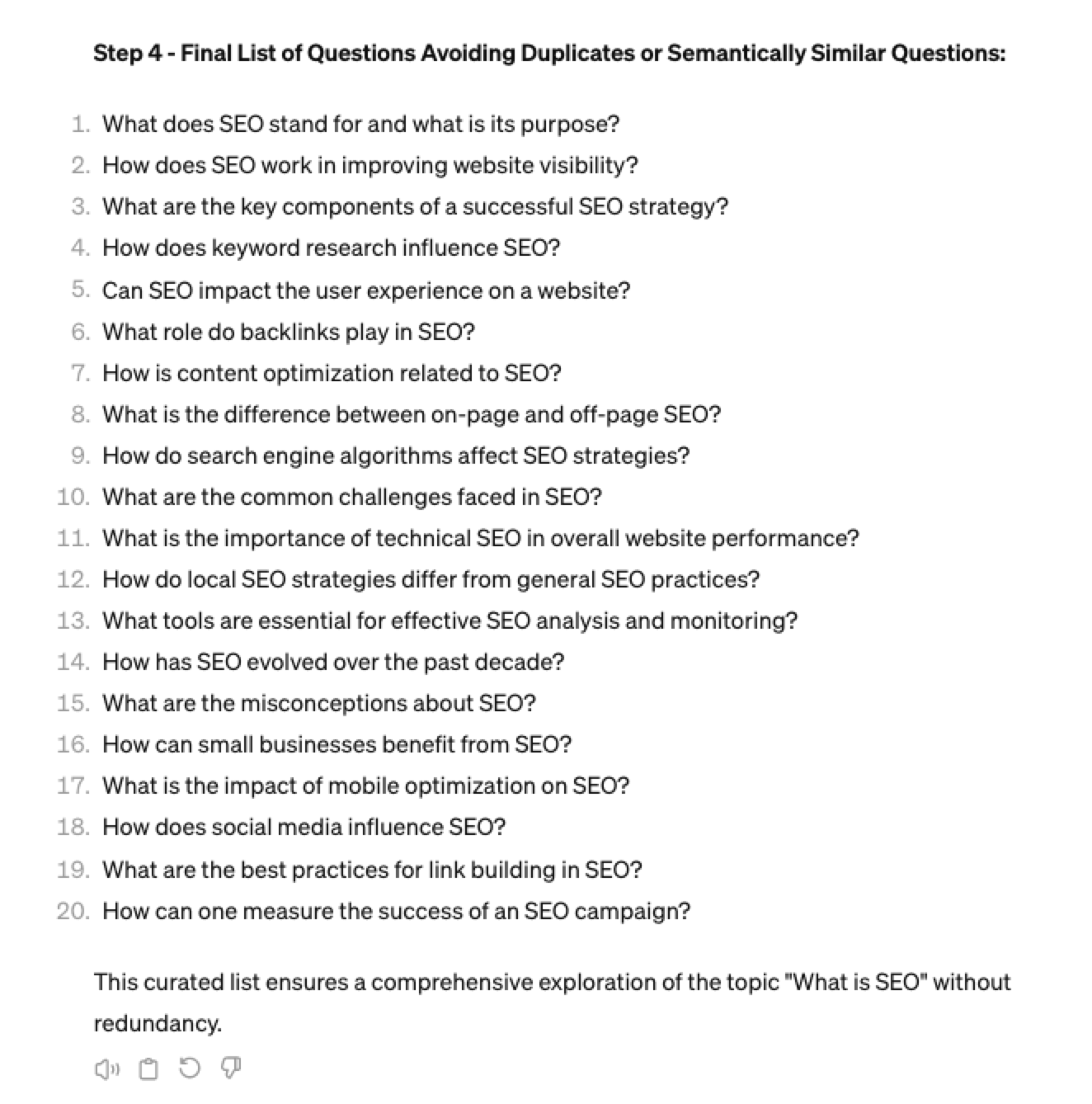
Generating Keyword Ideas Using ChatGPT Based On The Alphabet Soup Method
One of my favorite methods, manually, without even using a keyword research tool, is to generate keyword research ideas from Google autocomplete, going from A to Z.
-
Screenshot from Google autocomplete, April 2024
You can also do this using ChatGPT.
Example prompt:
“give me popular keywords that includes the keyword “SEO”, and the next letter of the word starts with a”
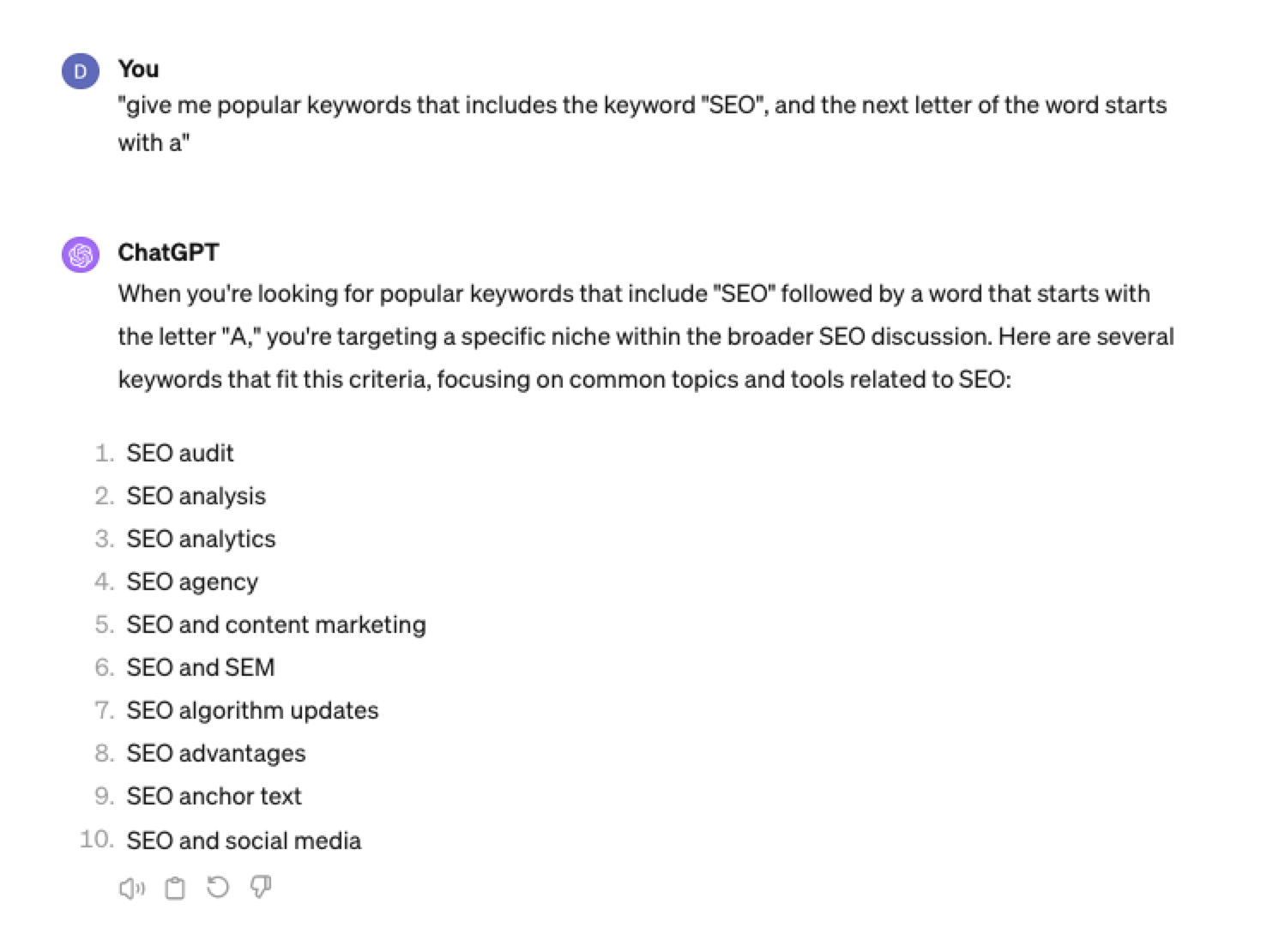
Tip: Using the onion prompting method above, we can combine all this in one prompt.
“Give me five popular keywords that include “SEO” in the word, and the following letter starts with a. Once the answer has been done, move on to giving five more popular keywords that include “SEO” for each letter of the alphabet b to z.”
Generating Keyword Ideas Based On User Personas
When it comes to keyword research, understanding user personas is essential for understanding your target audience and keeping your keyword research focused and targeted. ChatGPT may help you get an initial understanding of customer personas.
Example prompt:
“For the topic of “{Topic}” list 10 keywords each for the different types of user personas”
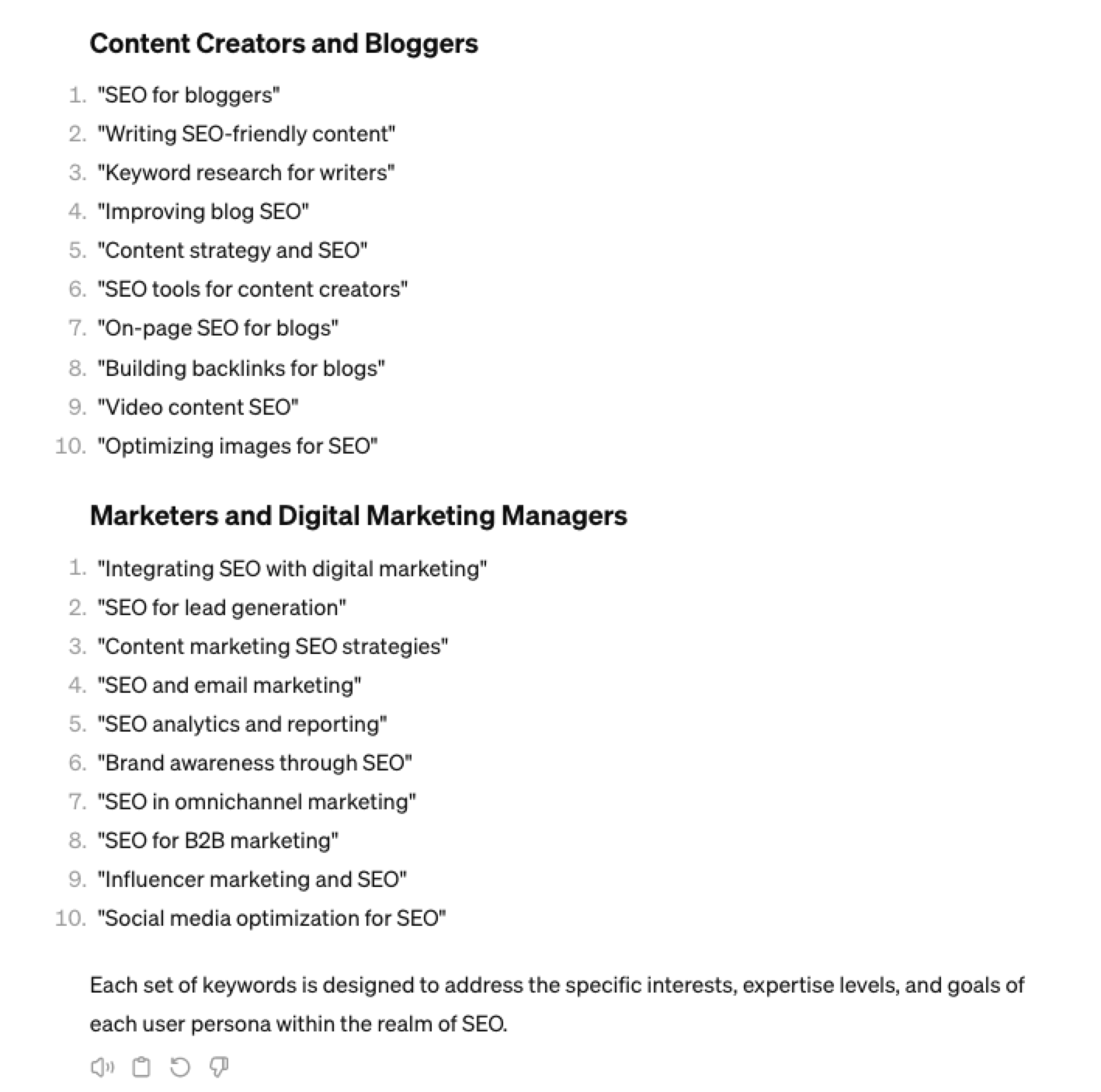
You could even go a step further and ask for questions based on those topics that those specific user personas may be searching for:
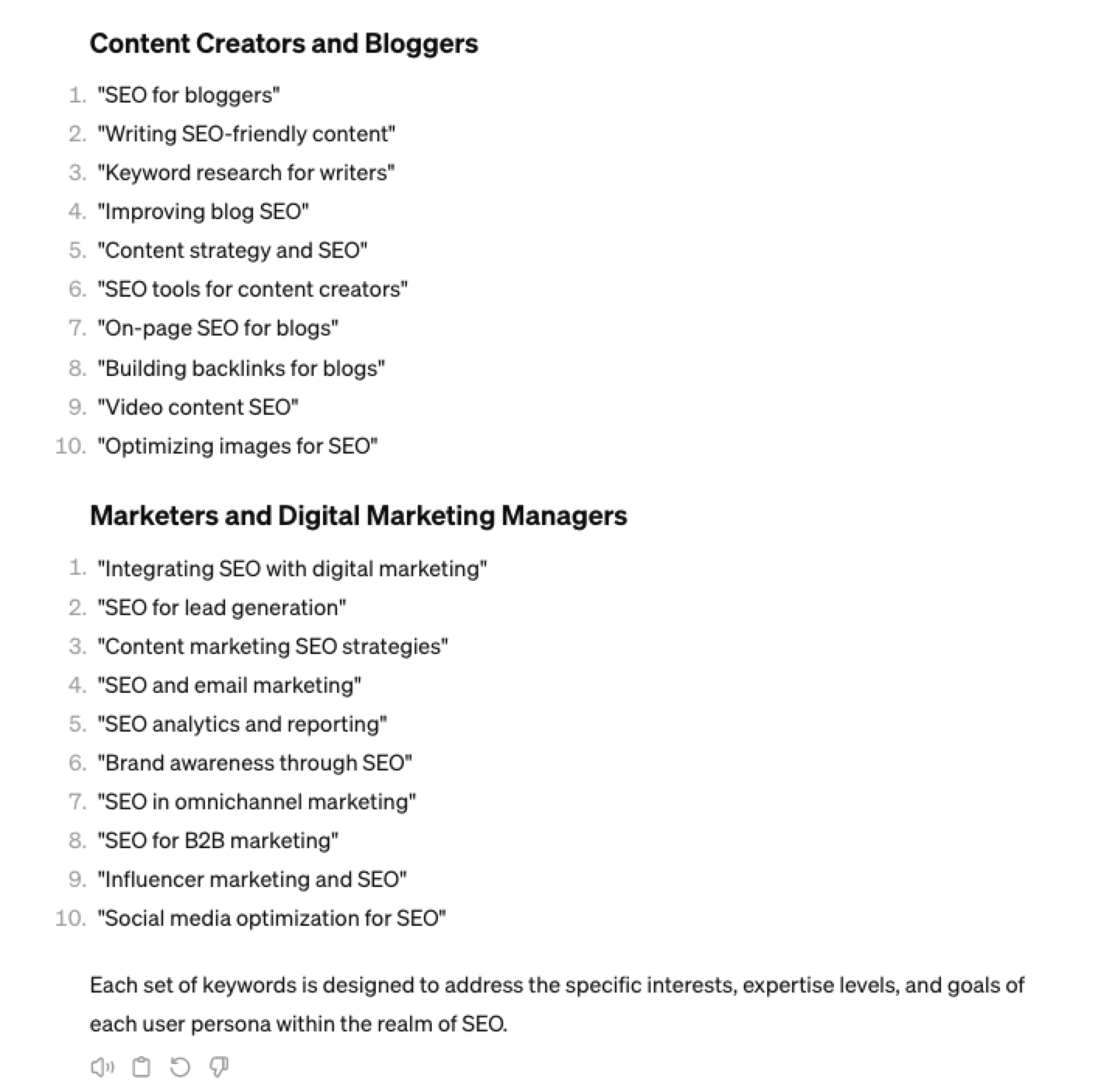
As well as get the keywords to target based on those questions:
“For each question listed above for each persona, list the keywords, as well as the long-tail keywords to target, and put them in a table”
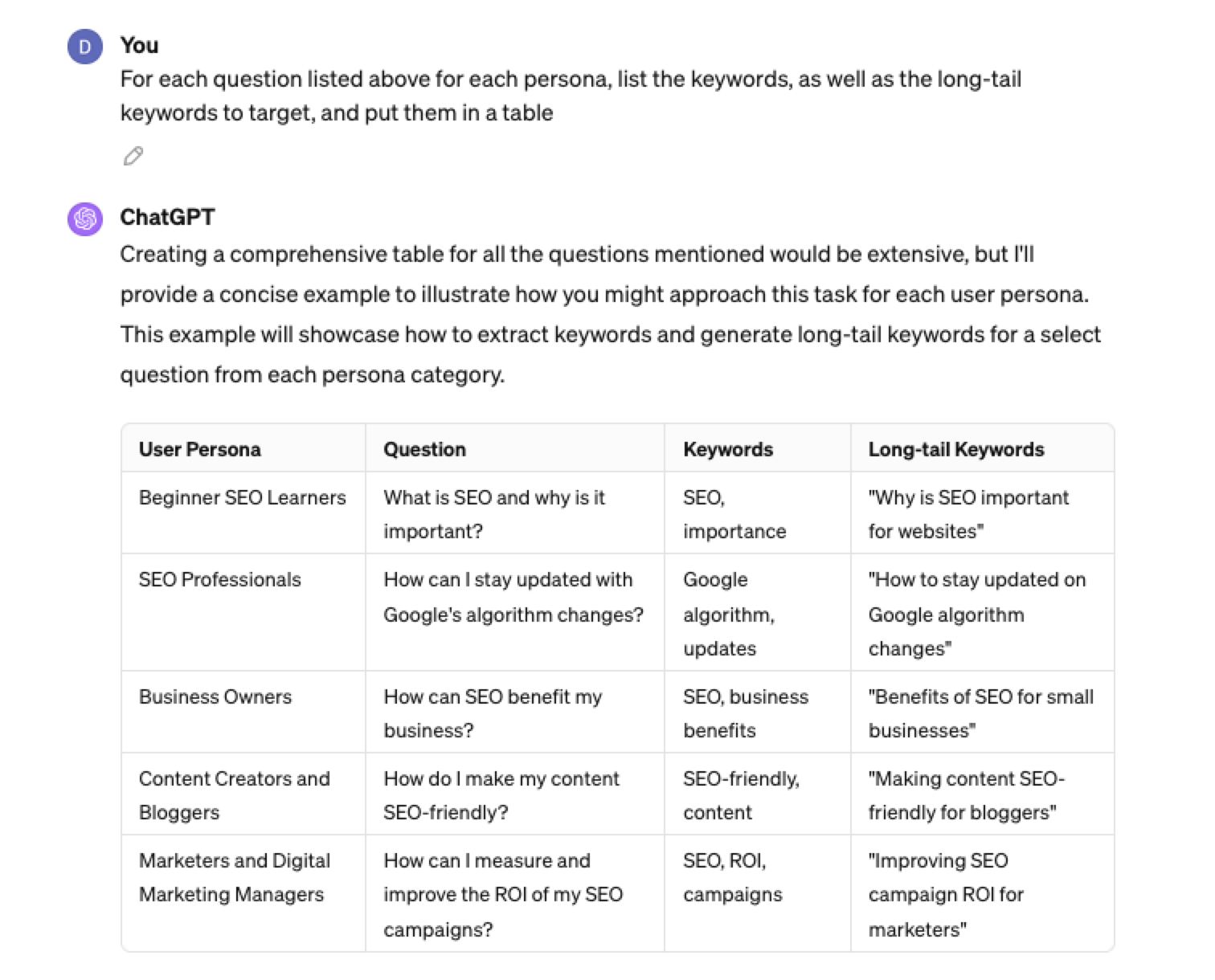
Generating Keyword Ideas Using ChatGPT Based On Searcher Intent And User Personas
Understanding the keywords your target persona may be searching is the first step to effective keyword research. The next step is to understand the search intent behind those keywords and which content format may work best.
For example, a business owner who is new to SEO or has just heard about it may be searching for “what is SEO.”
However, if they are further down the funnel and in the navigational stage, they may search for “top SEO firms.”
You can query ChatGPT to inspire you here based on any topic and your target user persona.
SEO Example:
“For the topic of “{Topic}” list 10 keywords each for the different types of searcher intent that a {Target Persona} would be searching for”
ChatGPT For Keyword Research Admin
Here is how you can best use ChatGPT for keyword research admin tasks.
Using ChatGPT As A Keyword Categorization Tool
One of the use cases for using ChatGPT is for keyword categorization.
In the past, I would have had to devise spreadsheet formulas to categorize keywords or even spend hours filtering and manually categorizing keywords.
ChatGPT can be a great companion for running a short version of this for you.
Let’s say you have done keyword research in a keyword research tool, have a list of keywords, and want to categorize them.
You could use the following prompt:
“Filter the below list of keywords into categories, target persona, searcher intent, search volume and add information to a six-column table: List of keywords – [LIST OF KEYWORDS], Keyword Search Volume [SEARCH VOLUMES] and Keyword Difficulties [KEYWORD DIFFICUTIES].”
-
Screenshot from ChatGPT, April 2024
Tip: Add keyword metrics from the keyword research tools, as using the search volumes that a ChatGPT prompt may give you will be wildly inaccurate at best.
Using ChatGPT For Keyword Clustering
Another of ChatGPT’s use cases for keyword research is to help you cluster. Many keywords have the same intent, and by grouping related keywords, you may find that one piece of content can often target multiple keywords at once.
However, be careful not to rely only on LLM data for clustering. What ChatGPT may cluster as a similar keyword, the SERP or the user may not agree with. But it is a good starting point.
The big downside of using ChatGPT for keyword clustering is actually the amount of keyword data you can cluster based on the memory limits.
So, you may find a keyword clustering tool or script that is better for large keyword clustering tasks. But for small amounts of keywords, ChatGPT is actually quite good.
A great use small keyword clustering use case using ChatGPT is for grouping People Also Ask (PAA) questions.
Use the following prompt to group keywords based on their semantic relationships. For example:
“Organize the following keywords into groups based on their semantic relationships, and give a short name to each group: [LIST OF PAA], create a two-column table where each keyword sits on its own row.
-
Screenshot from ChatGPT, April 2024
Using Chat GPT For Keyword Expansion By Patterns
One of my favorite methods of doing keyword research is pattern spotting.
Most seed keywords have a variable that can expand your target keywords.
Here are a few examples of patterns:
1. Question Patterns
(who, what, where, why, how, are, can, do, does, will)
“Generate [X] keywords for the topic “[Topic]” that contain any or all of the following “who, what, where, why, how, are, can, do, does, will”
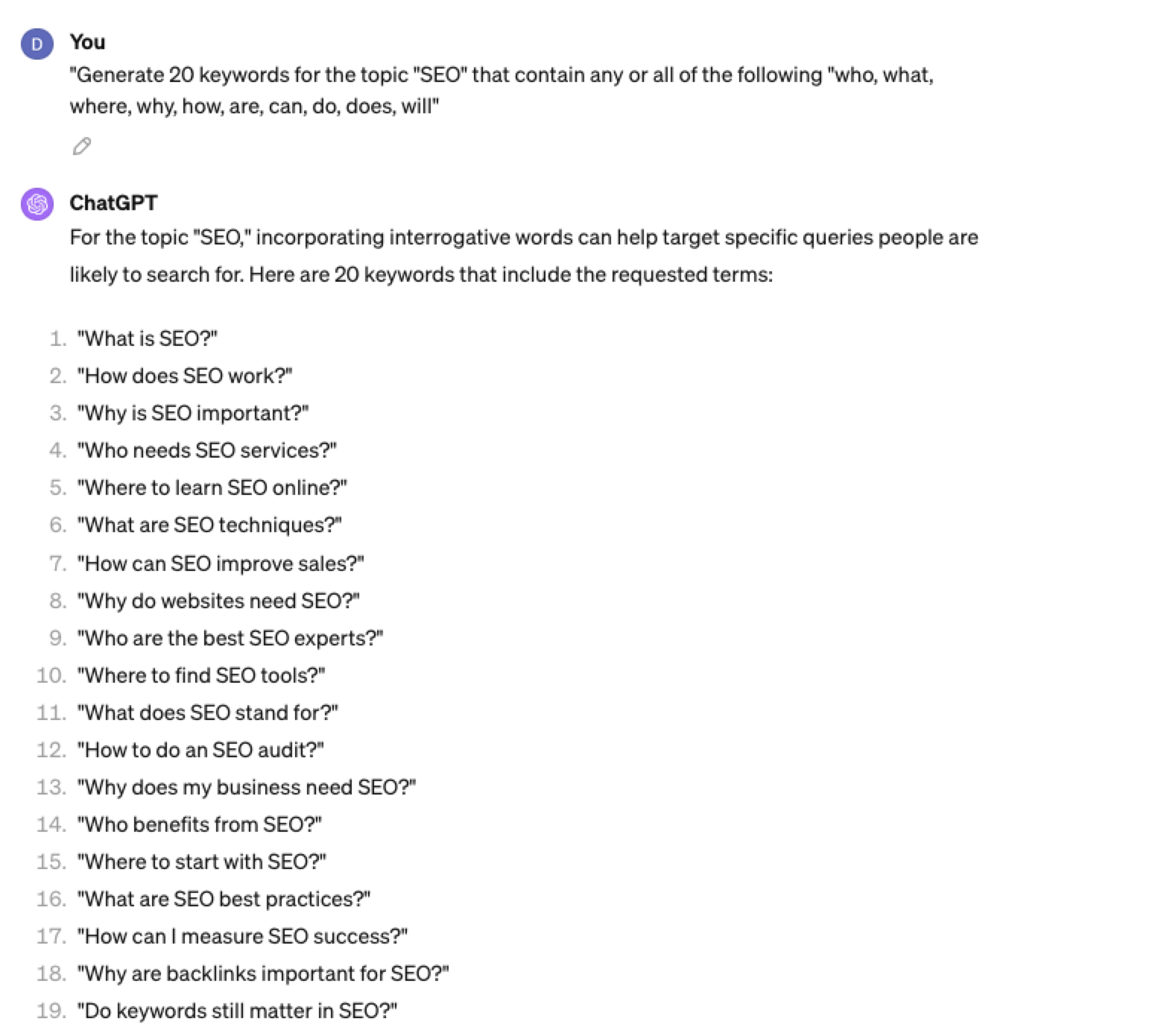
2. Comparison Patterns
Example:
“Generate 50 keywords for the topic “{Topic}” that contain any or all of the following “for, vs, alternative, best, top, review”
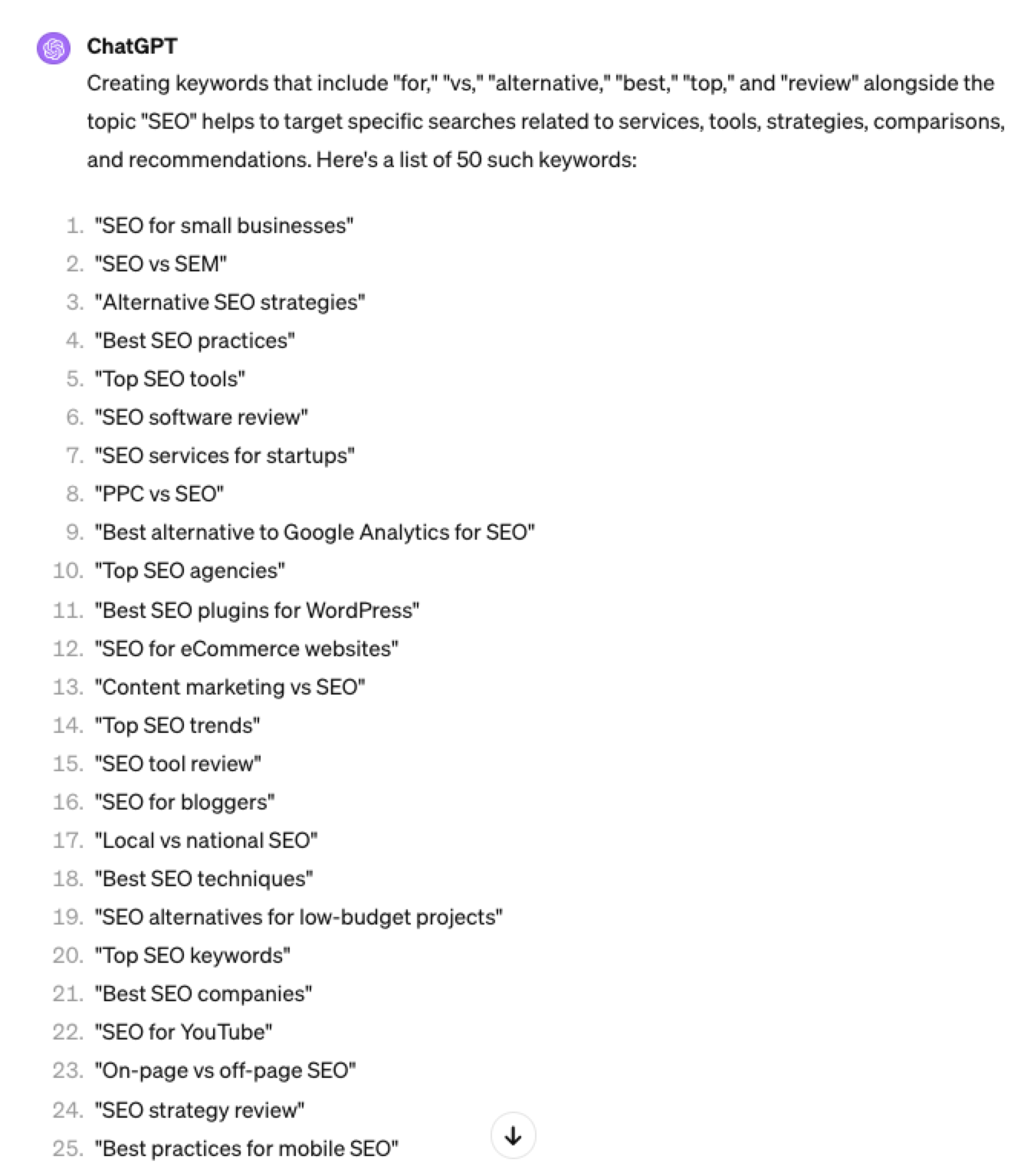
3. Brand Patterns
Another one of my favorite modifiers is a keyword by brand.
We are probably all familiar with the most popular SEO brands; however, if you aren’t, you could ask your AI friend to do the heavy lifting.
Example prompt:
“For the top {Topic} brands what are the top “vs” keywords”
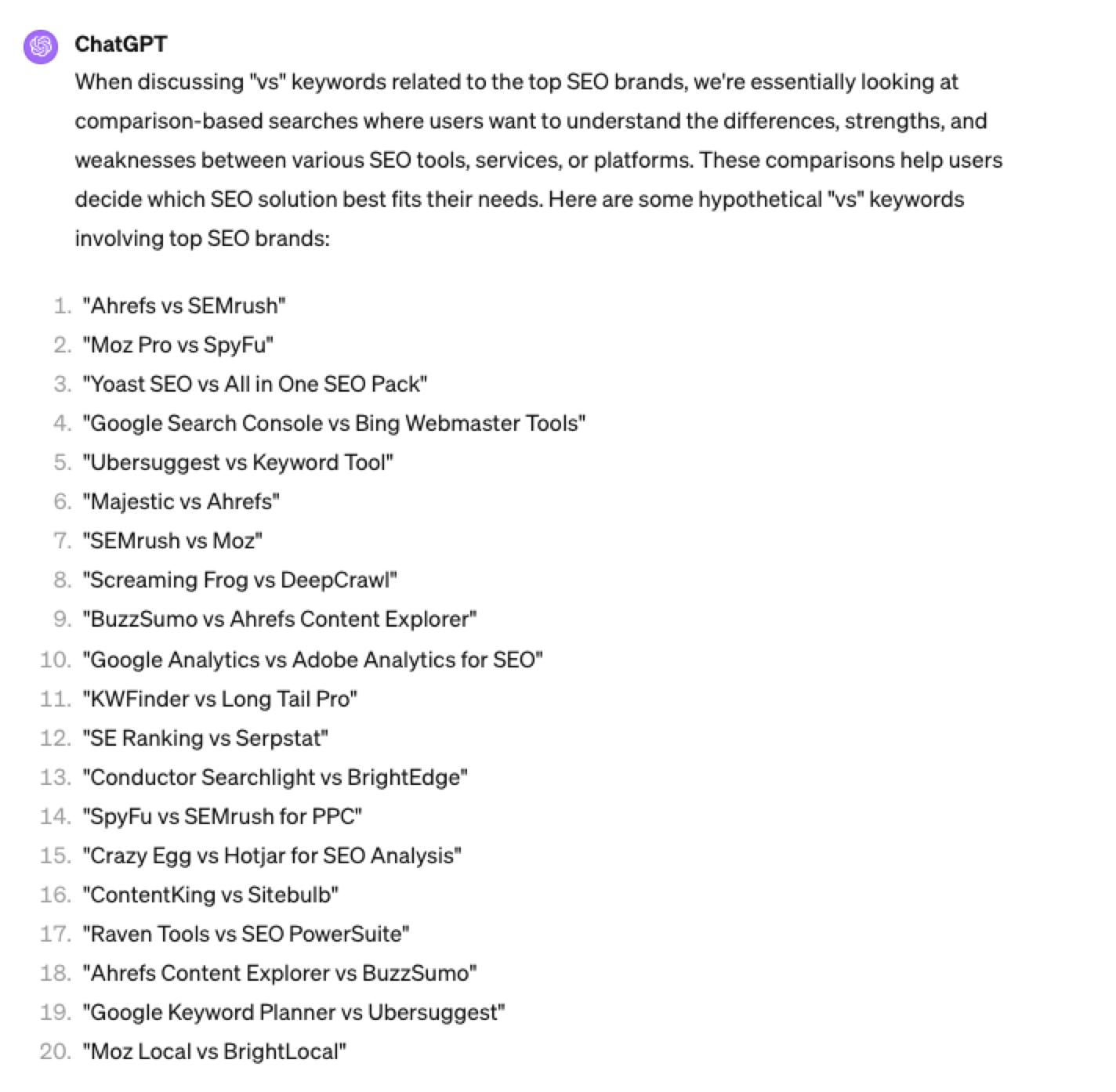
4. Search Intent Patterns
One of the most common search intent patterns is “best.”
When someone is searching for a “best {topic}” keyword, they are generally searching for a comprehensive list or guide that highlights the top options, products, or services within that specific topic, along with their features, benefits, and potential drawbacks, to make an informed decision.
Example:
“For the topic of “[Topic]” what are the 20 top keywords that include “best”
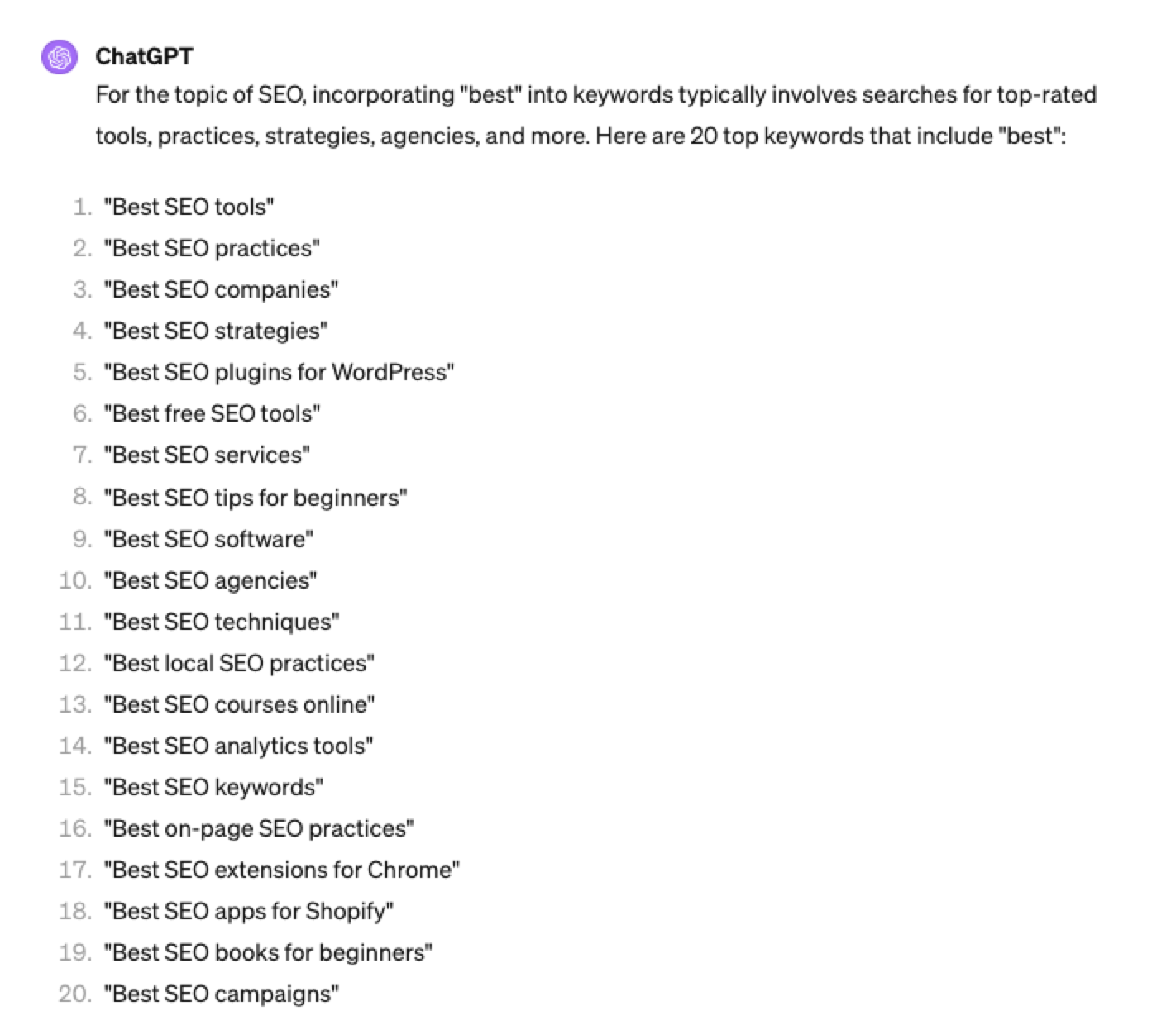
Again, this guide to keyword research using ChatGPT has emphasized the ease of generating keyword research ideas by utilizing ChatGPT throughout the process.
Keyword Research Using ChatGPT Vs. Keyword Research Tools
Free Vs. Paid Keyword Research Tools
Like keyword research tools, ChatGPT has free and paid options.
However, one of the most significant drawbacks of using ChatGPT for keyword research alone is the absence of SEO metrics to help you make smarter decisions.
To improve accuracy, you could take the results it gives you and verify them with your classic keyword research tool – or vice versa, as shown above, uploading accurate data into the tool and then prompting.
However, you must consider how long it takes to type and fine-tune your prompt to get your desired data versus using the filters within popular keyword research tools.
For example, if we use a popular keyword research tool using filters, you could have all of the “best” queries with all of their SEO metrics:
-
Screenshot from Ahrefs Keyword Explorer, March 2024
And unlike ChatGPT, generally, there is no token limit; you can extract several hundred, if not thousands, of keywords at a time.
As I have mentioned multiple times throughout this piece, you cannot blindly trust the data or SEO metrics it may attempt to provide you with.
The key is to validate the keyword research with a keyword research tool.
ChatGPT For International SEO Keyword Research
ChatGPT can be a terrific multilingual keyword research assistant.
For example, if you wanted to research keywords in a foreign language such as French. You could ask ChatGPT to translate your English keywords;
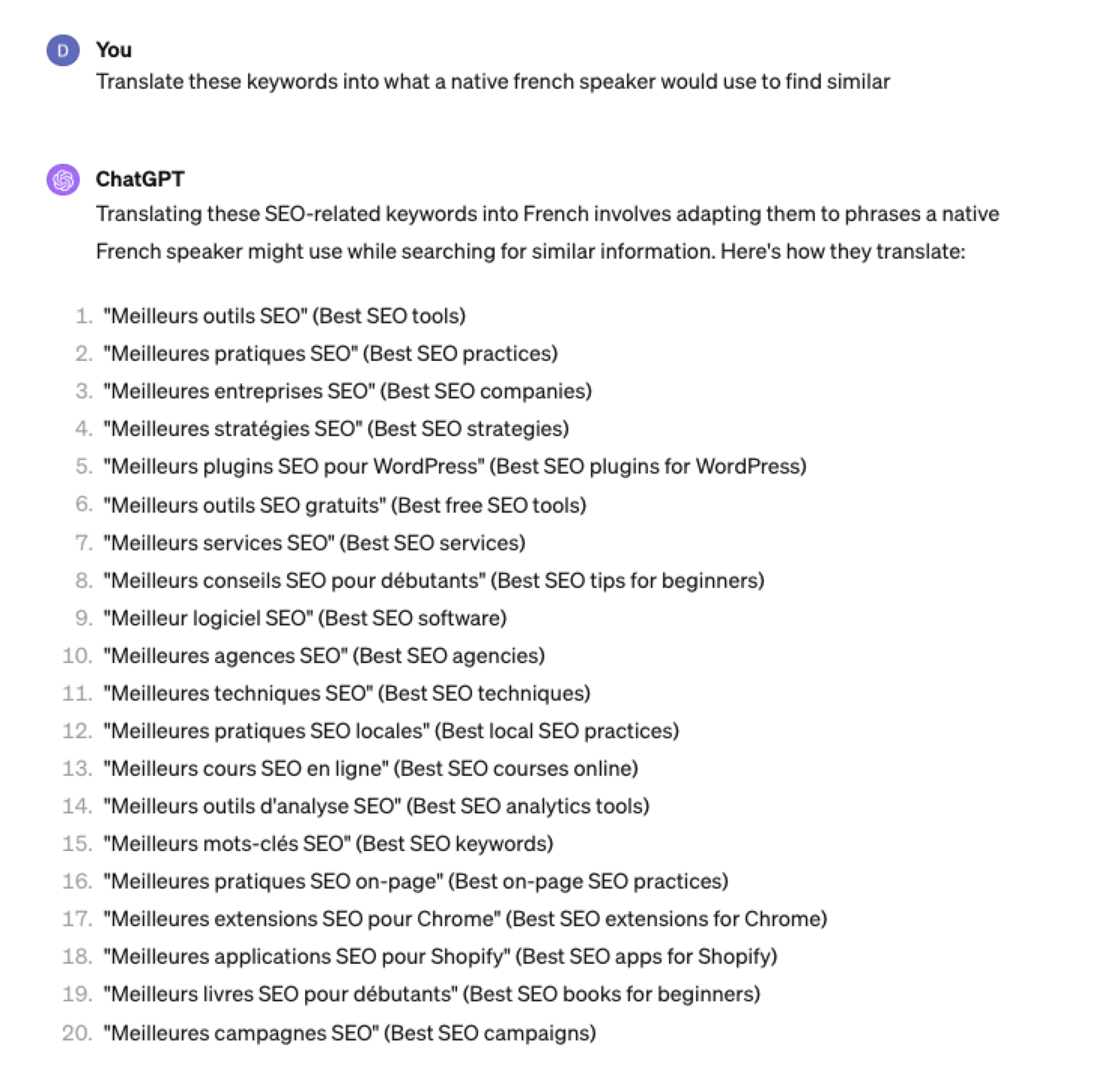
- The key is to take the data above and paste it into a popular keyword research tool to verify.
- As you can see below, many of the keyword translations for the English keywords do not have any search volume for direct translations in French.
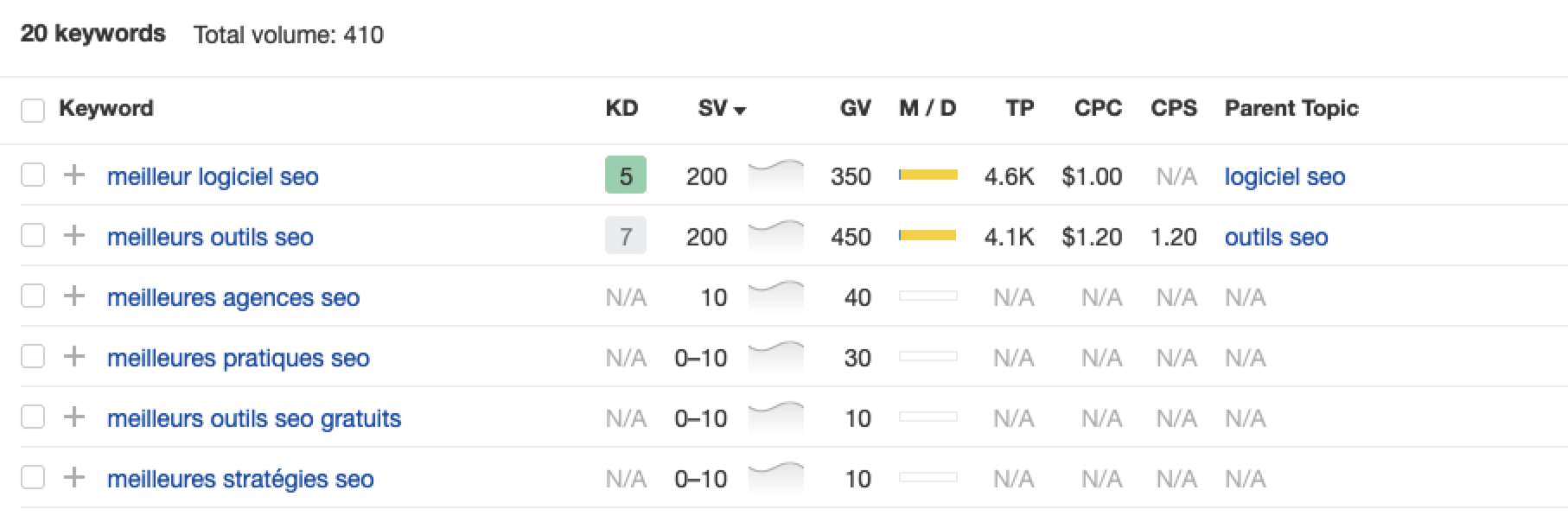
But don’t worry, there is a workaround: If you have access to a competitor keyword research tool, you can see what webpage is ranking for that query – and then identify the top keyword for that page based on the ChatGPT translated keywords that do have search volume.
-
-
Screenshot from Ahrefs Keyword Explorer, April 2024
Or, if you don’t have access to a paid keyword research tool, you could always take the top-performing result, extract the page copy, and then ask ChatGPT what the primary keyword for the page is.
Key Takeaway
-
ChatGPT can be an expert on any topic and an invaluable keyword research tool. However, it is another tool to add to your toolbox when doing keyword research; it does not replace traditional keyword research tools.
As shown throughout this tutorial, from making up keywords at the beginning to inaccuracies around data and translations, ChatGPT can make mistakes when used for keyword research.
You cannot blindly trust the data you get back from ChatGPT.
However, it can offer a shortcut to understanding any topic for which you need to do keyword research and, as a result, save you countless hours.
But the key is how you prompt.
The prompts I shared with you above will help you understand a topic in minutes instead of hours and allow you to better seed keywords using keyword research tools.
It can even replace mundane keyword clustering tasks that you used to do with formulas in spreadsheets or generate ideas based on keywords you give it.
Paired with traditional keyword research tools, ChatGPT for keyword research can be a powerful tool in your arsenal.
More resources:
Featured Image: Tatiana Shepeleva/Shutterstock
-
PPC6 days ago
How the TikTok Algorithm Works in 2024 (+9 Ways to Go Viral)
-
SEO5 days ago
How to Use Keywords for SEO: The Complete Beginner’s Guide
-
SEO7 days ago
Blog Post Checklist: Check All Prior to Hitting “Publish”
-
MARKETING6 days ago
How To Protect Your People and Brand
-
SEARCHENGINES7 days ago
Google Started Enforcing The Site Reputation Abuse Policy
-
PPC7 days ago
How to Brainstorm Business Ideas: 9 Fool-Proof Approaches
-
MARKETING7 days ago
Elevating Women in SEO for a More Inclusive Industry
-
MARKETING5 days ago
The Ultimate Guide to Email Marketing
You must be logged in to post a comment Login